High-Confidence Sample Generation Technology and Application for Global Land-Cover Classification
IEEE Journal of Selected Topics in Applied Earth Observations and Remote Sensing(2023)
摘要
Deep learning technology has become one of the most important technologies in remote sensing land classification applications. Its powerful sample-learning and information-mining abilities promote the continuous improvement of classification accuracy. A large volume of high-quality and representative sample data is the premise for the successful application of deep learning technology. Conventional methods of obtaining samples through manual delineation or surface surveys require a great deal of manpower and material resources. Therefore, the inability to obtain sufficient and widely representative high-quality samples is one of the key factors limiting the application of deep learning technology. In this study, the method of generating sample data obtains high-confidence classification results from a variety of existing high-quality classification products as deep learning samples, which are then used to support the application of deep learning technology for land-cover classification. When the three global land-cover classification products, FROM-GLC-2015, GLC_FCS30-2015, and GlobeLand30, have the same type of discrimination, the sample is considered a high-confidence sample. Based on this, a large volume of sample data widely distributed around the world was obtained. Using the extracted samples, a random forest classifier was trained using multiple types of information from the Landsat data, and land-cover classification was achieved. Application experiments were conducted in several typical regions, and the classification results were verified. The results showed that the classification accuracy of random forests under the support of samples generated using the sample extraction method proposed in this article was considerably higher than that of the three land-cover classification products.
更多查看译文
关键词
classification,sample,high-confidence,land-cover
AI 理解论文
溯源树
样例
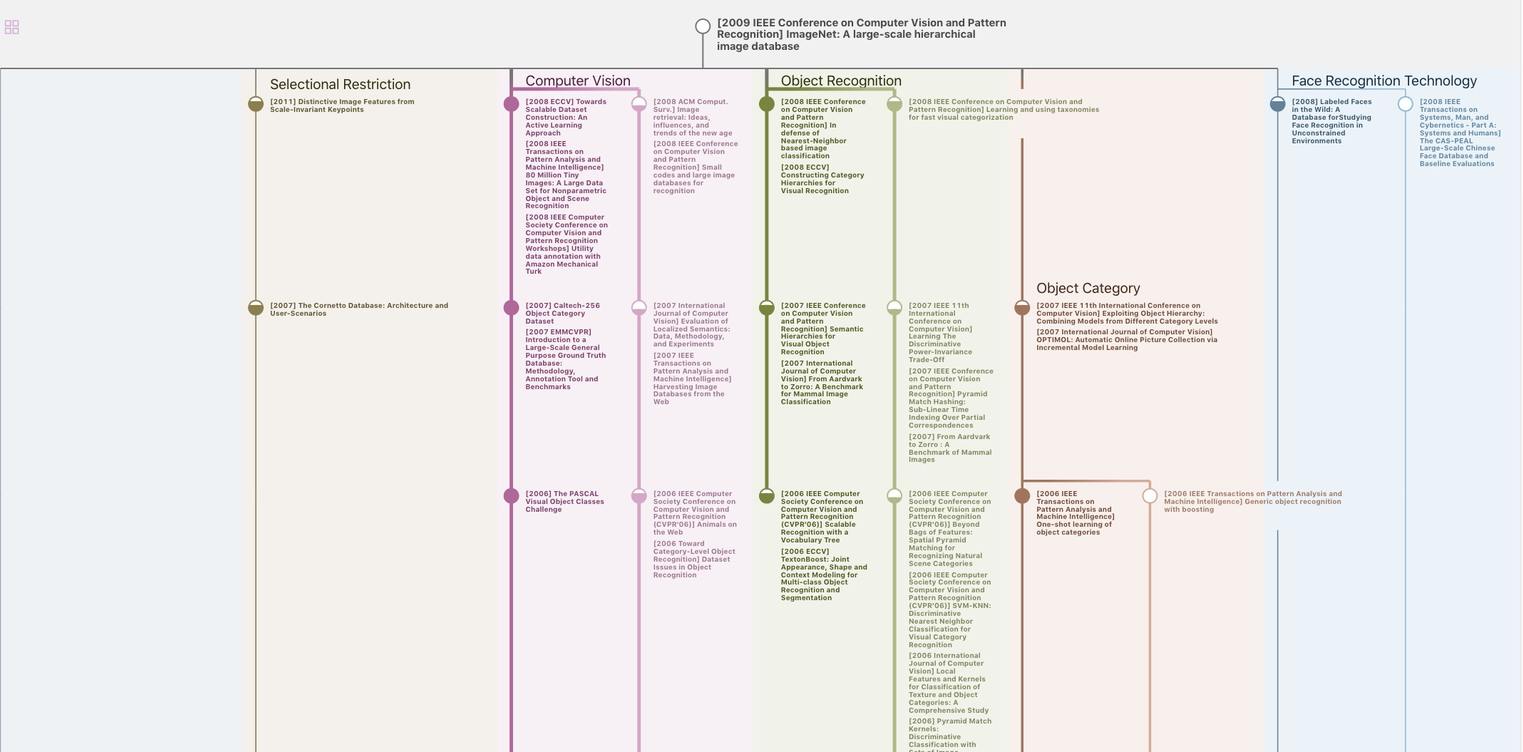
生成溯源树,研究论文发展脉络
Chat Paper
正在生成论文摘要