Deep learning based on byte sample entropy for VPN encrypted traffic identification
2022 5th International Conference on Advanced Electronic Materials, Computers and Software Engineering (AEMCSE)(2022)
摘要
Network traffic identification is important for traffic engineering, resource allocation, network management, attack detection and improving network QoS. However, with the rapid development of computer network technology, various VPN technologies and applications have emerged, which use encryption and decryption technology, tunneling technology and authentication technology to obfuscate and hide traffic characteristics, making VPN traffic difficult to identify. The recent rise of V2Ray makes up for and completes the shortcomings of previous VPN technologies with a more complete protocol, more robust performance and richer functionality, using V2Ray’s customised VMess protocol, and the VMess protocol supports TLS-based implementations, making it a full-featured and powerful application. These undoubtedly pose a huge challenge for network traffic identification and auditing, as well as a huge risk for network security. Therefore, the identification of VPN traffic is of great importance. In this paper, we propose a VPN traffic identification method based on byte sample entropy and session interaction time difference. We use the byte sample entropy and session interaction time difference of some message sequences in network traffic as feature data, and use Random Forest RF (RF) algorithm to identify V2Ray VMess traffic, TLS-based VMess traffic and ISCX VPN-NonVPN public dataset, achieving 95.97%, 90.32% and 91.78% recognition accuracy, respectively. The experimental results show that the method can be used for the detection and identification of V2Ray traffic, and also supports the detection and identification of the rest of VPN traffic.
更多查看译文
关键词
Encrypted raffic identification,VPN traffic,byte sample entropy,random forest algorithm
AI 理解论文
溯源树
样例
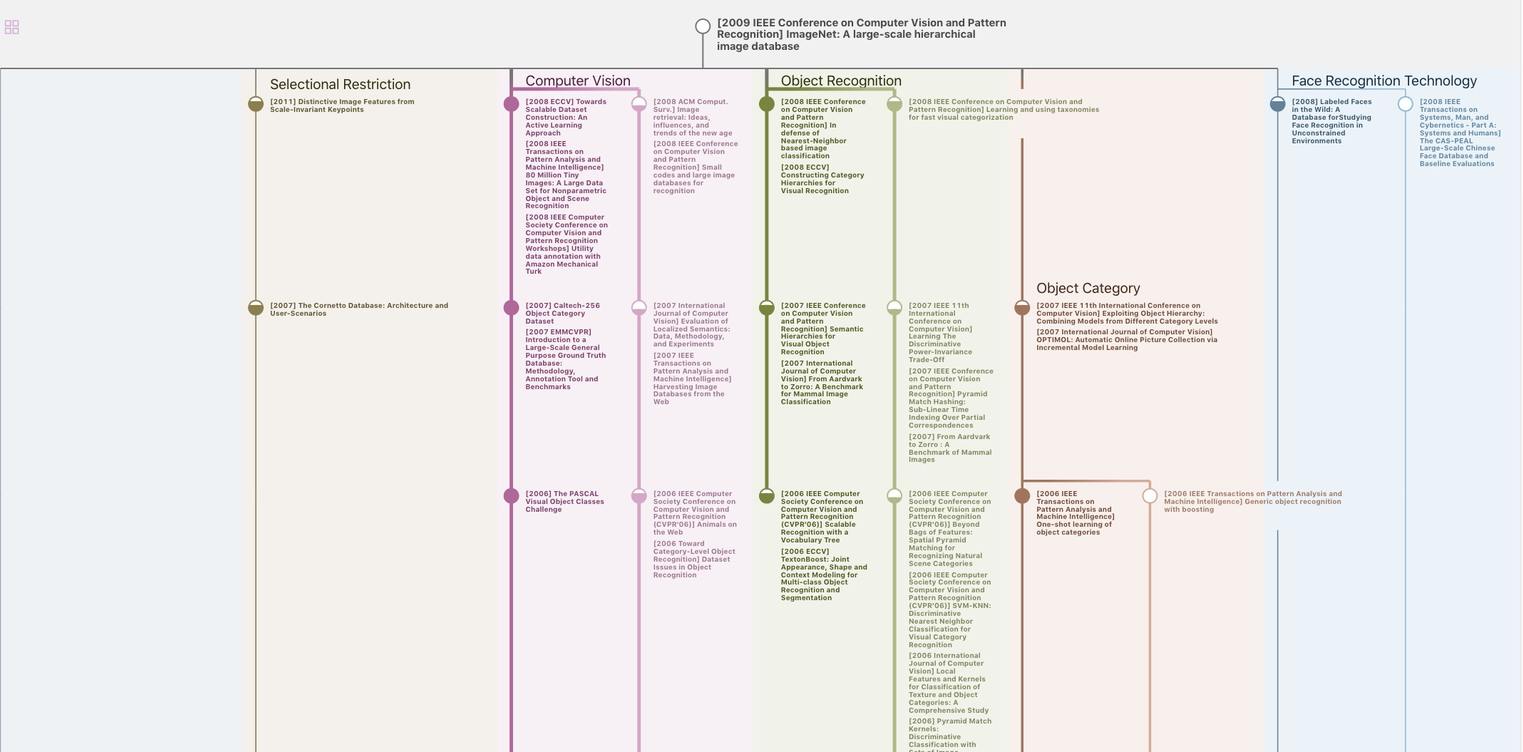
生成溯源树,研究论文发展脉络
Chat Paper
正在生成论文摘要