RADAR: A TTP-based Extensible, Explainable, and Effective System for Network Traffic Analysis and Malware Detection
PROCEEDINGS OF THE 2023 EUROPEAN INTERDISCIPLINARY CYBERSECURITY CONFERENCE, EICC 2023(2023)
摘要
Network analysis and machine learning techniques have been widely applied for building malware detection systems. Though these systems attain impressive results, they often are (i) not extensible, being monolithic, well tuned for the specific task they have been designed for but very difficult to adapt and/or extend to other settings, and (ii) not interpretable, being black boxes whose inner complexity makes it impossible to link the result of detection with its root cause, making further analysis of threats a challenge. In this paper we present RADAR, an extensible and explainable system that exploits the popular TTP (Tactics, Techniques, and Procedures) ontology of adversary behaviour described in the industrystandard MITRE ATT&CK framework in order to unequivocally identify and classify malicious behaviour using network traffic. We evaluate RADAR on a very large dataset comprising of 2,286,907 malicious and benign samples, representing a total of 84,792,452 network flows. The experimental analysis confirms that the proposed methodology can be effectively exploited: RADAR's ability to detect malware is comparable to other state-of-the-art non-interpretable systems' capabilities. To the best of our knowledge, RADAR is the first TTP-based system for malware detection that uses machine learning while being extensible and explainable.
更多查看译文
关键词
Malware,Cyber Security,Explainable AI,Network Analysis
AI 理解论文
溯源树
样例
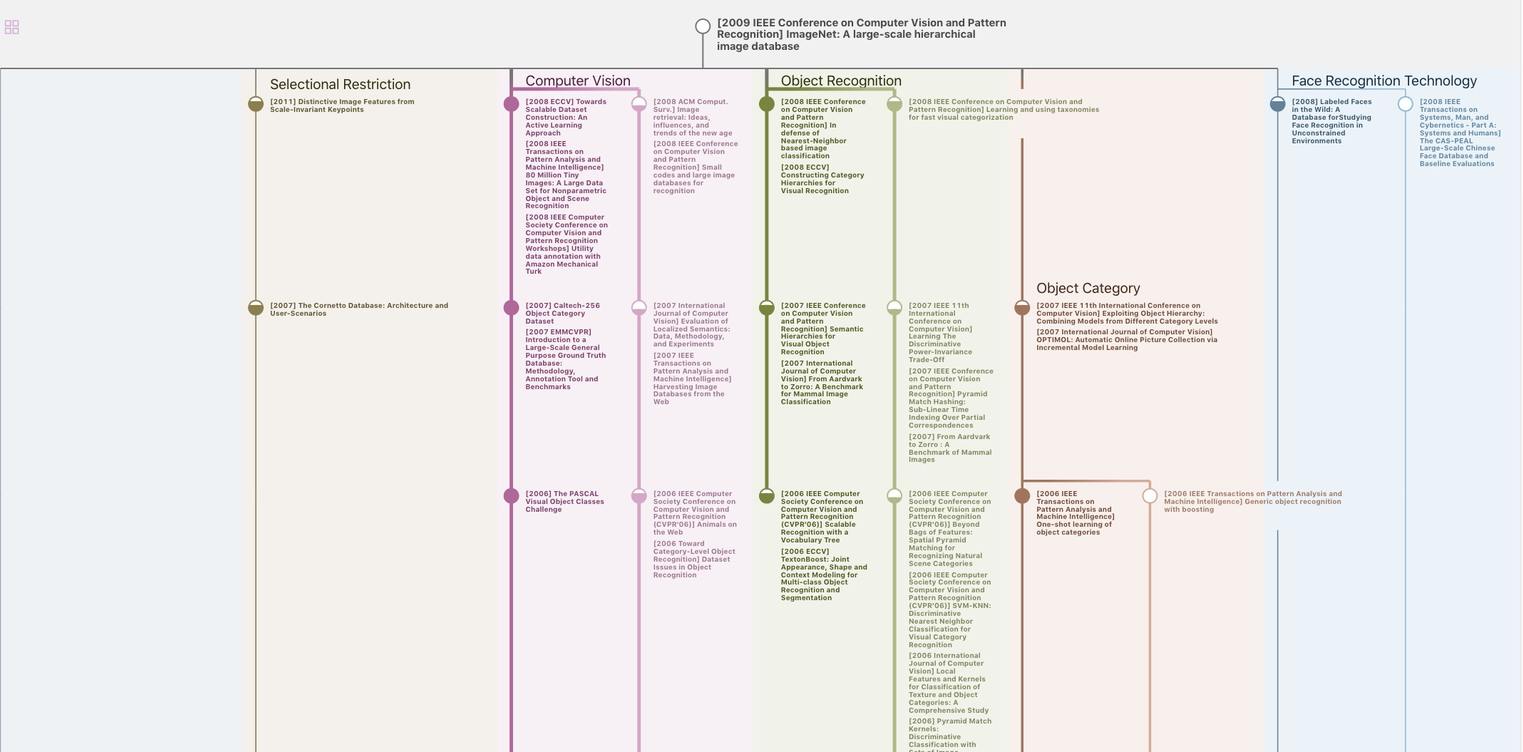
生成溯源树,研究论文发展脉络
Chat Paper
正在生成论文摘要