A hardware-friendly pruning approach by exploiting local statistical pruning and fine grain pruning techniques
2022 IEEE International Conference on Consumer Electronics-Asia (ICCE-Asia)(2022)
摘要
Deep neural networks (DNN) have recently become a popular research topic and achieved well success in many signal processing tasks. However, when we deploy these neural networks on resource-limited hardware; e.g., edge devices, computation complexity and memory capability become great challenges. Many researches devote in compressing model parameters without significant accuracy loss, which is so-call pruning approach, so as to reduce the requirements of computation resource and memory space. However, pruned neural network (NN) model often shows heavy irregular sparsity between layers, convolution kernels, etc. Thus the utilizations of multiply-and-accumulate (MAC) array or processing element (PE) array could be low so that the inference time could not be reduced accordingly. In this paper, we propose a hardware-friendly pruning approach by exploiting local statistical pruning and fine-grain pruning techniques to possibly improve the utilizations of MAC array or PE array. Performance evaluations demonstrate that the performance of a NN-based super-resolution was kept good (i.e., > 37dB) with a high pruning ratio (i.e., > 55%)
更多查看译文
关键词
deep neural network (DNN),model compression,pruning,sparsity,super-resolution
AI 理解论文
溯源树
样例
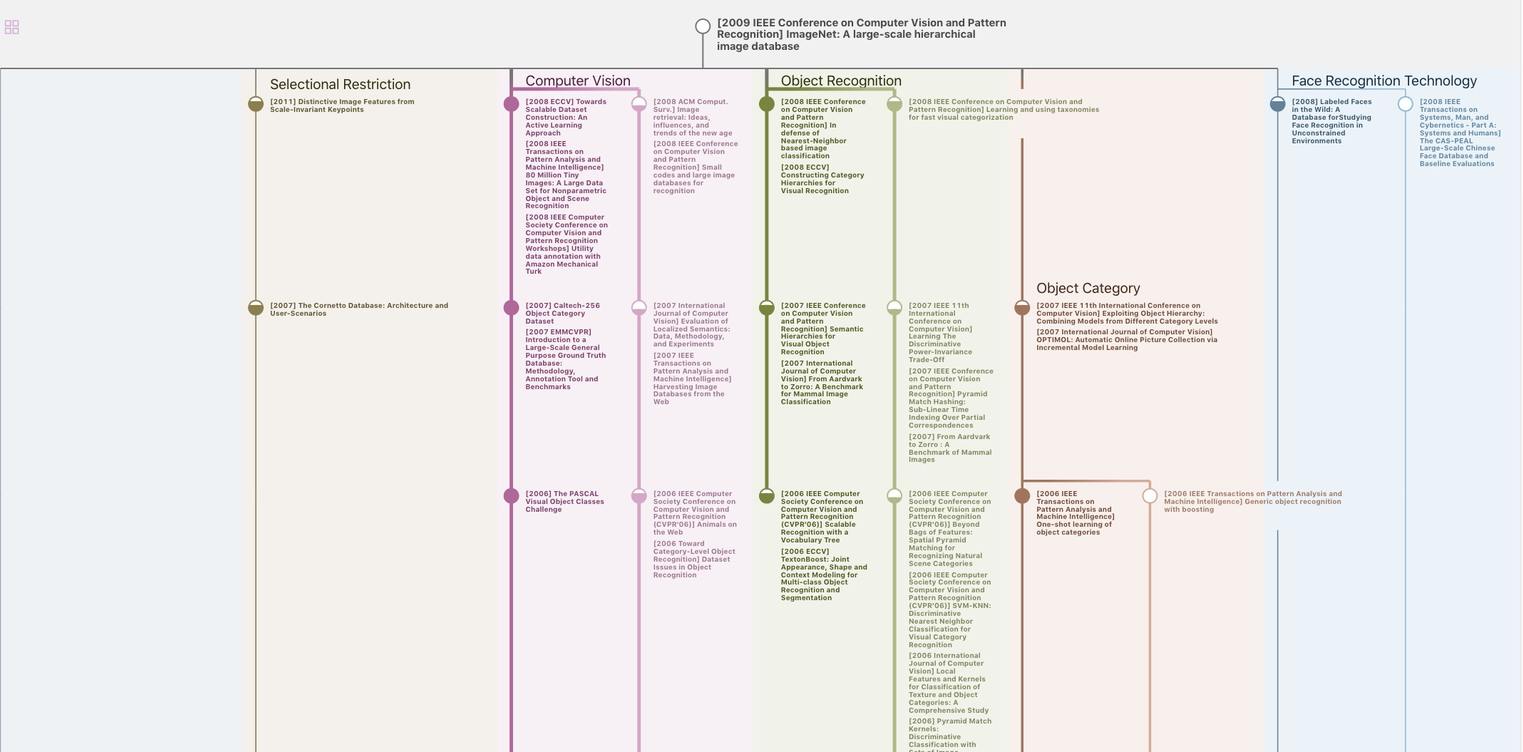
生成溯源树,研究论文发展脉络
Chat Paper
正在生成论文摘要