Deep Learning–Based Bathymetry Mapping from Multispectral Satellite Data Around Europa Island
European Spatial Data for Coastal and Marine Remote Sensing(2022)
摘要
Bathymetry studies are important to monitor the changes occurring in coastal topographies, to update navigation charts, and to understand the dynamics of the marine environment. Satellite-derived bathymetry enables rapid mapping of large coastal areas through measurement of optical penetration of the water column. In this study, bathymetry prediction is investigated using Pleiades multispectral satellite data. This research work explores the possibility of using very-high-resolution multispectral satellite data with a deep learning U-Net-inspired neural network architecture to infer bathymetry estimates around Europa Island (22o20′S, 40o22′E), which is a coralline island in the Mozambique Channel. This study is among the first to provide an overview suitable for bathymetry mapping using a deep learning approach based on optical satellite data. An airborne light detection and ranging (LiDAR) dataset of 1 m resolution is used as ground truth to train the model. From experiments, the overall accuracy evaluation of the model shows a good relationship (R2 = 0.99, standard error = 0.492) between the predicted and reference depth values that satisfy the International Hydrographic Organization (IHO) S-57 Category of Zone of Confidence (CATZOC) levels A1, A2, B, and C (IHO, 2014). These predicted bathymetry values could potentially be incorporated into electronic navigational charts. The image reconstruction shows accurate results to estimate bathymetry in the shallow waters with mean absolute error not exceeding 1.5 m in that case. The U-Net-inspired deep learning technique exhibits promising outcomes to predict water depth from very-high-resolution satellite data to operate bathymetry mapping automatically over a wide area.
更多查看译文
关键词
Bathymetry mapping, Europa Island, Pleiades satellite, LiDAR, Deep learning, U-Net architecture, Remote sensing
AI 理解论文
溯源树
样例
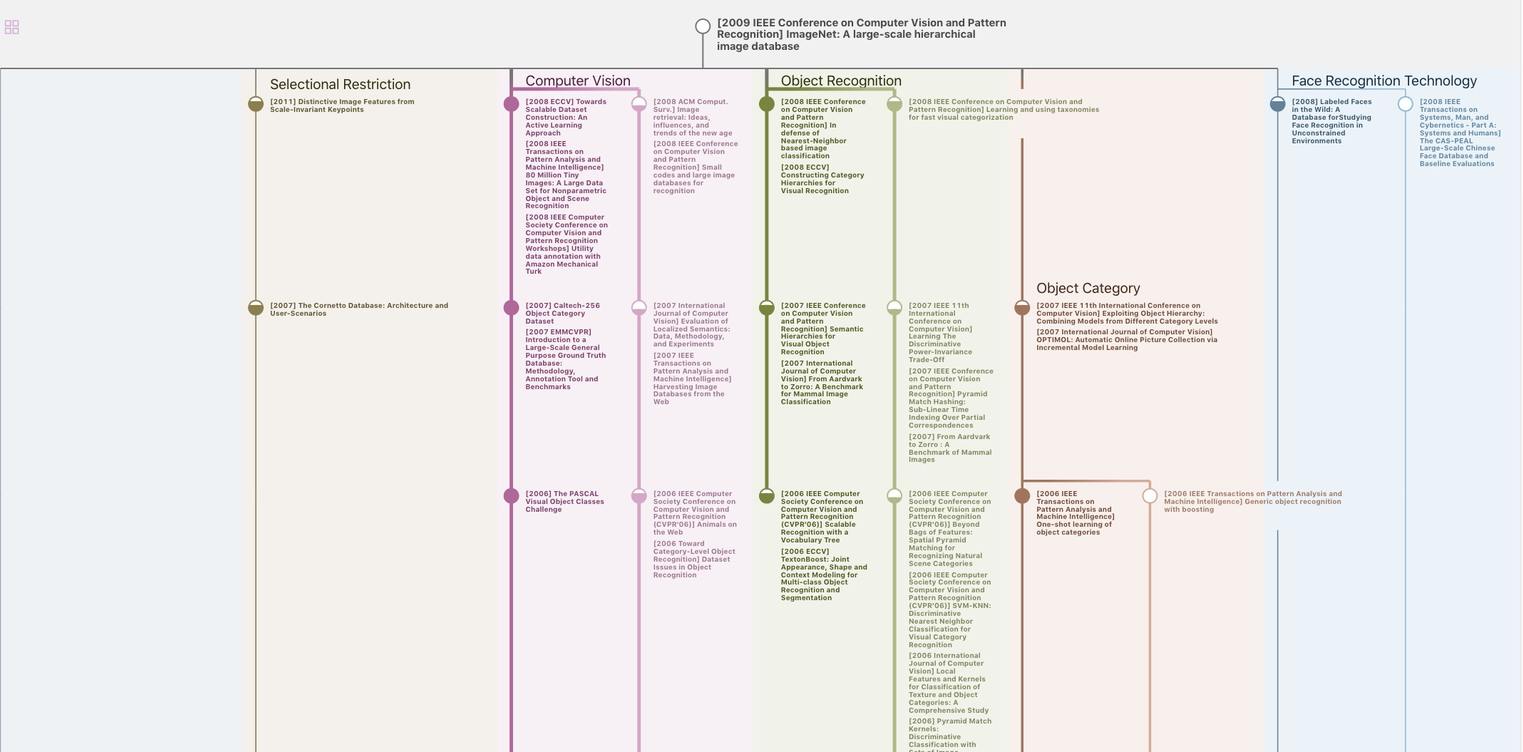
生成溯源树,研究论文发展脉络
Chat Paper
正在生成论文摘要