Classification of High Frequency NILM Transients Based on Convolutional Neural Networks
2022 IEEE Green Energy and Smart System Systems(IGESSC)(2022)
摘要
Smart electric meters require efficient signal processing algorithms for load identification and energy disaggregation. Non-intrusive load monitoring (NILM) systems are able to extract features from the fundamental power signal in order to collect information about the end use of electric loads. Switching transients induced by turning on or off a certain appliance can be used to identify which appliance is connected or disconnected at a given time in the electrical network. The dataset used in this work is the most recent version of the Plug-Load Appliance Identification Dataset (PLAID) which contains records of voltages and currents of different electrical appliances captured at a high sampling frequency (30 kHz). This paper presents a new approach for appliance classification with deep learning techniques by using a finite impulse response (FIR) high pass filter to remove the fundamental signal, then the short time Fourier transform (STFT) is computed for the feature extraction of high frequency start-up transients induced in the fundamental signal. The proposed convolutional neural network architecture yields a classification accuracy of 95.22% and 88.20% for twelve and sixteen different appliances, respectively.
更多查看译文
关键词
Switching transients,FIR Filter,Short Time Fourier transform,Convolutional Neural Networks
AI 理解论文
溯源树
样例
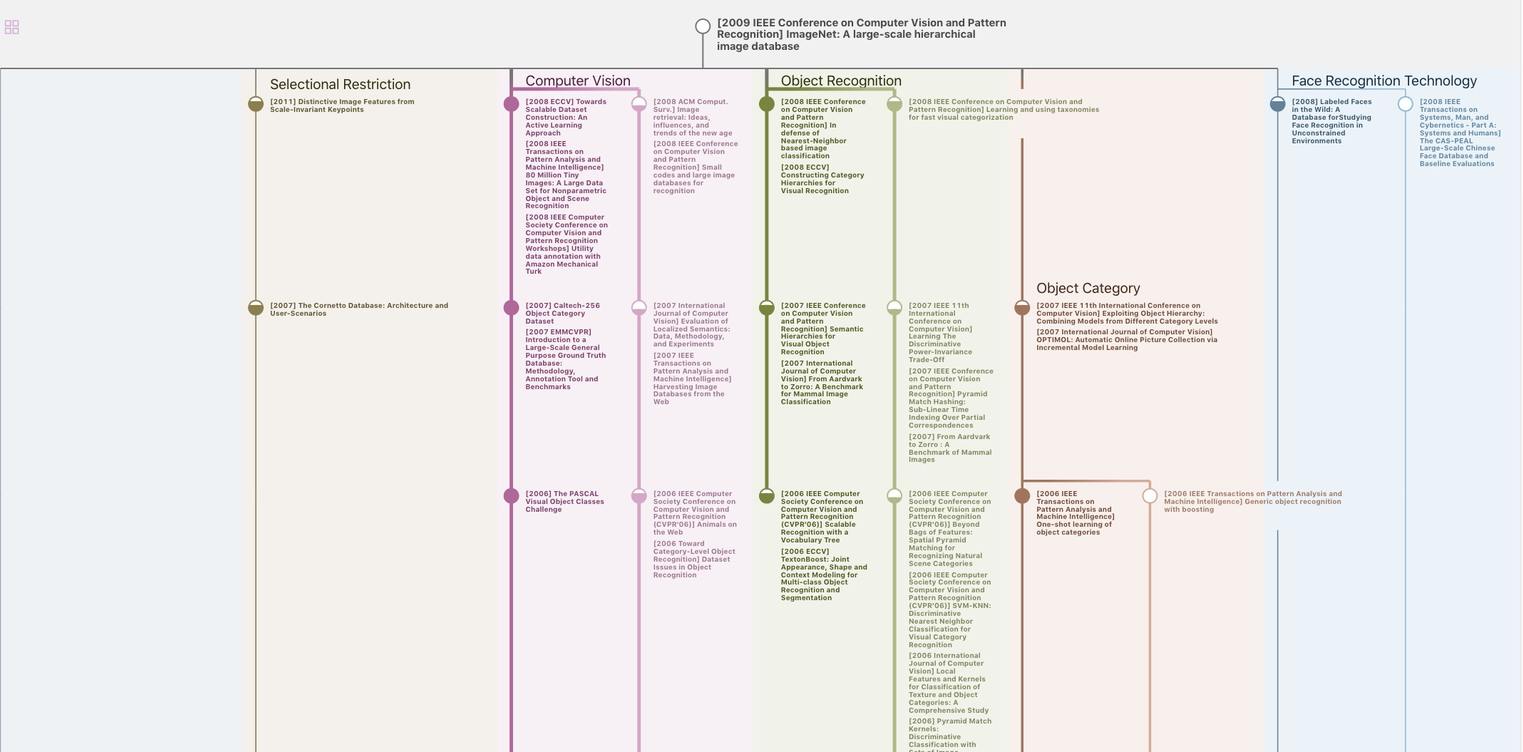
生成溯源树,研究论文发展脉络
Chat Paper
正在生成论文摘要