AsyInst: Asymmetric Affinity with DepthGrad and Color for Box-Supervised Instance Segmentation
arxiv(2022)
摘要
The weakly supervised instance segmentation is a challenging task. The existing methods typically use bounding boxes as supervision and optimize the network with a regularization loss term such as pairwise color affinity loss for instance segmentation. Through systematic analysis, we found that the commonly used pairwise affinity loss has two limitations: (1) it works with color affinity but leads to inferior performance with other modalities such as depth gradient, (2)the original affinity loss does not prevent trivial predictions as intended but actually accelerates this process due to the affinity loss term being symmetric. To overcome these two limitations, in this paper, we propose a novel asymmetric affinity loss which provides the penalty against the trivial prediction and generalizes well with affinity loss from different modalities. With the proposed asymmetric affinity loss, our method outperforms the state-of-the-art methods on the Cityscapes dataset and outperforms our baseline method by 3.5% in mask AP.
更多查看译文
关键词
instance segmentation,asymmetric affinity,depthgrad,box-supervised
AI 理解论文
溯源树
样例
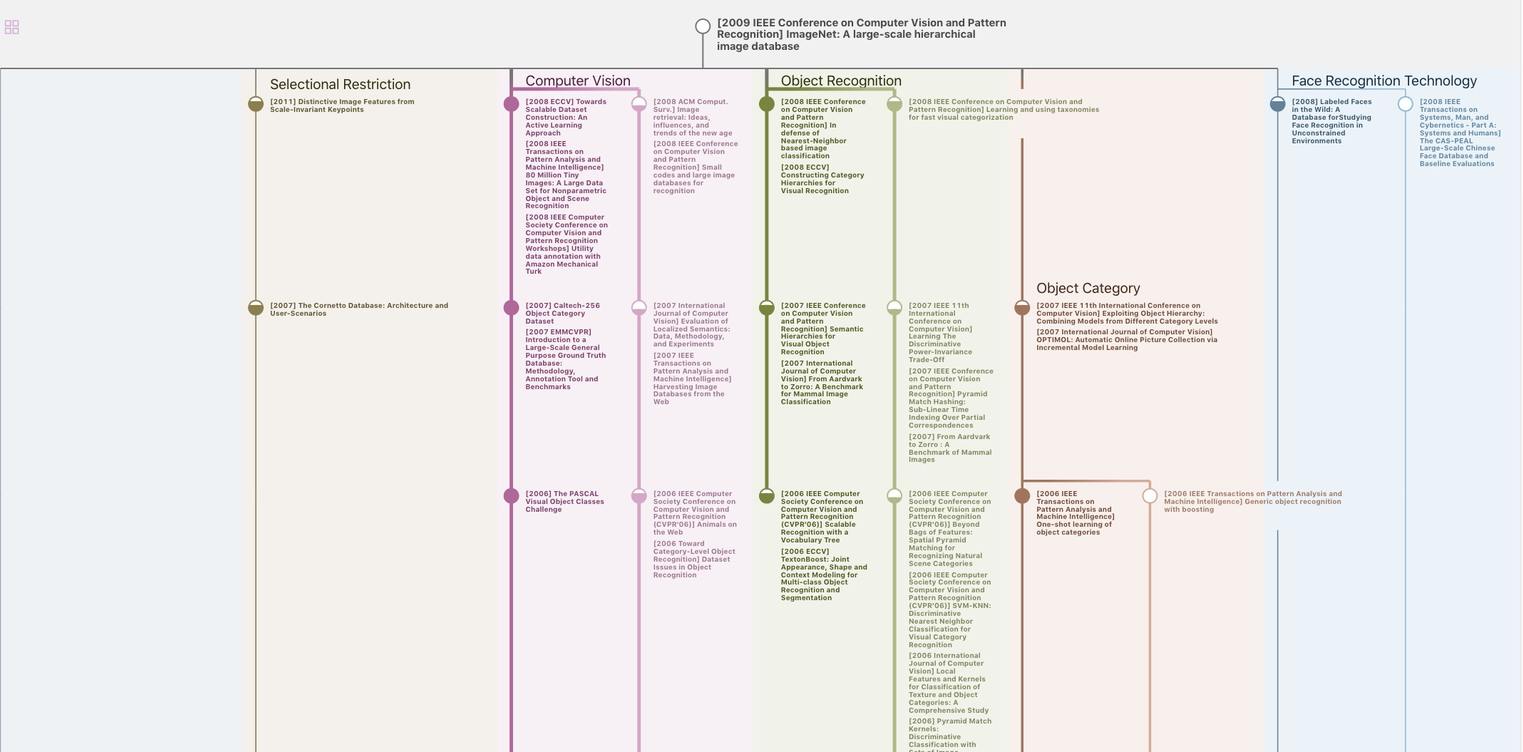
生成溯源树,研究论文发展脉络
Chat Paper
正在生成论文摘要