A prediction model for asthma exacerbations after stopping asthma biologics.
Annals of allergy, asthma & immunology : official publication of the American College of Allergy, Asthma, & Immunology(2022)
摘要
BACKGROUND:Little is known regarding the prediction of the risks of asthma exacerbation after stopping asthma biologics.
OBJECTIVE:To develop and validate a predictive model for the risk of asthma exacerbations after stopping asthma biologics using machine learning models.
METHODS:We identified 3057 people with asthma who stopped asthma biologics in the OptumLabs Database Warehouse and considered a wide range of demographic and clinical risk factors to predict subsequent outcomes. The primary outcome used to assess success after stopping was having no exacerbations in the 6 months after stopping the biologic. Elastic-net logistic regression (GLMnet), random forest, and gradient boosting machine models were used with 10-fold cross-validation within a development (80%) cohort and validation cohort (20%).
RESULTS:The mean age of the total cohort was 47.1 (SD, 17.1) years, 1859 (60.8%) were women, 2261 (74.0%) were White, and 1475 (48.3%) were in the Southern region of the United States. The elastic-net logistic regression model yielded an area under the curve (AUC) of 0.75 (95% confidence interval [CI], 0.71-0.78) in the development and an AUC of 0.72 in the validation cohort. The random forest model yielded an AUC of 0.75 (95% CI, 0.68-0.79) in the development cohort and an AUC of 0.72 in the validation cohort. The gradient boosting machine model yielded an AUC of 0.76 (95% CI, 0.72-0.80) in the development cohort and an AUC of 0.74 in the validation cohort.
CONCLUSION:Outcomes after stopping asthma biologics can be predicted with moderate accuracy using machine learning methods.
更多查看译文
AI 理解论文
溯源树
样例
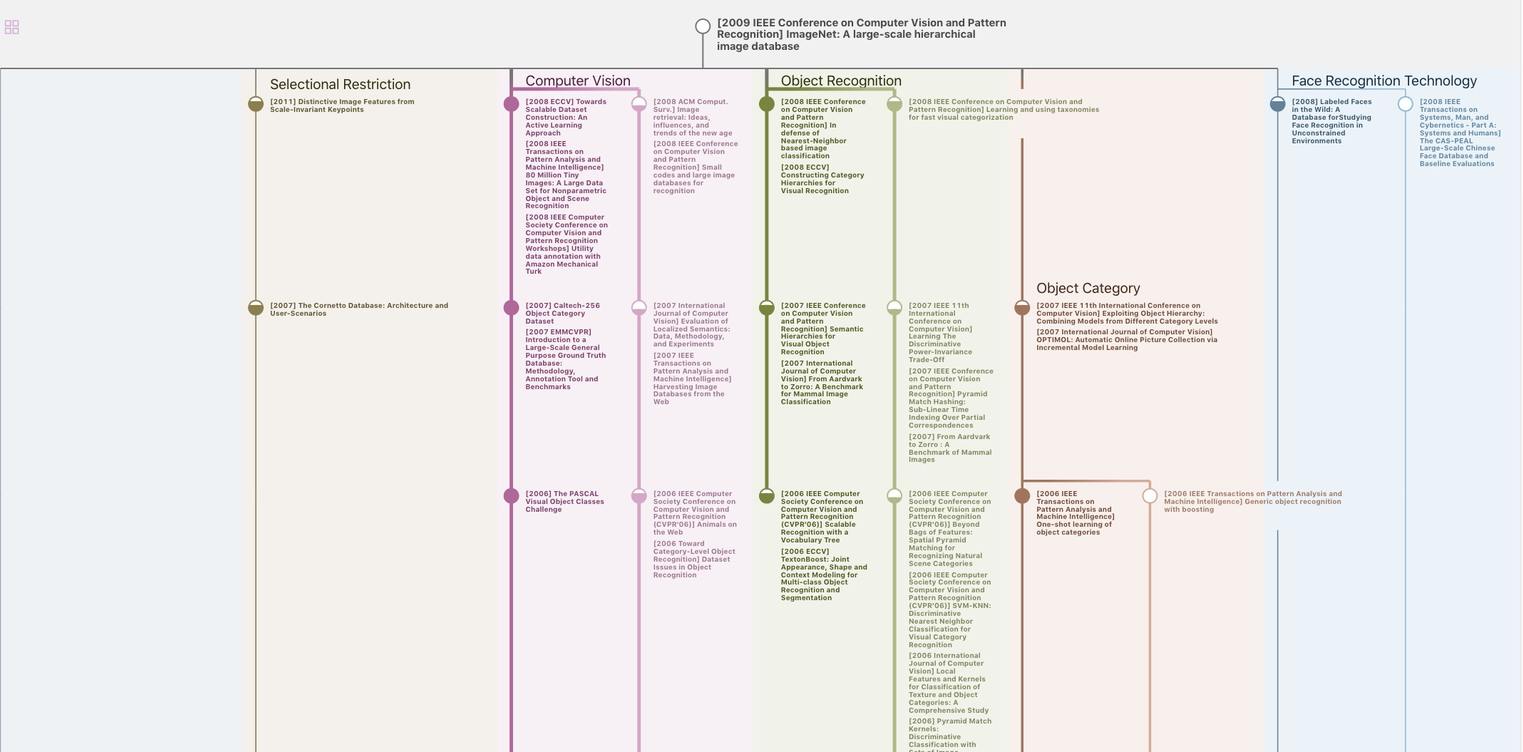
生成溯源树,研究论文发展脉络
Chat Paper
正在生成论文摘要