Improved Convergence Guarantees for Shallow Neural Networks
arxiv(2022)
摘要
We continue a long line of research aimed at proving convergence of depth 2 neural networks, trained via gradient descent, to a global minimum. Like in many previous works, our model has the following features: regression with quadratic loss function, fully connected feedforward architecture, RelU activations, Gaussian data instances and network initialization, adversarial labels. It is more general in the sense that we allow both layers to be trained simultaneously and at {\em different} rates. Our results improve on state-of-the-art [Oymak Soltanolkotabi 20] (training the first layer only) and [Nguyen 21, Section 3.2] (training both layers with Le Cun's initialization). We also report several simple experiments with synthetic data. They strongly suggest that, at least in our model, the convergence phenomenon extends well beyond the ``NTK regime''.
更多查看译文
关键词
improved convergence guarantees,neural networks,shallow
AI 理解论文
溯源树
样例
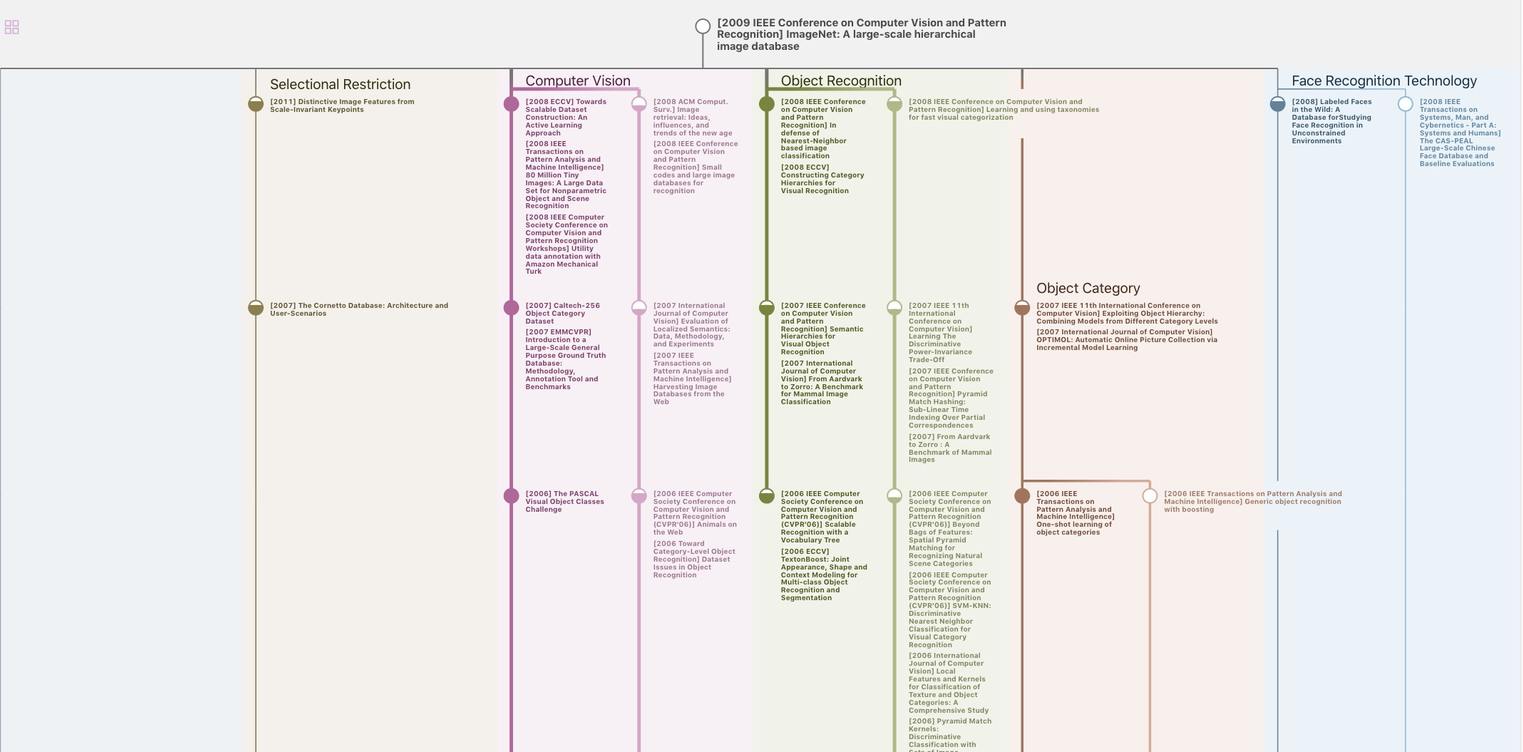
生成溯源树,研究论文发展脉络
Chat Paper
正在生成论文摘要