Relation-based Motion Prediction using Traffic Scene Graphs.
International Conference on Intelligent Transportation Systems (ITSC)(2022)
摘要
Representing relevant information of a traffic scene and understanding its environment is crucial for the success of autonomous driving. Modeling the surrounding of an autonomous car using semantic relations, i.e., how different traffic participants relate in the context of traffic rule based behaviors, is hardly been considered in previous work. This stems from the fact that these relations are hard to extract from real-world traffic scenes. In this work, we model traffic scenes in a form of spatial semantic scene graphs for various different predictions about the traffic participants, e.g., acceleration and deceleration. Our learning and inference approach uses Graph Neural Networks (GNNs) and shows that incorporating explicit information about the spatial semantic relations between traffic participants improves the predicdtion results. Specifically, the acceleration prediction of traffic participants is improved by up to 12% compared to the baselines, which do not exploit this explicit information. Furthermore, by including additional information about previous scenes, we achieve 73% improvements.
更多查看译文
关键词
motion prediction,graphs,relation-based
AI 理解论文
溯源树
样例
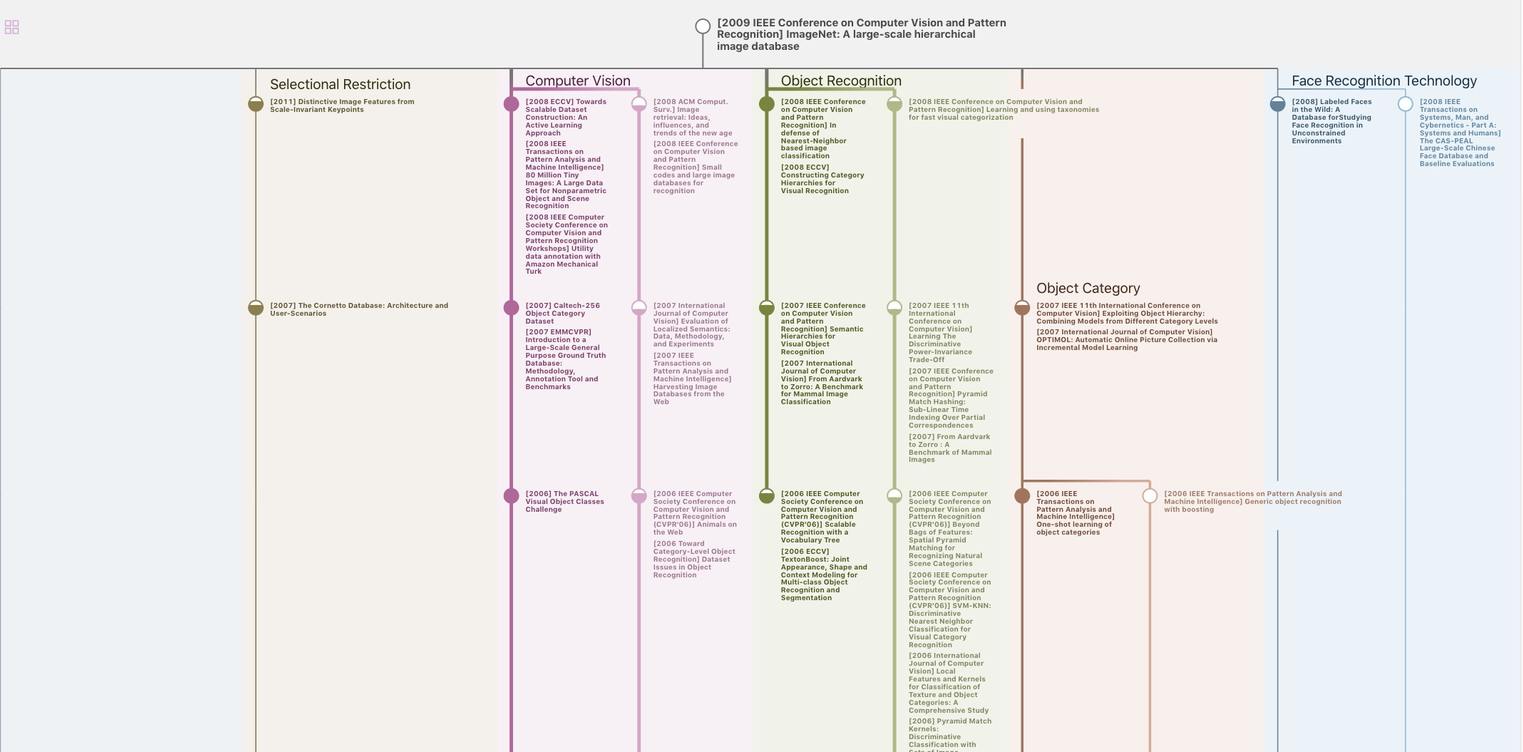
生成溯源树,研究论文发展脉络
Chat Paper
正在生成论文摘要