NLED: Neighbor Linear-Embedding Denoising for Fluorescence Microscopy Images
2022 Medical Technologies Congress (TIPTEKNO)(2022)
摘要
As noise corruption is an inevitable issue for all imaging technologies, this problem causes serious difficulties in analyzing the biological fine-details of fluorescence microscopy images. While Gaussian only, Poisson only and mixture of Poisson- Gaussian can generally be observed, the mixed-noise is more prominent in fluorescence microscopy. In this paper, a novel patch-based denoiser-learning approach is proposed for the images captured by fluorescence microscopy. The developed algorithm mainly builds upon linear-embeddings of neighboring image patches, and it learns a linear transformation between noisy and clean intrinsic geometric properties of patch-spaces. Experimental results demonstrate that the proposed “Neighbor Linear- Embedding Denoising” (NLED) has competitive performance both visually and statistically when compared to other algorithms in literature, for noise corrupted fluorescence microscopy images.
更多查看译文
关键词
Fluorescence microscopy,de noising,neighbor-embedding,linear-embedding
AI 理解论文
溯源树
样例
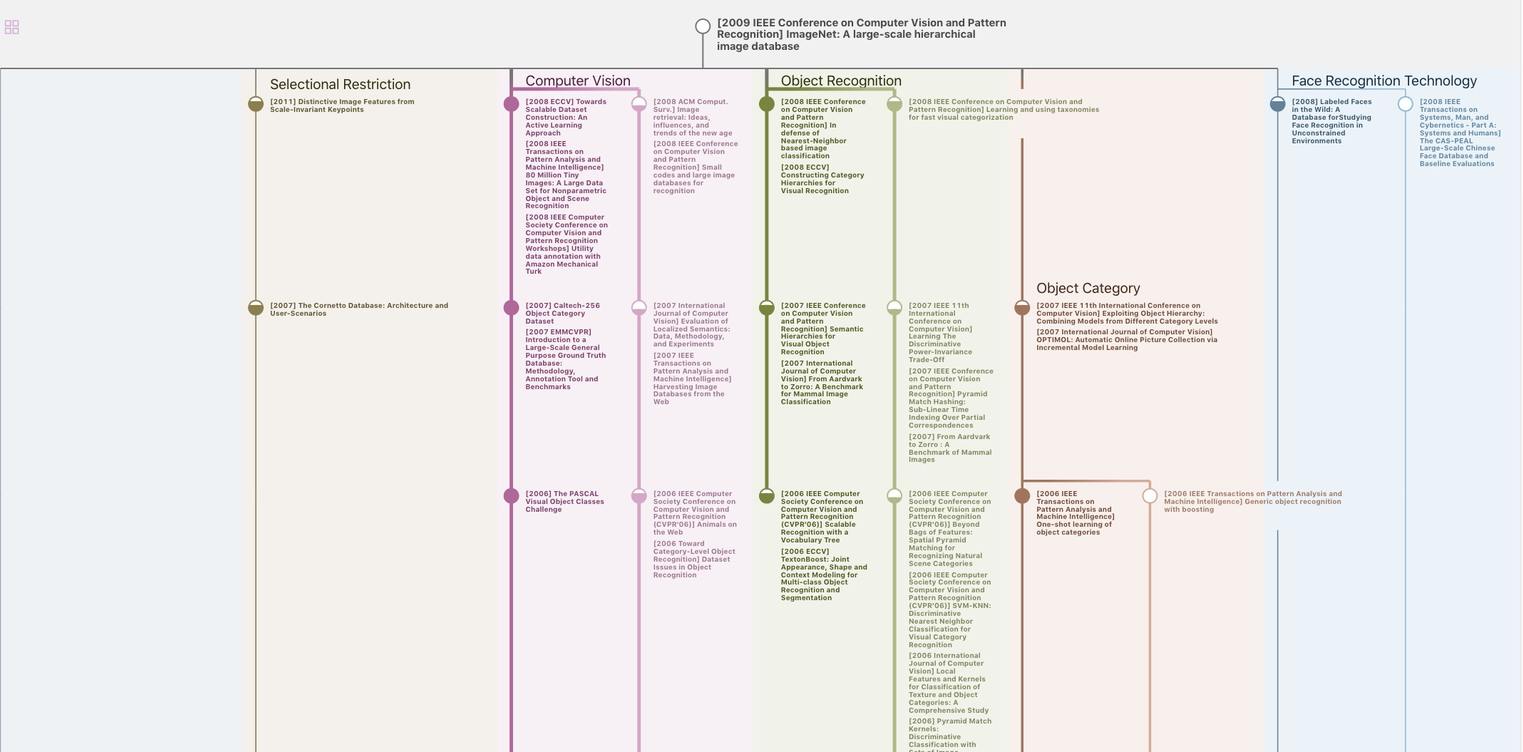
生成溯源树,研究论文发展脉络
Chat Paper
正在生成论文摘要