Clustering-based customer representation learning from dynamic transactional data
2022 IEEE 2nd Conference on Information Technology and Data Science (CITDS)(2022)
摘要
We propose a new clustering-based method for customer feature vector extraction based on the history of their financial transactions. Customer vector representations can be used to solve downstream tasks, such as customer segmentation or next purchase category prediction. The main advantage of the proposed method is that the obtained feature vectors may be interpreted in terms of temporal activity while preserving sufficient quality for solving downstream tasks. Using this method, we were able to extract well-interpreted customer segments (using the debit card transaction data from a large Russian bank) which are useful for various business cases (e.g., planning of marketing campaigns or customized recommendations of financial products). This interpretation would help meet the tasks of analyzing the typical customer behavior and its reasons. In addition, we demonstrate that our method of constructing embeddings provides comparable quality for several downstream tasks (customer purchase category forecasting, missing category prediction, and campaign targeting) with non-interpretable algorithms such as word2vec and autoencoders approaches.
更多查看译文
关键词
client representation learning,transactional data,clustering,customer embeddings,customer feature extraction,customer segmentation
AI 理解论文
溯源树
样例
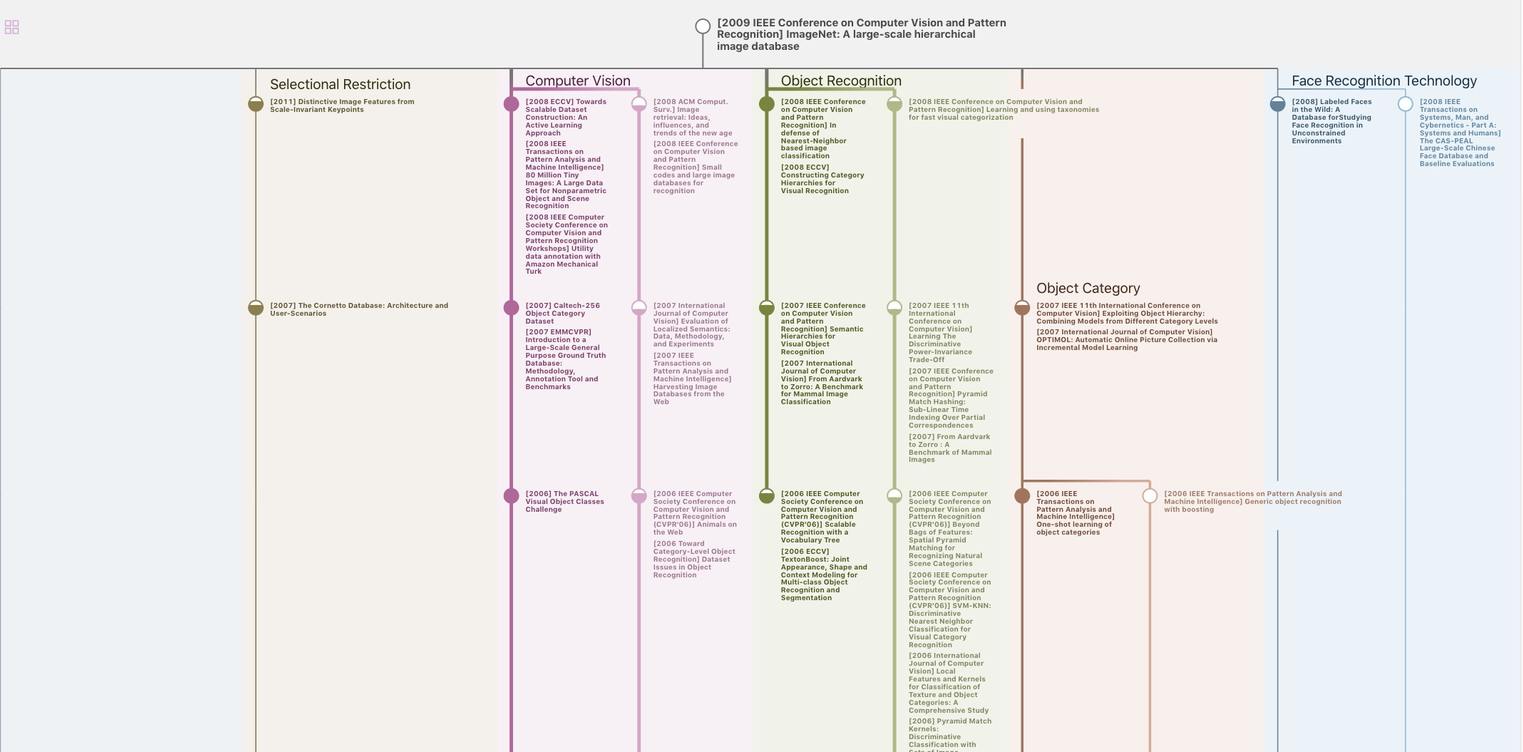
生成溯源树,研究论文发展脉络
Chat Paper
正在生成论文摘要