Crowdsourced Test Report Prioritization Based on Text Classification.
IEEE Access(2022)
摘要
In crowdsourced testing, crowd workers from different places help developers conduct testing and submit test reports for the observed abnormal behaviors. Developers manually inspect each test report and make an initial decision for the potential bug. However, due to the poor quality, test reports are handled extremely slowly. Meanwhile, due to the limitation of resources, some test reports are not handled at all. Therefore, some researchers attempt to resolve the problem of test report prioritization and have proposed many methods. However, these methods do not consider the impact of duplicate test reports. In this paper, we focus on the problem of test report prioritization and present a new method named DivClass by combining a diversity strategy and a classification strategy. First, we leverage Natural Language Processing (NLP) techniques to preprocess crowdsourced test reports. Then, we build a similarity matrix by introducing an asymmetric similarity computation strategy. Finally, we combine the diversity strategy and the classification strategy to determine the inspection order of test reports. To validate the effectiveness of DivClass, experiments are conducted on five crowdsourced test report datasets. Experimental results show that DivClass achieves 0.8887 in terms of APFD (Average Percentage of Fault Detected) and improves the state-of-the-art technique DivRisk by 14.12% on average. The asymmetric similarity computation strategy can improve DivClass by 4.82% in terms of APFD on average. In addition, empirical results show that DivClass can greatly reduce the number of inspected test reports.
更多查看译文
关键词
Computer bugs,Diversity reception,Software,Inspection,Task analysis,Software testing,Smart phones,Crowdsourced testing,test report prioritization,text classification,diversity strategy,asymmetric similarity
AI 理解论文
溯源树
样例
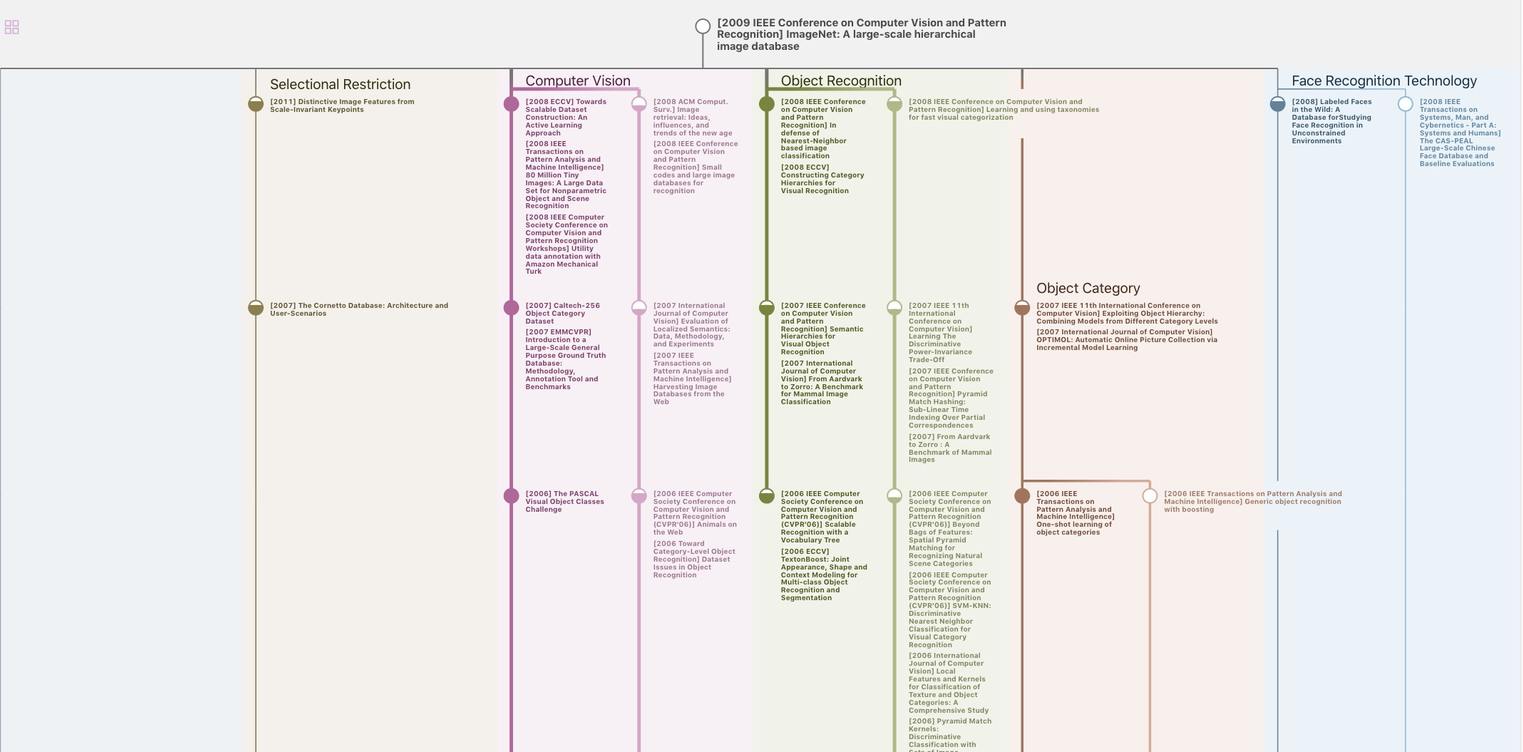
生成溯源树,研究论文发展脉络
Chat Paper
正在生成论文摘要