Adaptive Control of Data Center Cooling using Deep Reinforcement Learning
2022 IEEE International Conference on Autonomic Computing and Self-Organizing Systems Companion (ACSOS-C)(2022)
摘要
In this paper, we explore the use of Reinforcement Learning (RL) to improve the control of cooling equipment in Data Centers (DCs). DCs are inherently complex systems, and thus challenging to model from first principles. Machine learning offers a way to address this by instead training a model to capture the thermal dynamics of a DC. In RL, an agent learns to control a system through trial-and-error. However, for systems such as DCs, an interactive trial-and-error approach is not possible, and instead, a high-fidelity model is needed. In this paper, we develop a DC model using Computational Fluid Dynamics (CFD) based on the Lattice Boltzmann Method (LBM) Bhatnagar-Gross-Krook (BGK) algorithm. The model features transient boundary conditions for simulating the DC room, heat-generating servers, and Computer Room Air Handlers (CRAHs) as well as rejection components outside the server room such as heat exchangers, compressors, and dry coolers. This model is used to train an RL agent to control the cooling equipment. Evaluations show that the RL agent can outperform traditional controllers and also can adapt to changes in the environment, such as equipment breaking down.
更多查看译文
关键词
Reinforcement Learning,CFD Modeling,Data-center Cooling,Adaptive Control
AI 理解论文
溯源树
样例
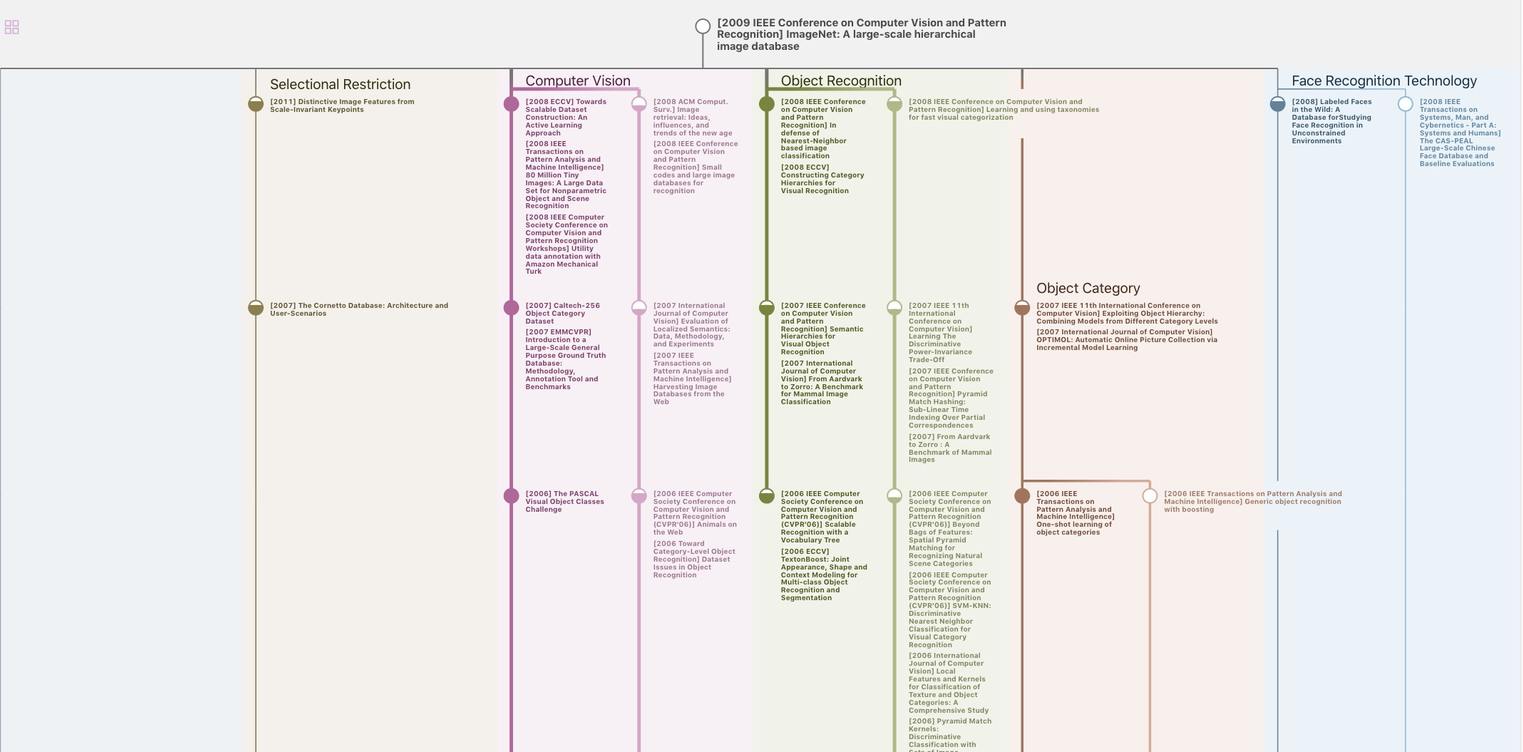
生成溯源树,研究论文发展脉络
Chat Paper
正在生成论文摘要