Causal network inference based on cross-validation predictability
biorxiv(2022)
摘要
Identifying causal relations or causal networks among molecules/genes, rather than just their correlations, is of great importance but challenging in biology and medical field, which is essential for unravelling molecular mechanisms of disease progression and developing effective therapies for disease treatment. However, there is still a lack of high-quality causal inference algorithms for any observed data in contrast to time series data. In this study, we developed a new causal concept for any observed data and its causal inference algorithm built on cross-validated predictability (CVP) can quantify the causal effects of interventions in the whole system. The causality was extensively validated by combining a large variety of statistical simulation experiments and available benchmark data (simulated data and various real data). Combining the predicted causal network and the real benchmark network, the CVP algorithm demonstrates high accuracy and strong robustness in comparison with the mainstream algorithms. In particular, the CVP algorithm is robust in identifying reliable driver genes and network biomarkers from the perspective of network biology, with the prediction results outperforming the mainstream conventional methods for predicting driver genes. CRISPR-Cas9 knockdown experiments in the liver cancer have validated that the functional driver genes identified by the CVP algorithm effectively inhibit the growth and colony formation of liver cancer cells. Combining knockdown and control experiments, we demonstrated the accuracy and biological significance of the causality predicted by CVP and identified the novel regulatory targets of functional driver genes SNRNP200 and RALGAPB in the liver cancer. These inferred causal networks explain regulatory patterns in different biological contexts in a clear sense and provide biological insights into molecular mechanisms of disease progression from a causality perspective.
### Competing Interest Statement
The authors have declared no competing interest.
更多查看译文
关键词
network,cross-validation
AI 理解论文
溯源树
样例
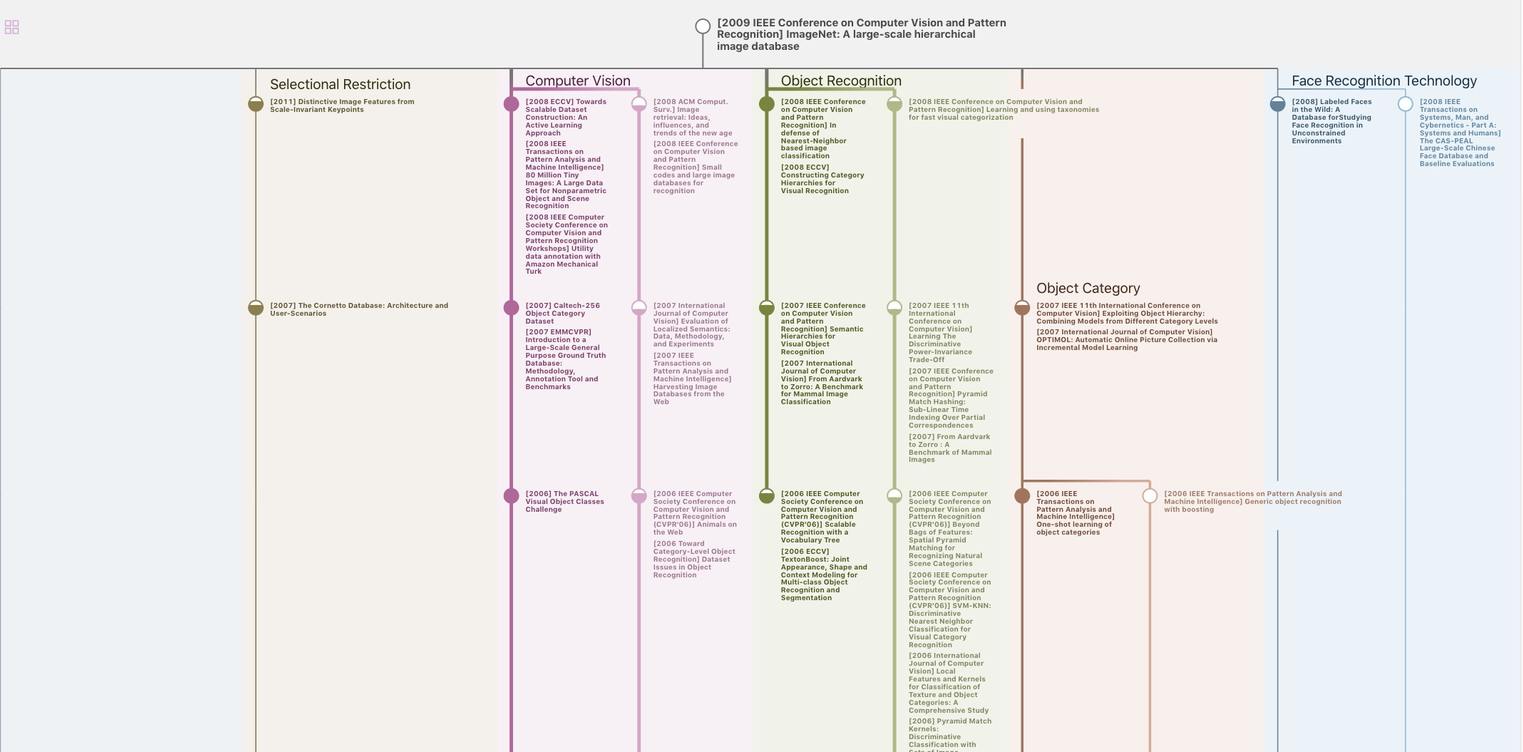
生成溯源树,研究论文发展脉络
Chat Paper
正在生成论文摘要