Comparative Analysis of Machine Learning Techniques for Acoustic Machine Tracking under Different Signal Durations
2022 3rd International Conference on Artificial Intelligence and Data Sciences (AiDAS)(2022)
摘要
The concept of smart factory introduced in Industrial Revolution 4.0 raises demand for purchasing advanced machines among the industry. However, the idea to replace the outdated machines with limited functionality is not viable for small and medium-sized enterprises as those machines are working optimally through decades. Therefore, in order to alleviate low productivity output issue of the old machines, a machine activity tracking system via non-contact acoustic approach is proposed as an alternative option that saves purchasing and implementation costs altogether through simple installation of durable sensor onto the machines. The paper suggests that the alteration of acoustic signal duration potentially affect three critical attributes: tracking accuracy, training time and inference time that determine the effectiveness of the tracking system. Thus, the objective of the paper is to analyze the influence of the signal duration on the key attributes for various machine learning techniques in search of the best suited machine tracking technique. The paper also investigates the limitation of the acoustic tracking approach in terms of training dataset size. The experimental result had shown that with 4 seconds acoustic signal, the logistic regression technique with one-vs-rest scheme and liblinear solver yields the best f1-score of 0.909 and standard deviation of 0.013. The technique also performs significantly fast in terms of training and inference times for recognizing machines' sound. Additionally, most techniques grant f1-score near to 0.8 with 6 minutes training dataset.
更多查看译文
关键词
Internet-of-Things,legacy machine monitoring,operation status tracking,sound recognition
AI 理解论文
溯源树
样例
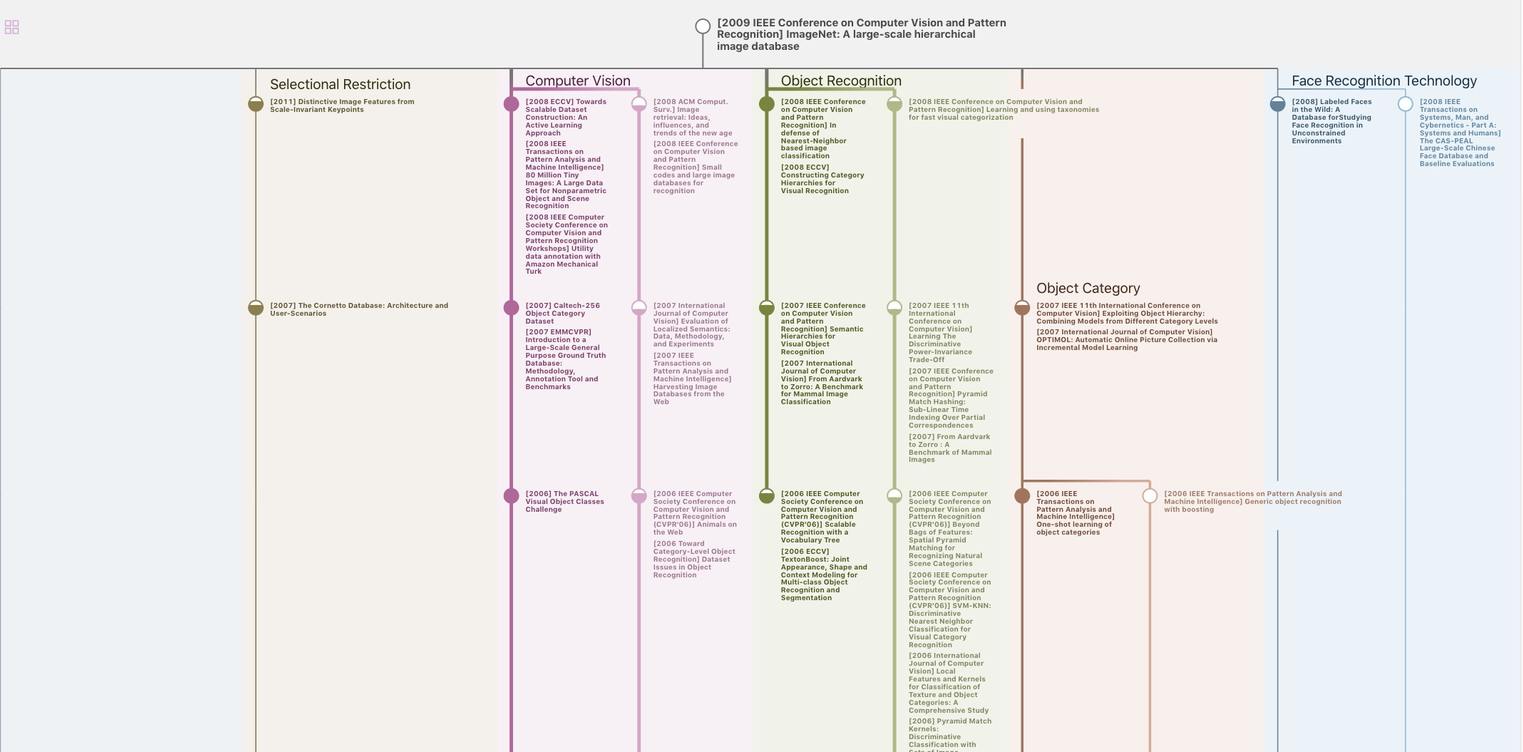
生成溯源树,研究论文发展脉络
Chat Paper
正在生成论文摘要