Progressive Multi-scale Self-supervised Learning for Speech Recognition
2023 ASIA PACIFIC SIGNAL AND INFORMATION PROCESSING ASSOCIATION ANNUAL SUMMIT AND CONFERENCE, APSIPA ASC(2023)
摘要
Self-supervised learning has shown great potential in improving automatic speech recognition (ASR) systems. However, further improvements in recognition performance could be achieved if models focus on audio content information learning. In this paper, we propose a progressive multi-scale self-supervised learning method that reinforces the learning process from easy to difficult. Our progressive strategy utilizes fine-grained target sets to compute self-supervised learning loss at the top layer while using coarse-grained target sets at intermediate layers. Additionally, to match the difficulty of the learning process, we introduce a multi-scale structure into the multi-head self-attention module. We evaluate our method on the Librispeech dataset and demonstrate its effectiveness. Our proposed method achieves a relative word error rate (WER) reduction of 13.7% and 12.7% on the test other evaluation subsets, respectively, when fine-tuned on 10-hour and 100-hour subsets, outperforming HuBERT.
更多查看译文
AI 理解论文
溯源树
样例
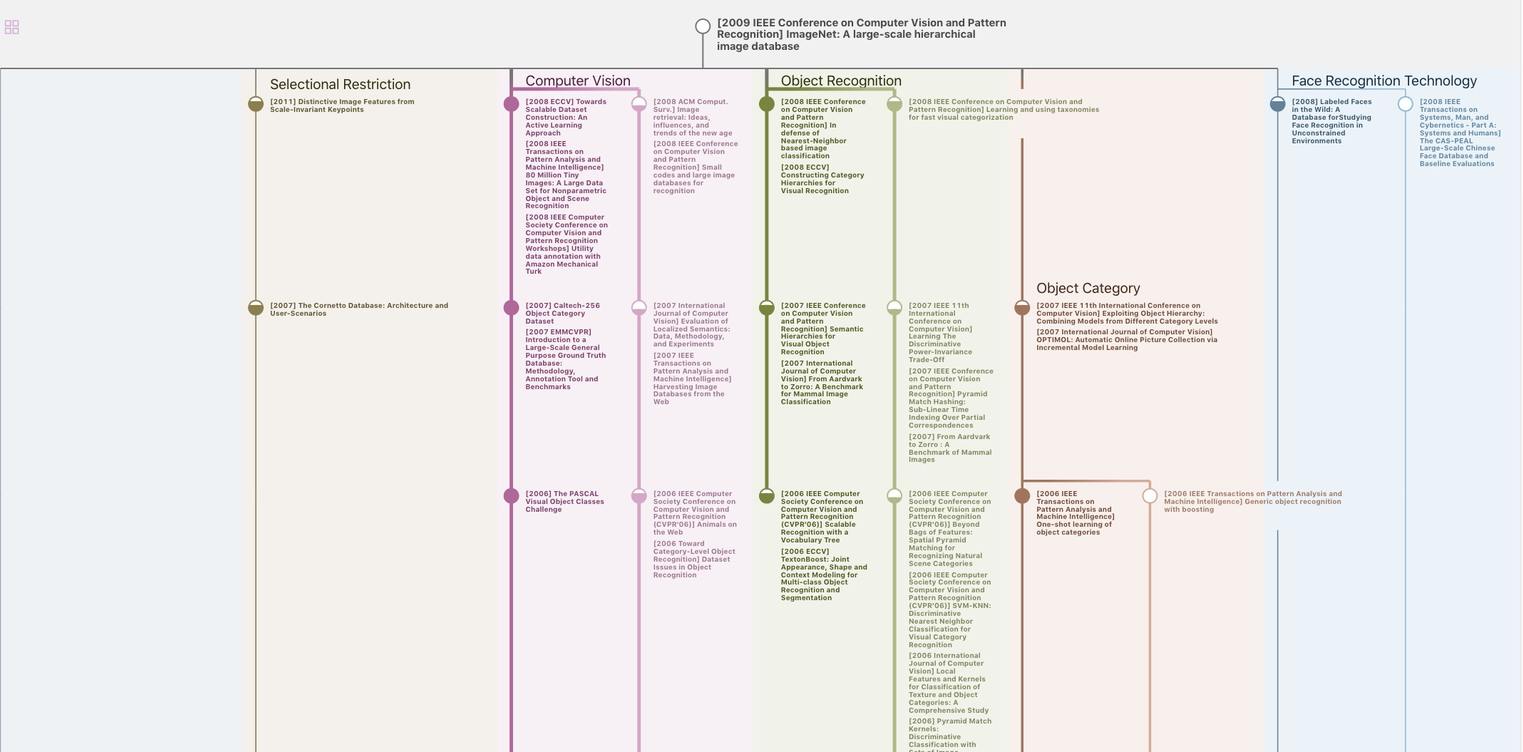
生成溯源树,研究论文发展脉络
Chat Paper
正在生成论文摘要