AutoGater: A Weakly Supervised Neural Network Model to Gate Cells in Flow Cytometric Analyses
biorxiv(2022)
摘要
Flow cytometry is a useful and efficient method for the rapid characterization of a cell population based on the optical and fluorescence properties of individual cells. Ideally, the cell population would consist of only healthy viable cells as dead cells can confound the analysis. Thus, separating out healthy cells from dying and dead cells, and any potential debris, is an important first step in analysis of flow cytometry data. While gating of debris can be conducted using measured optical properties, identifying dead and dying cells often requires utilizing fluorescent stains (e.g. Sytox, a nucleic acid stain that stains cells with compromised cell membranes) to identify cells that should be excluded from downstream analyses. These stains prolong the experimental preparation process and use a flow cytometers fluorescence channels that could otherwise be used to measure additional fluorescent markers within the cells (e.g. reporter proteins). Here we outline a stain-free method for identifying viable cells for downstream processing by gating cells that are dying or dead. AutoGater is a weakly supervised deep learning model that can separate healthy populations from unhealthy and dead populations using only light-scatter channels. In addition, AutoGater harmonizes different measurements of dead cells such as Sytox and CFUs.
### Competing Interest Statement
The authors have declared no competing interest.
更多查看译文
关键词
autogater cells,flow
AI 理解论文
溯源树
样例
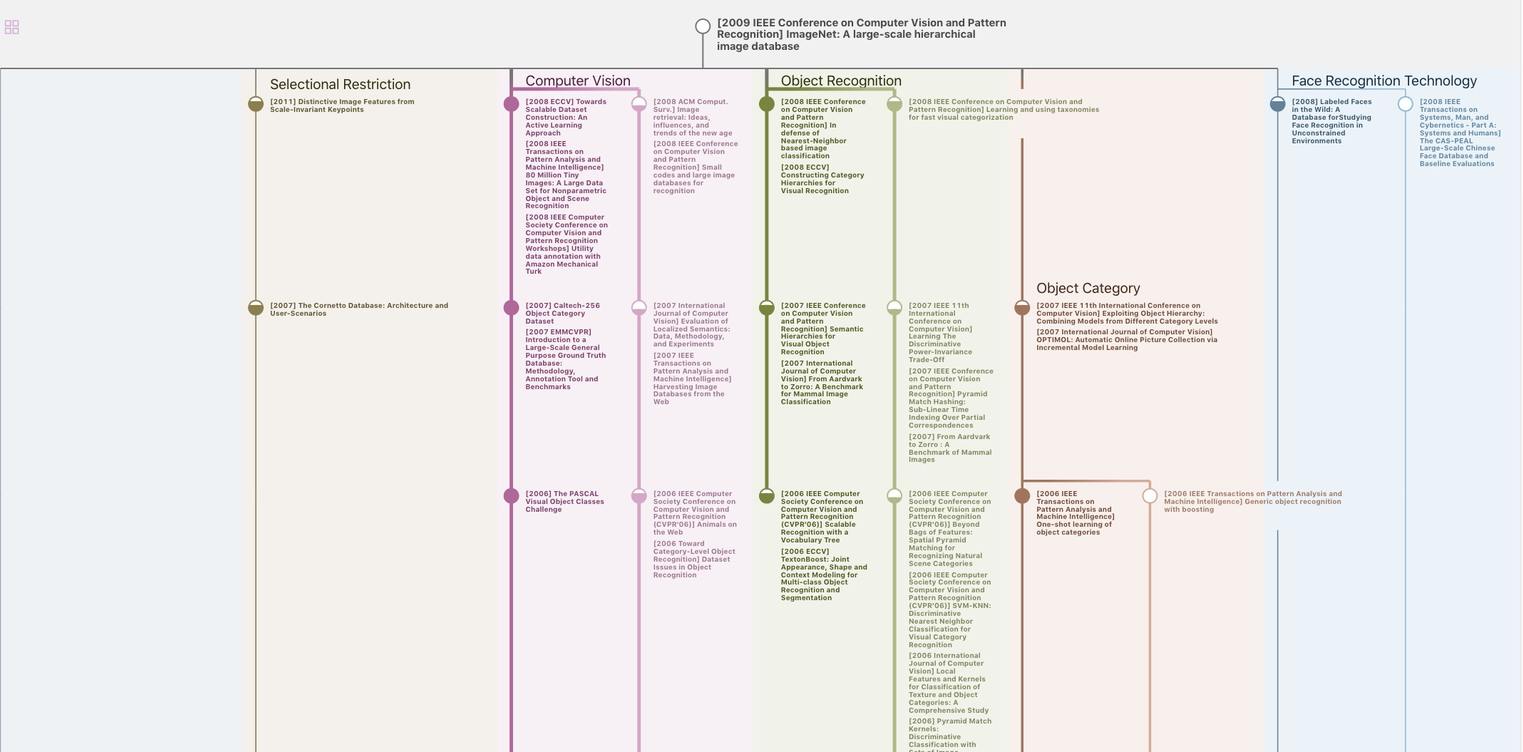
生成溯源树,研究论文发展脉络
Chat Paper
正在生成论文摘要