Reconstructing Training Data from Model Gradient, Provably
arxiv(2023)
摘要
Understanding when and how much a model gradient leaks information about the training sample is an important question in privacy. In this paper, we present a surprising result: even without training or memorizing the data, we can fully reconstruct the training samples from a single gradient query at a randomly chosen parameter value. We prove the identifiability of the training data under mild conditions: with shallow or deep neural networks and a wide range of activation functions. We also present a statistically and computationally efficient algorithm based on tensor decomposition to reconstruct the training data. As a provable attack that reveals sensitive training data, our findings suggest potential severe threats to privacy, especially in federated learning.
更多查看译文
关键词
model gradient,training data
AI 理解论文
溯源树
样例
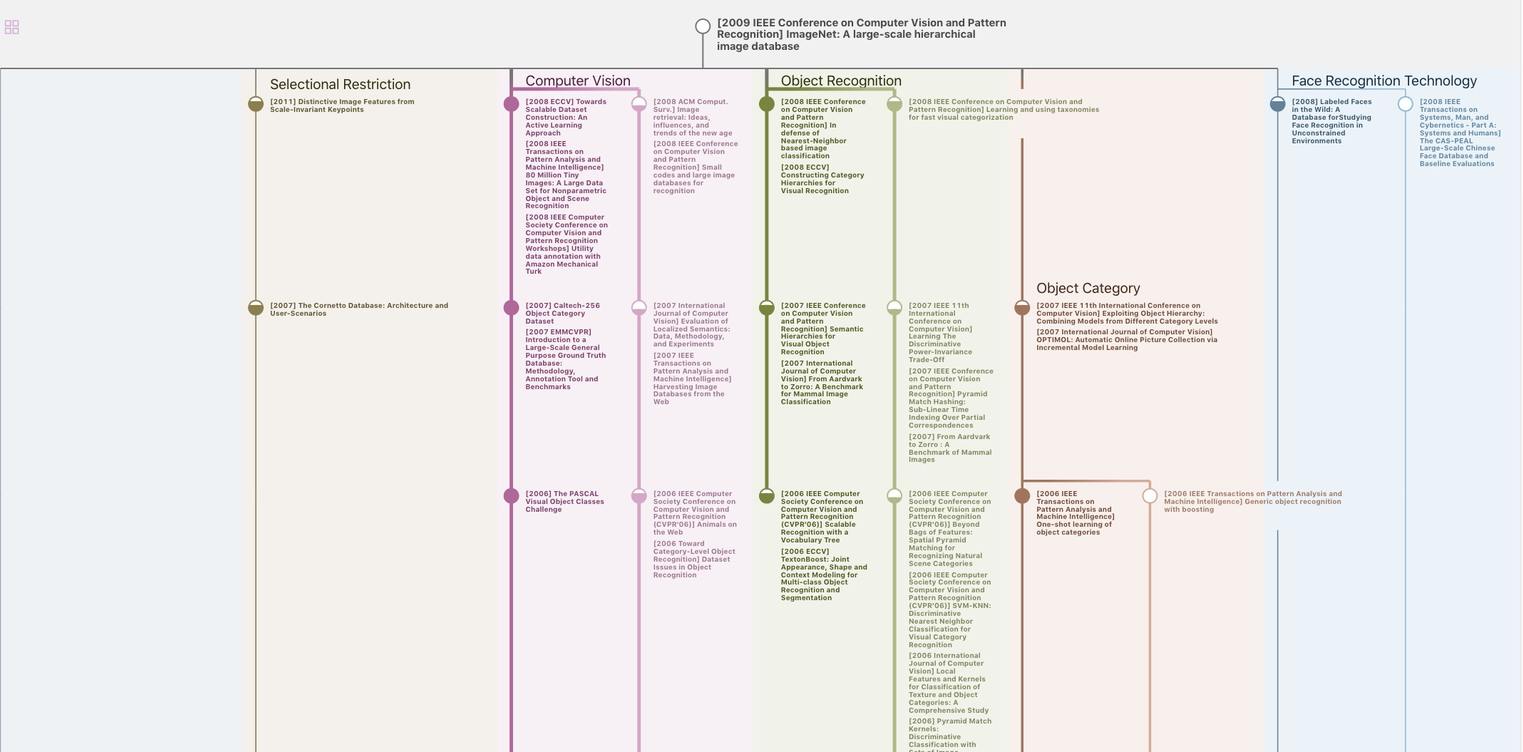
生成溯源树,研究论文发展脉络
Chat Paper
正在生成论文摘要