Graph neural networks learn emergent tissue properties from spatial molecular profiles
biorxiv(2022)
摘要
Tissue phenotypes such as metabolic states, inflammation, and tumor properties are functions of molecular states of cells that constitute the tissue. Recent spatial molecular profiling assays measure tissue architecture motifs in a molecular and often unbiased way and thus can explain some aspects of emergence of these phenotypes. Here, we characterize the ability of graph neural networks to model tissue-level emergent phenotypes based on spatial data by evaluating phenotype prediction across model complexities. First, we show that immune cell dispersion in colorectal tumors, which is known to be predictive of disease outcome, can be captured by graph neural networks. Second, we show that breast cancer tumor classes can be predicted from gene expression alone without spatial information and are thus too simplistic a phenotype to require a complex model of emergence. Third, we show that representation learning approaches for spatial graphs of molecular profiles are limited by overfitting in the prevalent regime of up to 100s of images per study. We address overfitting with within-graph self-supervision and illustrate its promise for tissue representation learning as a constraint for node representations.
### Competing Interest Statement
F.J.T. consults for Immunai Inc., Singularity Bio B.V., CytoReason Ltd, and Omniscope Ltd, and has ownership interest in Dermagnostix GmbH and Cellarity.
更多查看译文
关键词
graph neural networks,emergent tissue properties
AI 理解论文
溯源树
样例
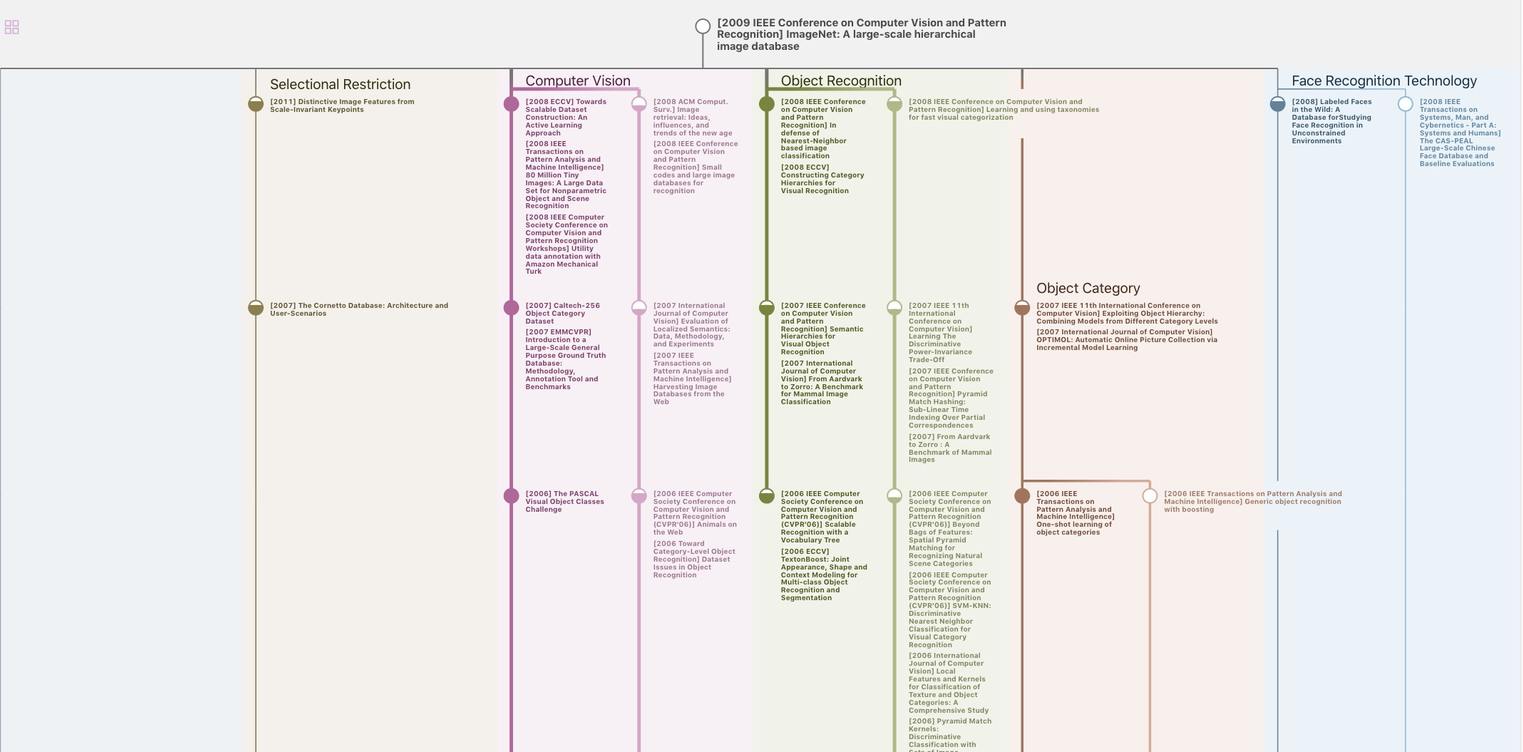
生成溯源树,研究论文发展脉络
Chat Paper
正在生成论文摘要