Graph Multi-Attention Network-based Taxi Demand Prediction
2022 4th International Conference on Data-driven Optimization of Complex Systems (DOCS)(2022)
摘要
Taxi is an important component of the urban transport system in most cities. Accurate taxi demand prediction can effectively reduce the waiting time of passengers and shorten the no-load travel of drivers, which is helpful in alleviating traffic congestion and improving traffic efficiency. Due to the complexity of the traffic system and spatiotemporal dependencies among regions in a road network, traditional prediction methods cannot predict taxi demands of different regions effectively. This paper introduces a Graph Multi-Attention Network (GMAN) to handle the taxi demand prediction problem with better performance, which aims to predict the taxi demands in all regions of a road network in the next time period. The effectiveness of the GMAN is validated based on a large-scale dataset of taxi demands from a real urban road network. Experimental results show that the GMAN outperforms 5 commonly used benchmarking models, including 3 state-of-the-art machine learning models.
更多查看译文
关键词
Deep Learning,Taxi Demand Prediction,GMAN,Attention Mechanism
AI 理解论文
溯源树
样例
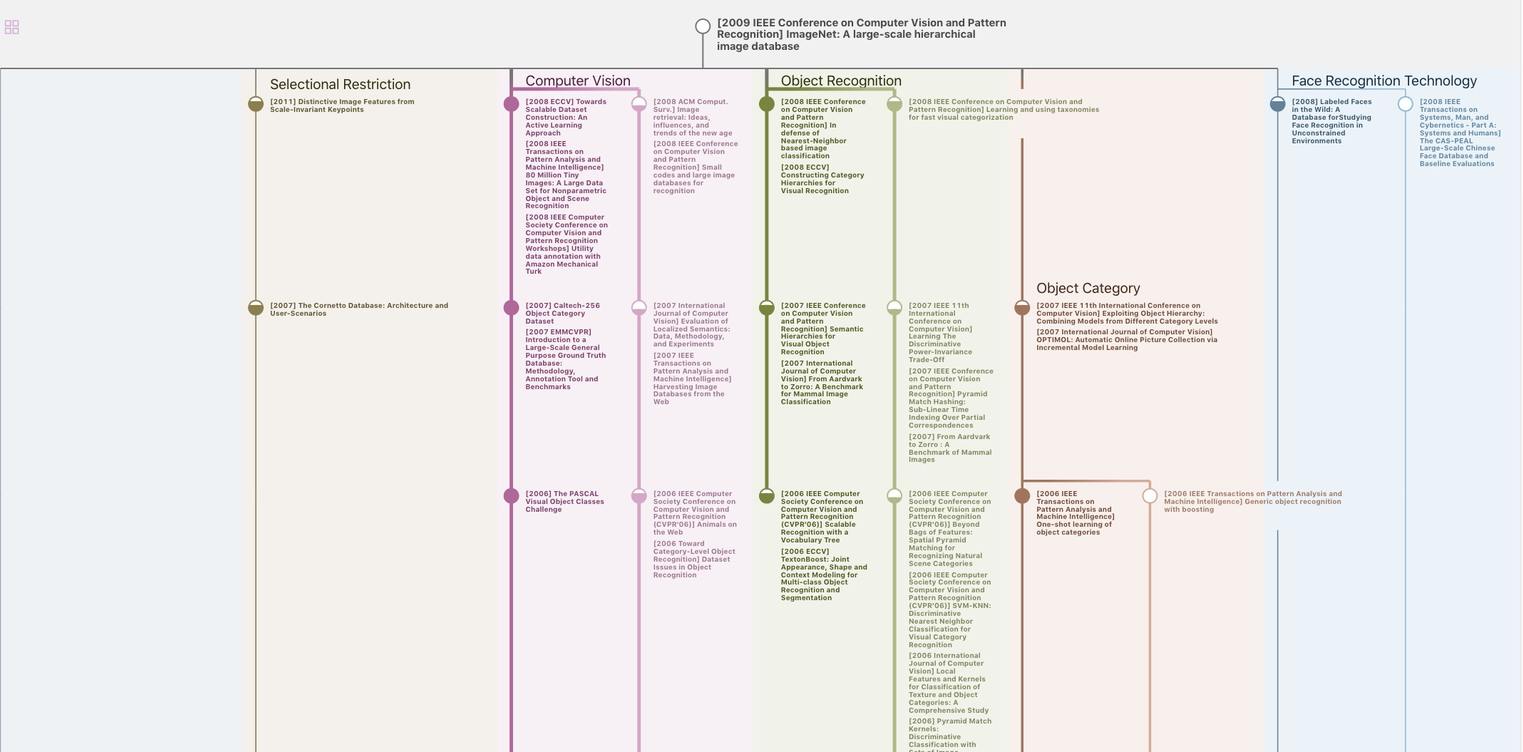
生成溯源树,研究论文发展脉络
Chat Paper
正在生成论文摘要