Multi-Energy Blended CBCT Spectral Imaging Using a Spectral Modulator with Flying Focal Spot (SMFFS)
arxiv(2022)
摘要
Conventional cone-beam CT (CBCT) can be easily compromised by scatter and
beam hardening artifacts, and the entanglement of scatter and spectral effects
introduces additional complexity. In this work, we present the first attempt to
develop a stationary spectral modulator with flying focal spot (SMFFS)
technology as a promising, low-cost approach to accurately solving the X-ray
scattering problem and physically enabling spectral imaging in a unified
framework. To deal with the intertwined scatter-spectral challenge, we propose
a novel scatter-decoupled material decomposition (SDMD) method for SMFFS based
on a hypothesis of scatter similarity. Monte Carlo simulations of a pure-water
cylinder phantom with different focal spot deflections show that focal spot
deflections within a range of 2 mm share quite similar scatter distributions
overall. Numerical simulations using a clinical abdominal CT dataset
demonstrate that SMFFS with SDMD method can achieve better material
decomposition and CT number accuracy with less artifacts. Physics experiments
on a tabletop CBCT system using a Gammex multi-energy CT phantom an
anthropomorphic chest phantom, are carried out to demonstrate the feasibility
of CBCT spectral imaging with SMFFS. For the chest phantom, the root mean
square error (RMSE) in selected regions of interest (ROIs) of virtual
monochromatic image (VMI) at 70 keV is 11.8 HU for SMFFS CB scan, and 14.5 and
437.6 HU for sequential 80/140 kVp (DKV) CB scan with and without scatter
correction, respectively. Also, the non-uniformity among selected regions is
14.1 HU for SMFFS CB scan, and 59.4 and 184.0 HU for the DKV CB scan with and
without a traditional scatter correction method, respectively. Our preliminary
results show that SMFFS can enable spectral imaging with simultaneous scatter
correction for CBCT and effectively improve its quantitative imaging
performance.
更多查看译文
AI 理解论文
溯源树
样例
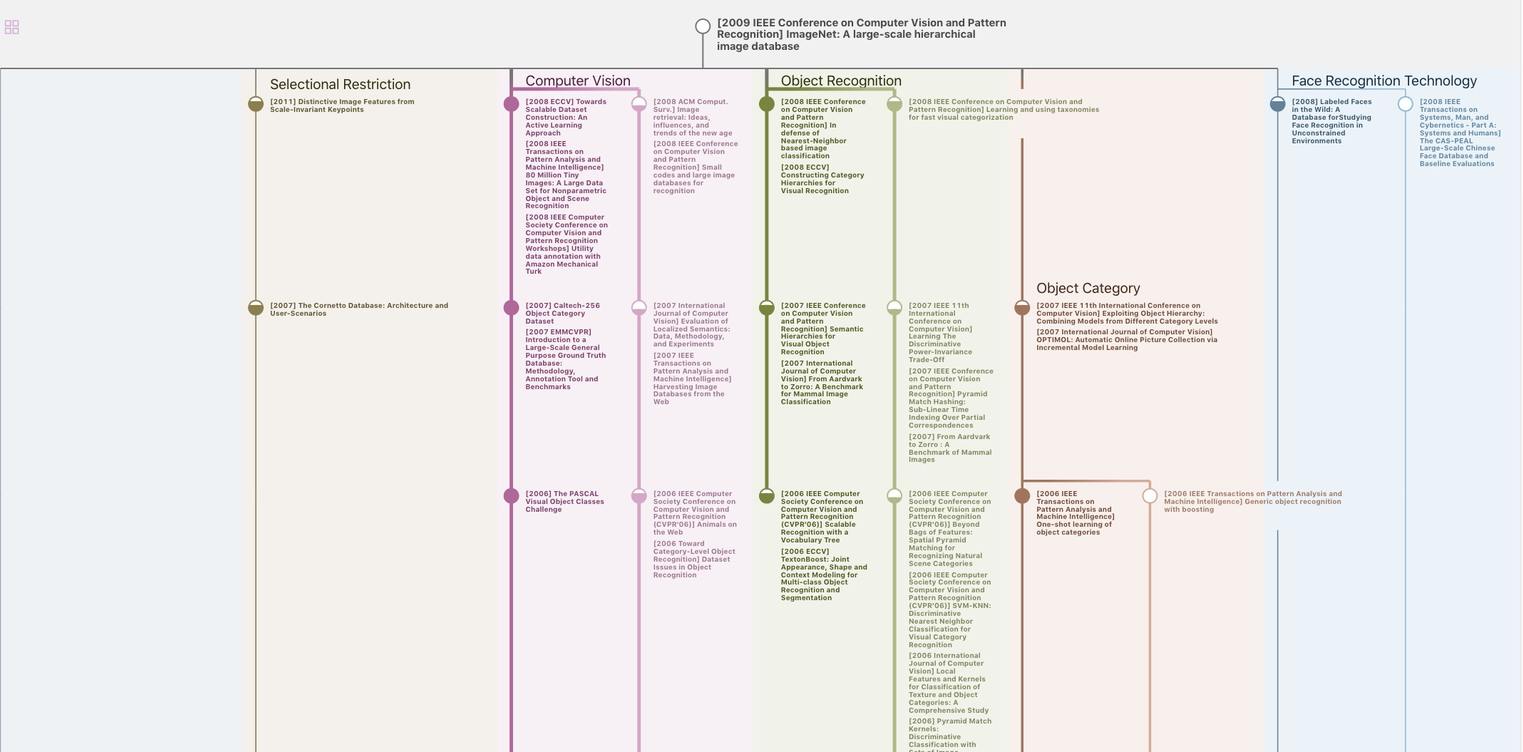
生成溯源树,研究论文发展脉络
Chat Paper
正在生成论文摘要