Memristive Devices for Time Domain Compute-in-Memory
IEEE Journal on Exploratory Solid-State Computational Devices and Circuits(2022)
摘要
Analog compute schemes and compute-in-memory (CIM) have emerged in an effort to reduce the increasing power hunger of convolutional neural networks (CNNs), which exceeds the constraints of edge devices. Memristive device types are a relatively new offering with interesting opportunities for unexplored circuit concepts. In this work, the use of memristive devices in cascaded time-domain CIM (TDCIM) is introduced with the primary goal of reducing the size of fully unrolled architectures. The different effects influencing the determinism in memristive devices are outlined together with reliability concerns. Architectures for binary as well as multibit multiply and accumulate (MAC) cells are presented and evaluated. As more involved circuits offer more accurate compute result, a tradeoff between design effort and accuracy comes into the picture. To further evaluate this tradeoff, the impact of variations on overall compute accuracy is discussed. The presented cells reach an energy/OP of 0.23 fJ at a size of
$1.2~{\mu{ }}\text{m}^{2}$
for binary and 6.04 fJ at
$3.2~\mu \text{m}^{2}$
for
$4\times 4$
bit MAC operations.
更多查看译文
关键词
Compute-in-memory (CIM),convolutional neural networks (CNNs),memristive devices,time-domain (TD) computing,time-domain CIM (TDCIM)
AI 理解论文
溯源树
样例
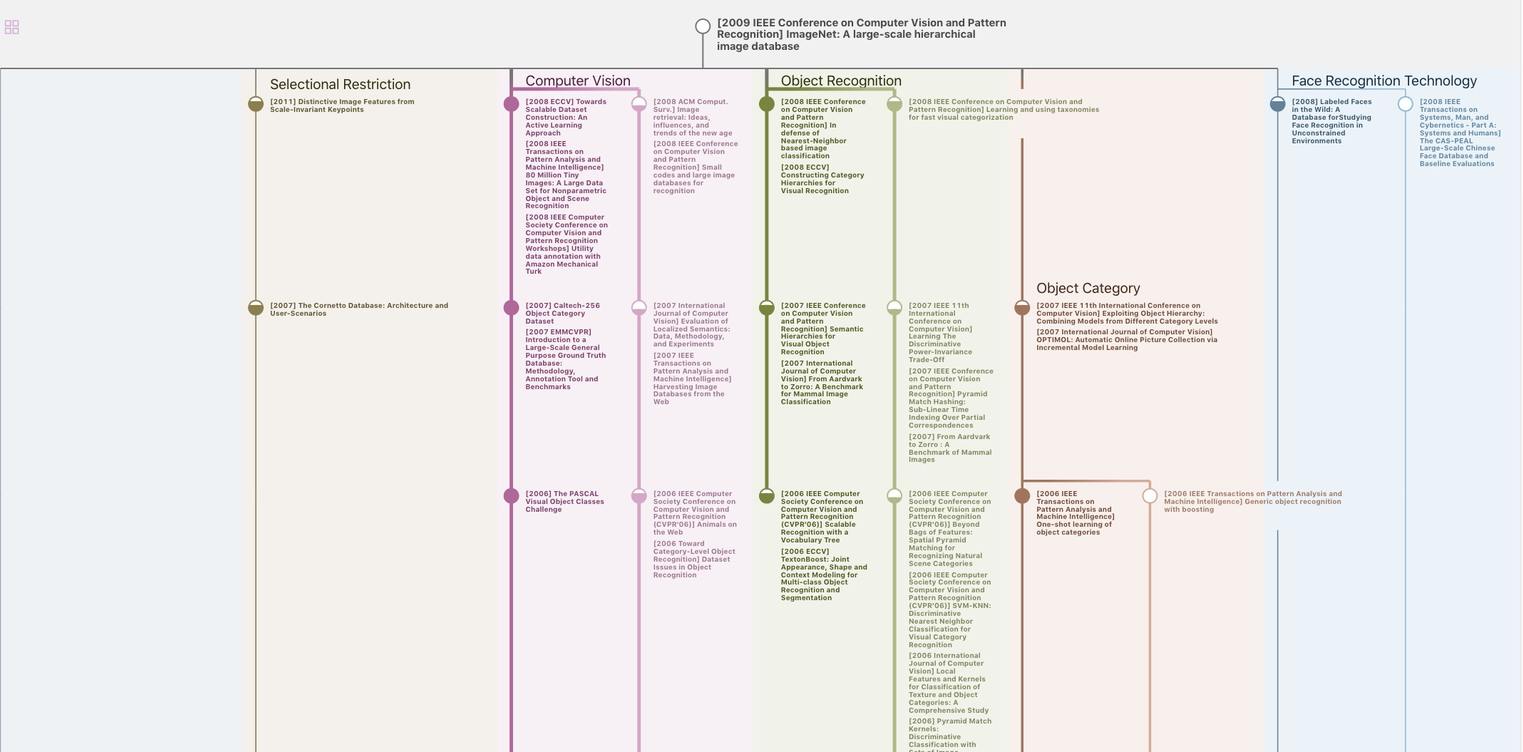
生成溯源树,研究论文发展脉络
Chat Paper
正在生成论文摘要