Discovering Association Rules from Big Graphs.
Proceedings of the VLDB Endowment(2022)
摘要
This paper tackles two challenges to discovery of graph rules. Existing discovery methods often (a) return an excessive number of rules, and (b) do not scale with large graphs given the intractability of the discovery problem. We propose an application-driven strategy to cut back rules and data that are irrelevant to users' interests, by training a machine learning (ML) model to identify data pertaining to a given application. Moreover, we introduce a sampling method to reduce a big graph G to a set H of small sample graphs. Given expected support and recall bounds, the method is able to deduce samples inH and mine rules fromH to satisfy the bounds in the entireG. As proof of concept, we develop an algorithm to discover Graph Association Rules (GARs), which are a combination of graph patterns and attribute dependencies, and may embed ML classifiers as predicates. We show that the algorithm is parallelly scalable, i.e., it guarantees to reduce runtime when more machines are used. We experimentally verify that the method is able to discover rules with recall above 91% when using sample ratio 10%, with speedup of 61 times.
更多查看译文
关键词
big graphs,association
AI 理解论文
溯源树
样例
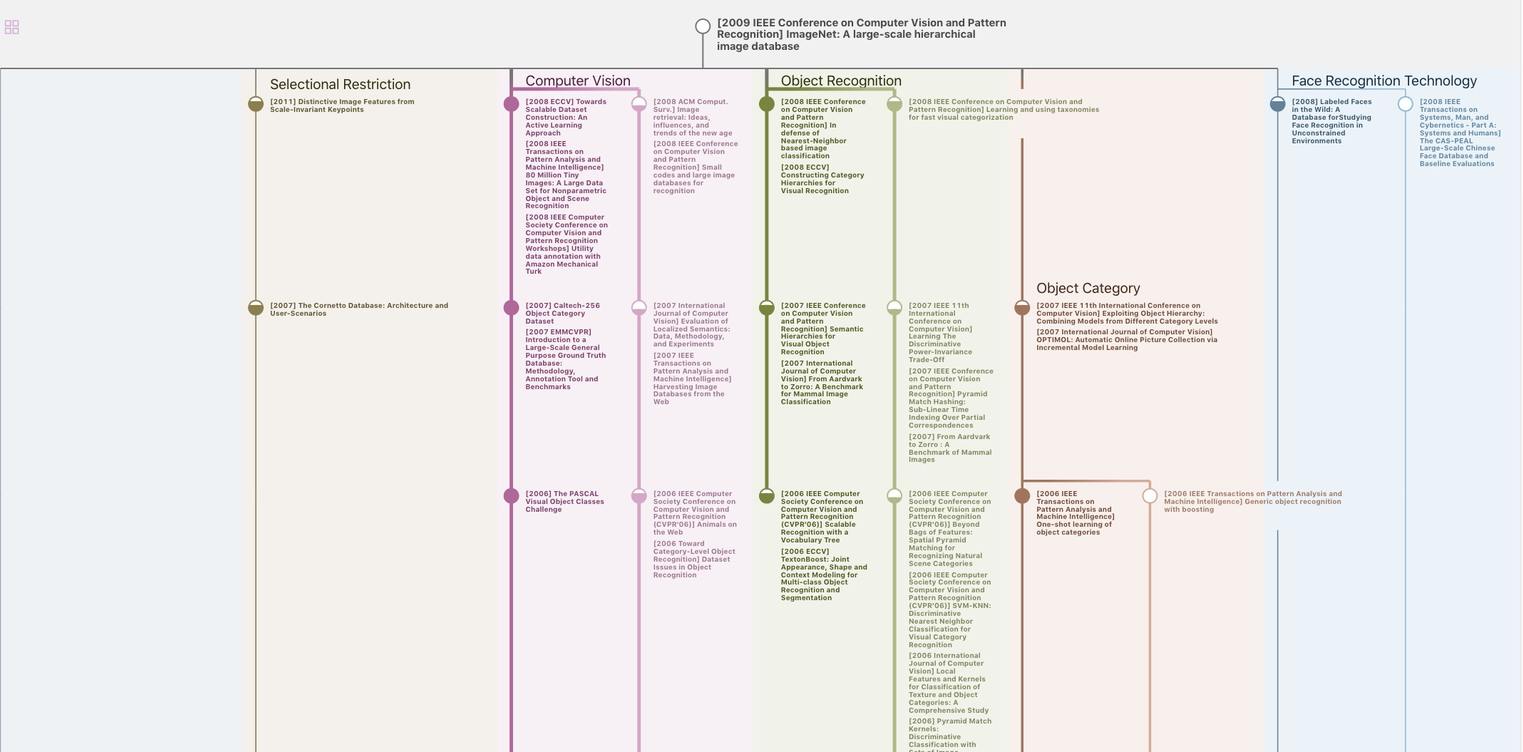
生成溯源树,研究论文发展脉络
Chat Paper
正在生成论文摘要