VMAF based quantization parameter prediction model for low resolution video coding
2022 International Conference on Advanced Technologies for Communications (ATC)(2022)
摘要
Perceptual video quality assessment (VQA) is now essential part of various video applications by controlling the quality for the videos delivered to the users. Recently, Video Multimethod Assessment Fusion (VMAF) is developed by Netflix, known as a full refence VQA model that combines spatial and temporal features to predict perceptual quality. This metric has stronger correlation with human visual system than the conventional metrics such as Peak Signal to Noise Ratio (PSNR) and Structure Similarity Index (SSIM). However, the perceptual video quality is normally degraded due to the content and bitrate variation. This leads to uncomfortable experience for the viewers. In this context, this paper proposes an adaptive quantization parameter (QP) prediction model based on VMAF for video coding to achieve better perceptual quality at a reasonable bitrate. In particular, the Rate Distortion Optimization (RDO) progress of x.264 video codec is improved by using the VMAF scores to find optimal QP map. The results showed that the compression performance of x.264 codec with the proposed method achieved up to 5.95% bitrate saving when compared to the conventional x.264 encoder.
更多查看译文
关键词
Video coding,Neural network,VMAF
AI 理解论文
溯源树
样例
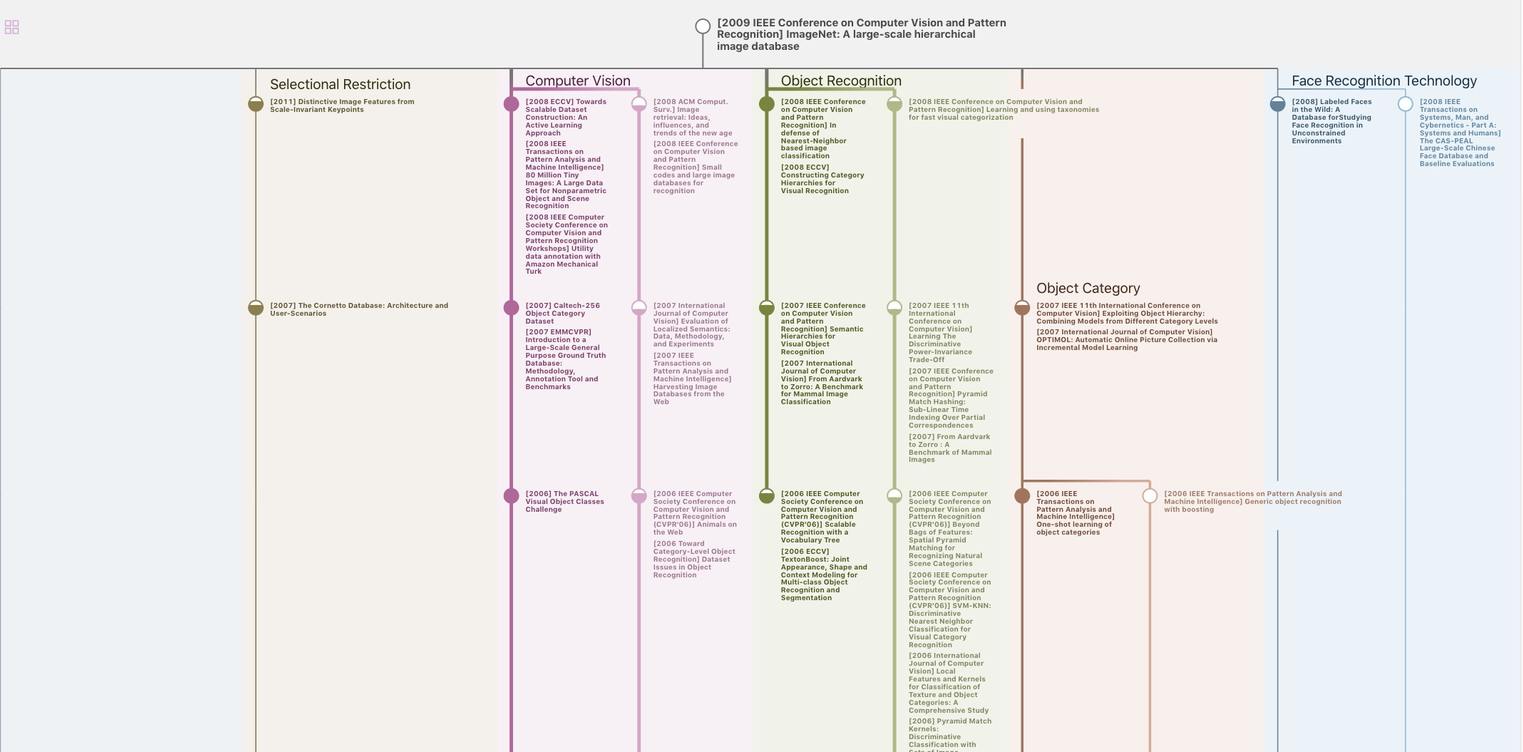
生成溯源树,研究论文发展脉络
Chat Paper
正在生成论文摘要