An Integrated Machine Learning Framework for Classification of Cirrhosis, Fibrosis, and Hepatitis
2022 Third International Conference on Latest trends in Electrical Engineering and Computing Technologies (INTELLECT)(2022)
摘要
Hepatitis C is an ailment that causes inflammation of the liver and leads to serious liver damage. In previous research, the accuracy of the model wasn't that accurate but the differences this paper made model worked well for the prediction of Hepatitis C disease. In the dataset, there are mainly four categories (Blood Donor, Suspected Blood Donor, Fibrosis, and Cirrhosis) used that are labeled. Its data type is polynomial with 0 missing values. The minimum value in the category is 7 for suspect blood donors and the most value is 533 for a blood donor. The machine learning algorithms used in medical approaches are increasing day by day for prediction tools, diagnosis tools, and detection of diseases such as the hepatitis C virus. We used the rapid miner Software for the application of machine learning algorithms. Firstly, took the dataset of the hepatitis C virus from the UCI machine learning site and then applied the five Machine Learning Algorithms, which include Naive Bayes, Random Forest, KNN, Decision Tree & Deep Learning (ANN). On applying feature selection, the attributes Age, ALB, ALP, AST, CHE, GGT, and PROT were selected. After applying different algorithms, the best results are shown by deep learning (ANN) with an accuracy of 95.50%. Rest all algorithms showed minimum accuracy as a Decision tree with 93.09%, Naïve Bayes with 91.89%, KNN with 93.09%, and Random Forest showed 94.29% of high accuracy.
更多查看译文
关键词
Hepatitis C,Cirrhosis,Fibrosis,Machine Learning,Classification
AI 理解论文
溯源树
样例
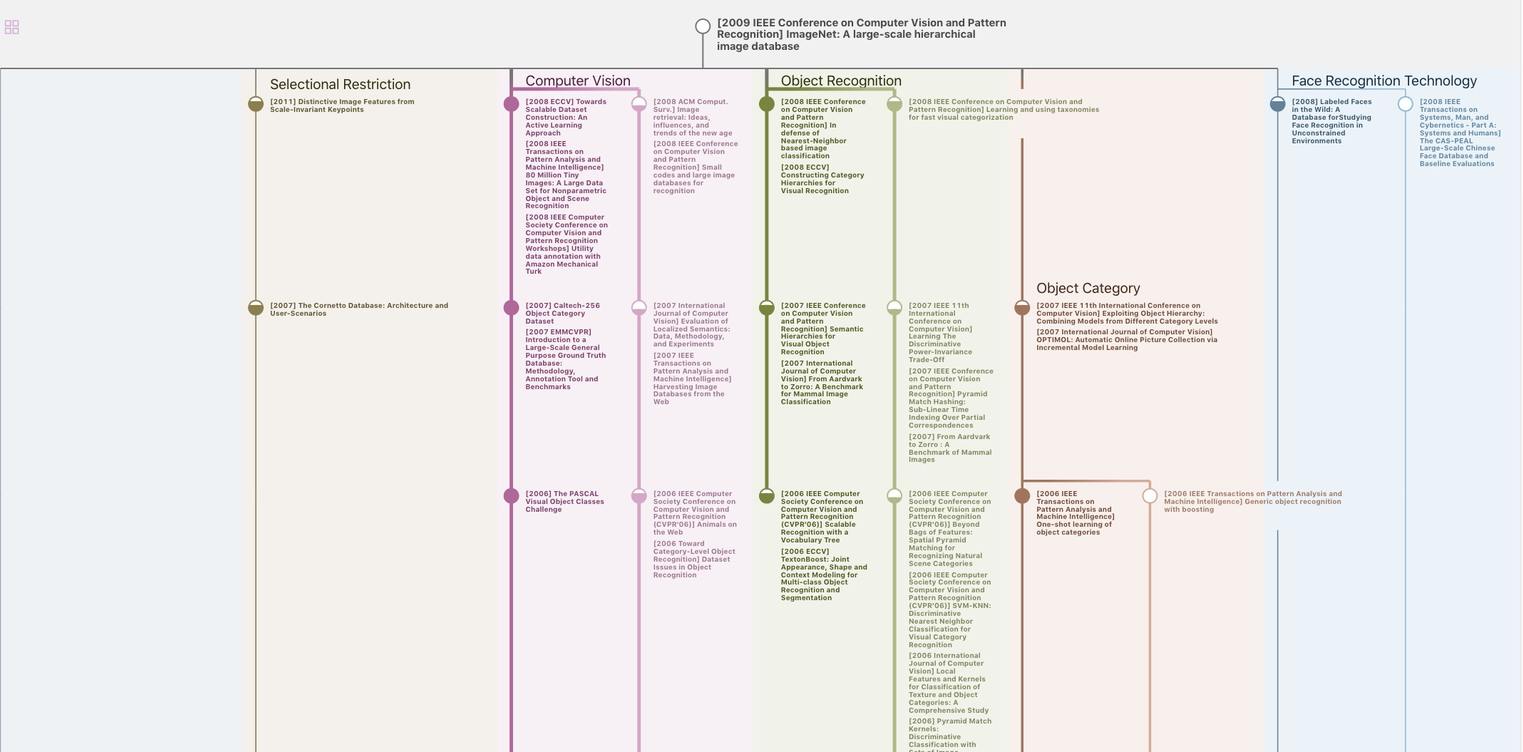
生成溯源树,研究论文发展脉络
Chat Paper
正在生成论文摘要