Time Series Predictive Models for Opponent Behavior Modeling in Bilateral Negotiations
PRIMA 2022: Principles and Practice of Multi-Agent Systems(2022)
摘要
In agent-based negotiations, it is crucial to understand the opponent’s behavior and predict its bidding pattern to act strategically. Foreseeing the utility of the opponent’s coming offer provides valuable insight to the agent so that it can decide its next move wisely. Accordingly, this paper addresses predicting the opponent’s coming offers by employing two deep learning-based approaches: Long Short-Term Memory Networks and Transformers. The learning process has three different targets: estimating the agent’s utility of the opponent’s coming offer, estimating the agent’s utility of that without using opponent-related variables, and estimating the opponent’s utility of that by using opponent-related variables. This work reports the performances of these models that are evaluated in various negotiation scenarios. Our evaluation showed promising results regarding the prediction performance of the proposed methods.
更多查看译文
关键词
Automated negotiation, Multi-agent systems, Time-series prediction, Utility prediction
AI 理解论文
溯源树
样例
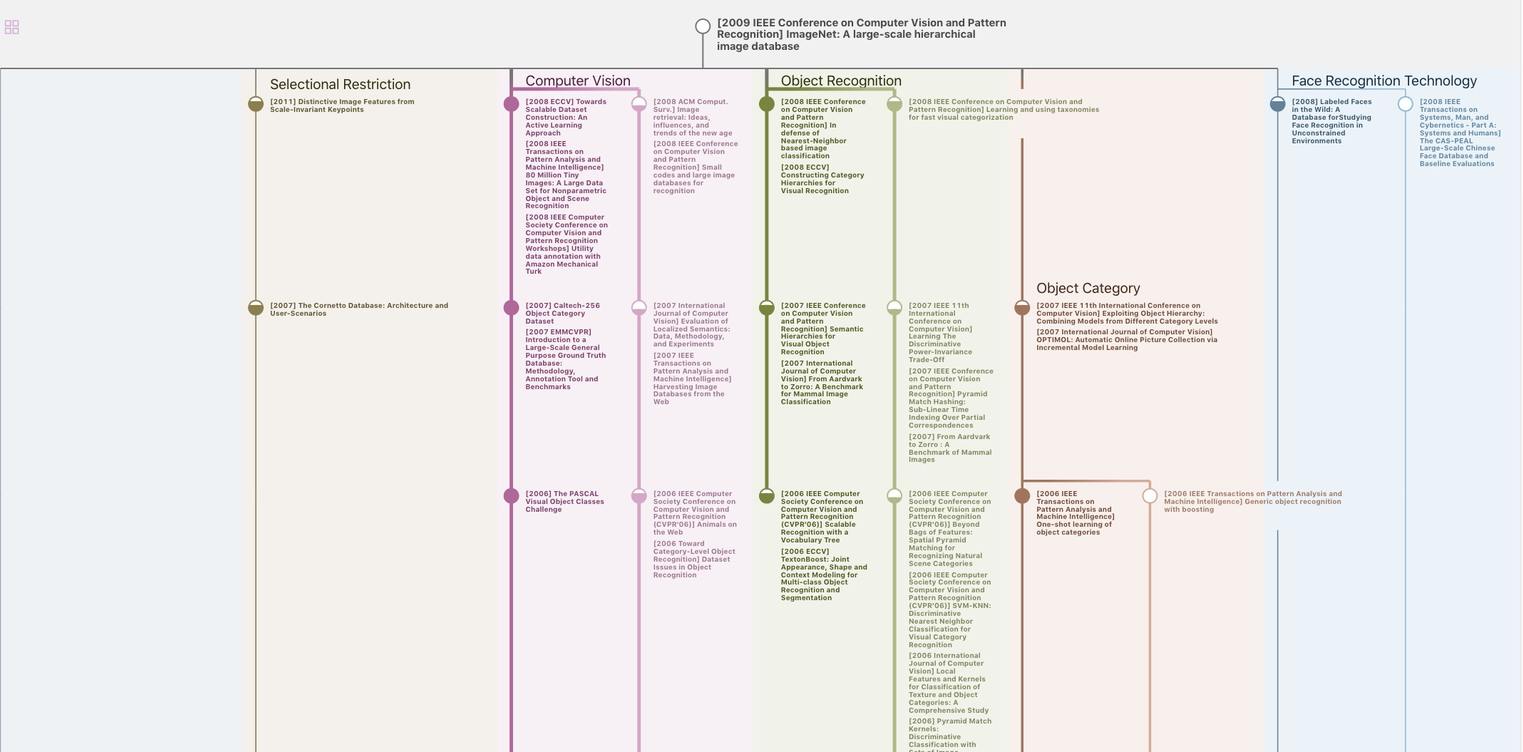
生成溯源树,研究论文发展脉络
Chat Paper
正在生成论文摘要