A Machine Learning Framework for Privacy-Aware Distributed Functional Compression over AWGN Channels
2022 IEEE Information Theory Workshop (ITW)(2022)
摘要
In many diverse fields, distributed IoT devices perform collaborative inference by communicating with an edge router. Often sensory data contains sensitive attributes that should not be revealed to the router. To address this, we develop, to the best of our knowledge, the first privacy-aware machine learning framework for distributed functional compression over AWGN channels. The key feature of our approach to privacy is that we focus only on sensitive attributes of data rather than paying a high cost to protect everything. Employing a mutual information based privacy constraint, we first propose a novel approximate upper bound to protect sensitive attributes in the compressed representations of the sensory data. Next, in conjunction with the upper bound, we propose an adversarial lower bound to enhance the protection further. Thirdly, we propose novel decompositions to these bounds such distributed edge devices can ensure overall privacy by independently privatizing their components. This allows us to propose an enhanced privacy-aware algorithm that protects sensitive information during training and inference. Our experiments show that the privacy-utility trade-off from our proposed methods is significantly better than existing mechanisms.
更多查看译文
关键词
functional compression,machine learning framework,privacy-aware
AI 理解论文
溯源树
样例
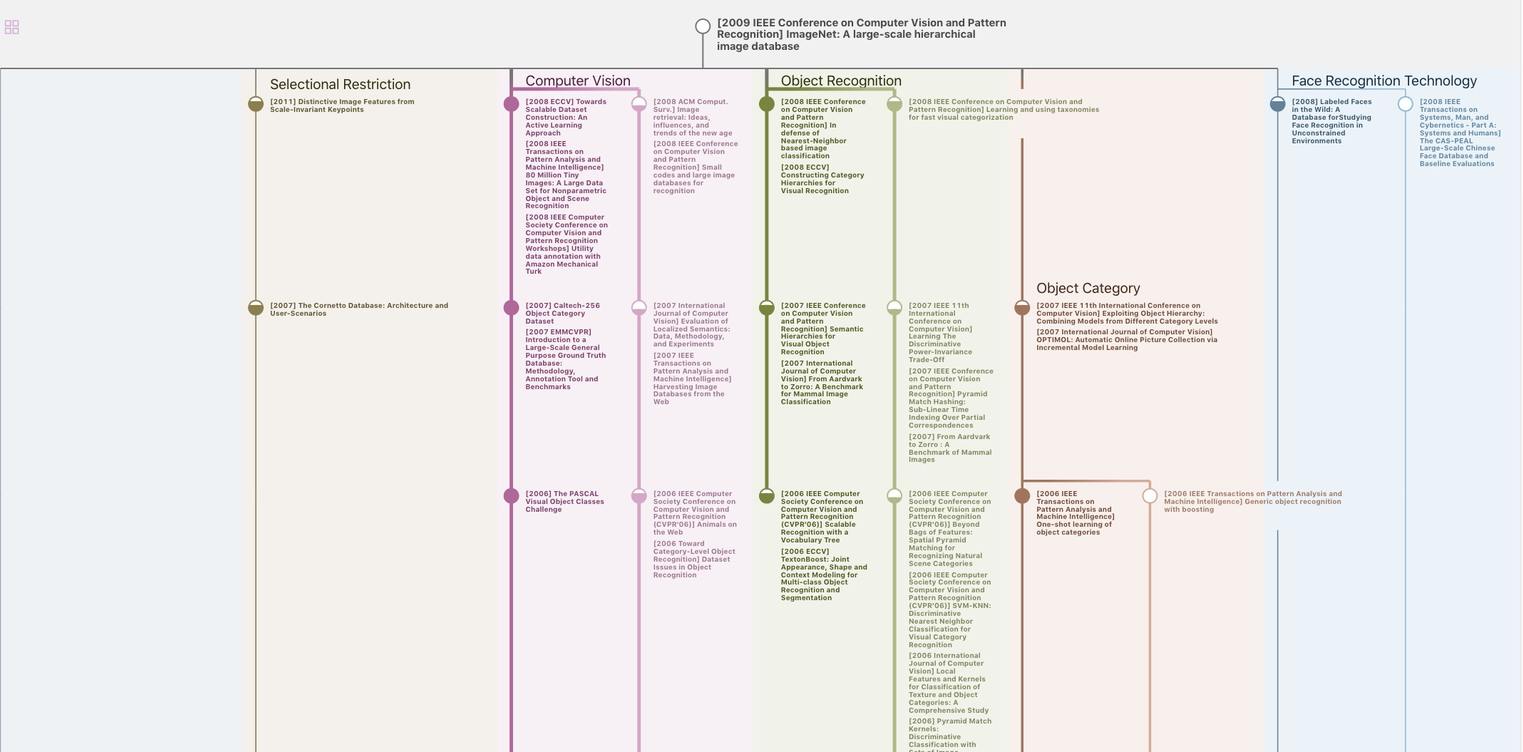
生成溯源树,研究论文发展脉络
Chat Paper
正在生成论文摘要