Security Analysis of SplitFed Learning
arxiv(2022)
摘要
Split Learning (SL) and Federated Learning (FL) are two prominent distributed collaborative learning techniques that maintain data privacy by allowing clients to never share their private data with other clients and servers, and find extensive IoT applications in smart healthcare, smart cities and smart industry. Prior work has extensively explored the security vulnerabilities of FL in the form of poisoning attacks. To mitigate the effect of these attacks, several defenses have also been proposed. Recently, a hybrid of both learning techniques has emerged (commonly known as SplitFed) that capitalizes on their advantages (fast training) and eliminates their intrinsic disadvantages (centralized model updates). In this paper, we perform the first empirical analysis of SplitFed's robustness to strong model poisoning attacks. We observe that the model updates in SplitFed have significantly smaller dimensionality as compared to FL that is known to have curse of dimensionality. We show that large models that have higher dimensionality are more susceptible to privacy and security attacks, whereas the clients in SplitFed do not have the complete model and have lower dimensionality, making them more robust to existing model poisoning attacks. Our results show that the accuracy reduction due to the model poisoning attack is 5x lower for SplitFed compared to FL.
更多查看译文
关键词
splitfed learning,security
AI 理解论文
溯源树
样例
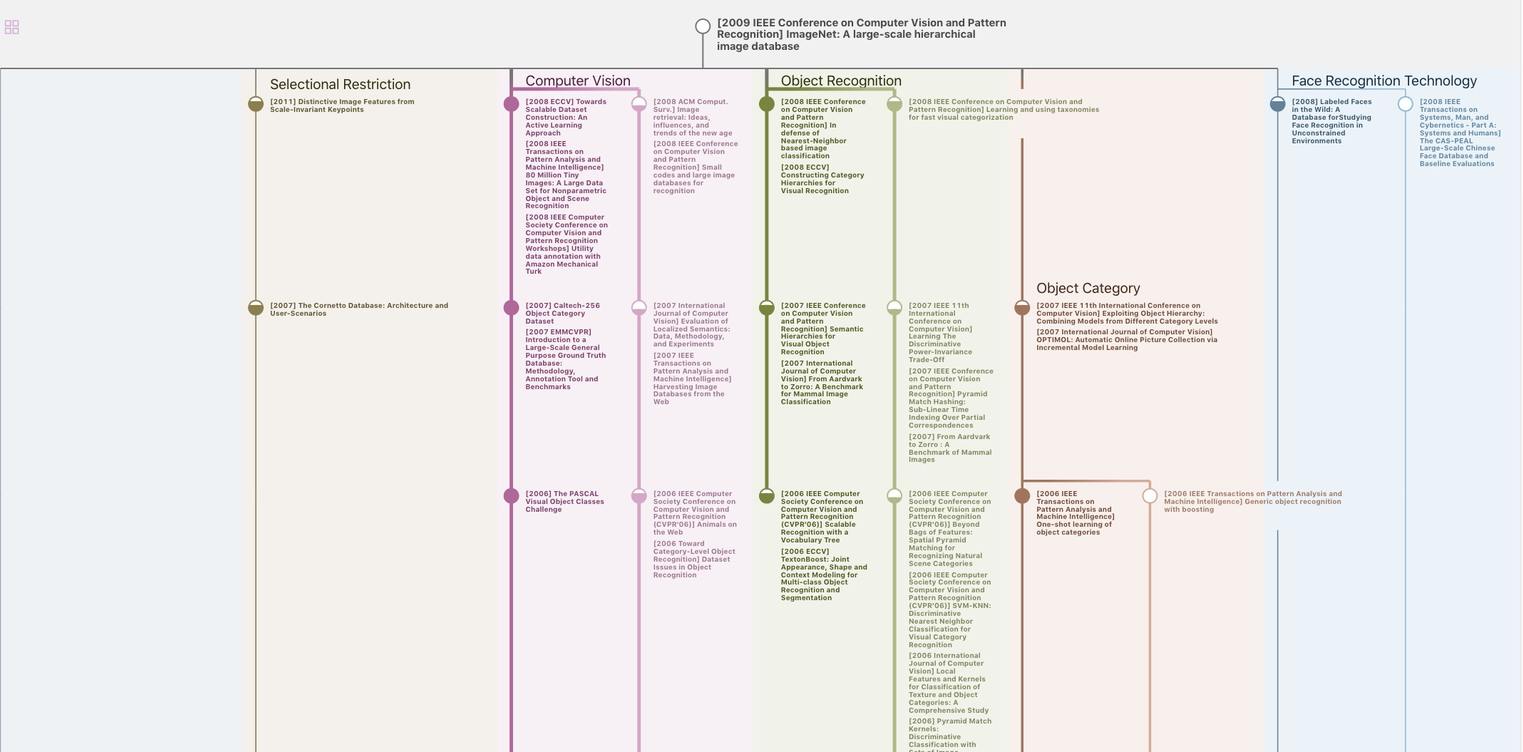
生成溯源树,研究论文发展脉络
Chat Paper
正在生成论文摘要