Day2Dark: Pseudo-Supervised Activity Recognition beyond Silent Daylight
arxiv(2022)
摘要
State-of-the-art activity recognizers are effective during the day, but not trustworthy in the dark. The main causes are the distribution shift from the lower color contrast as well as the limited availability of labeled dark videos. Our goal is to recognize activities in the dark as well as in the day. To compensate for the lack of labeled dark videos, we introduce a pseudo-supervised learning scheme, which utilizes task-irrelevant unlabeled dark videos to train an activity recognizer. Our proposed activity recognizer makes use of audio which is invariant to illumination. However, the usefulness of audio and visual features differs according to the illumination. Thus we propose to make our audio-visual recognizer `darkness-aware'. Experiments on EPIC-Kitchens, Kinetics-Sound, and Charades demonstrate that our proposals enable effective activity recognition in the dark and can even improve robustness to occlusions.
更多查看译文
关键词
silent daylight,activity,day2dark,recognition,pseudo-supervised
AI 理解论文
溯源树
样例
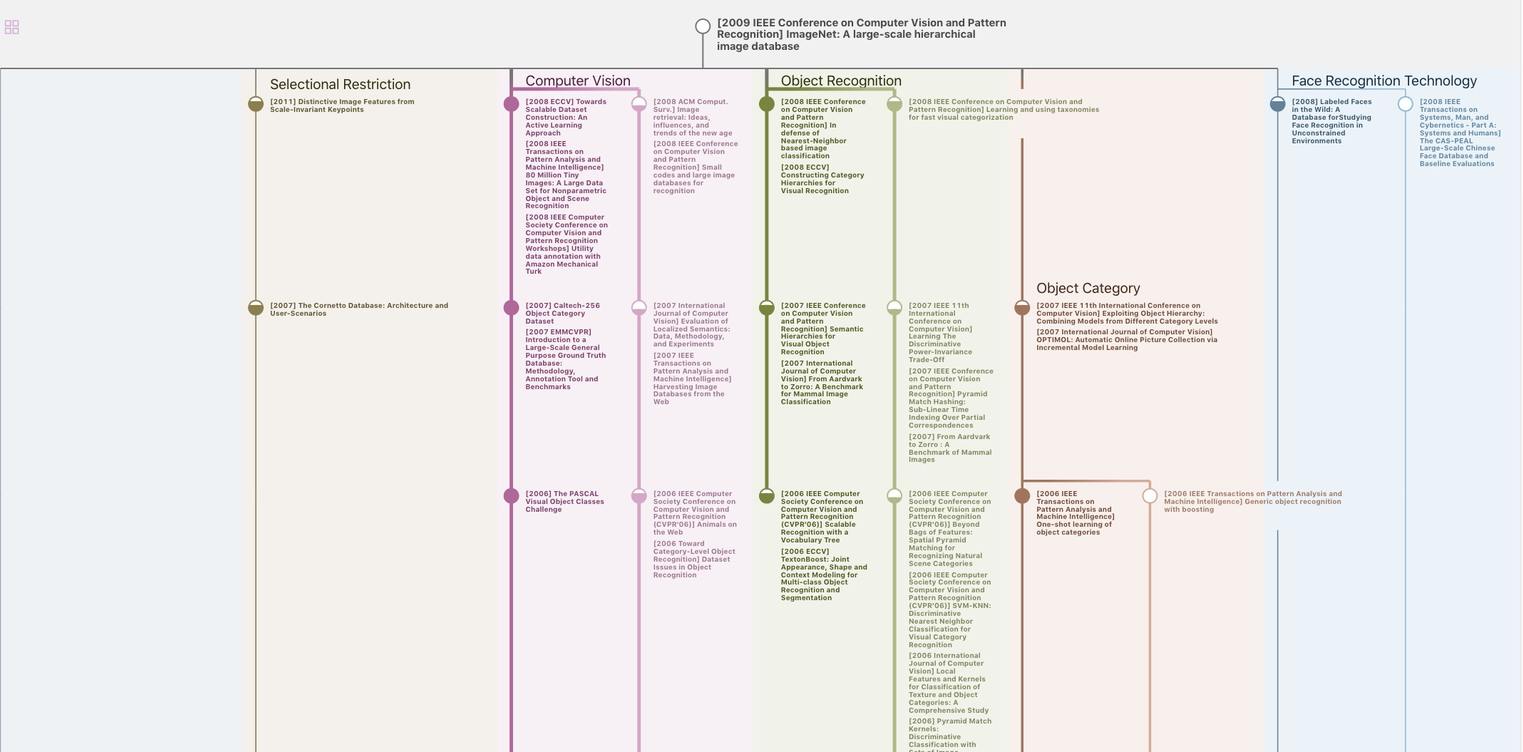
生成溯源树,研究论文发展脉络
Chat Paper
正在生成论文摘要