Bandit Learning-based Online User Clustering and Selection for Cellular Networks
2022 20th International Symposium on Modeling and Optimization in Mobile, Ad hoc, and Wireless Networks (WiOpt)(2022)
摘要
Current wireless networks employ sophisticated multi-user transmission techniques to fully utilize the physical layer resources for data transmission. At the MAC layer, these techniques rely on a semi-static map that translates the channel quality of users to the potential transmission rate (more precisely, a map from the Channel Quality Index to the Modulation and Coding Scheme) for user selection and scheduling decisions. However, such a static map does not adapt to the actual deployment scenario and can lead to large performance losses. Furthermore, adaptively learning this map can be inefficient, particularly when there are a large number of users. In this work, we make this learning efficient by clustering users. Specifically, we develop an online learning approach that jointly clusters users and channel-states, and learns the associated rate regions of each cluster. This approach generates a scenario-specific map that replaces the static map that is currently used in practice. Furthermore, we show that our learning algorithm achieves sub-linear regret when compared to an omniscient genie. Next, we develop a user selection algorithm for multi-user scheduling using the learned user-clusters and associated rate regions. Our algorithms are validated on the WiNGS simulator from AT&T Labs, that implements the PHY/MAC stack and simulates the channel. We show that our algorithm can efficiently learn user clusters and the rate regions associated with the user sets for any observed channel state. Moreover, our simulations show that a deployment-scenario-specific map significantly outperforms the current static map approach for resource allocation at the MAC layer.
更多查看译文
关键词
User clustering,Online learning,User selection,Cellular networks,Scheduling
AI 理解论文
溯源树
样例
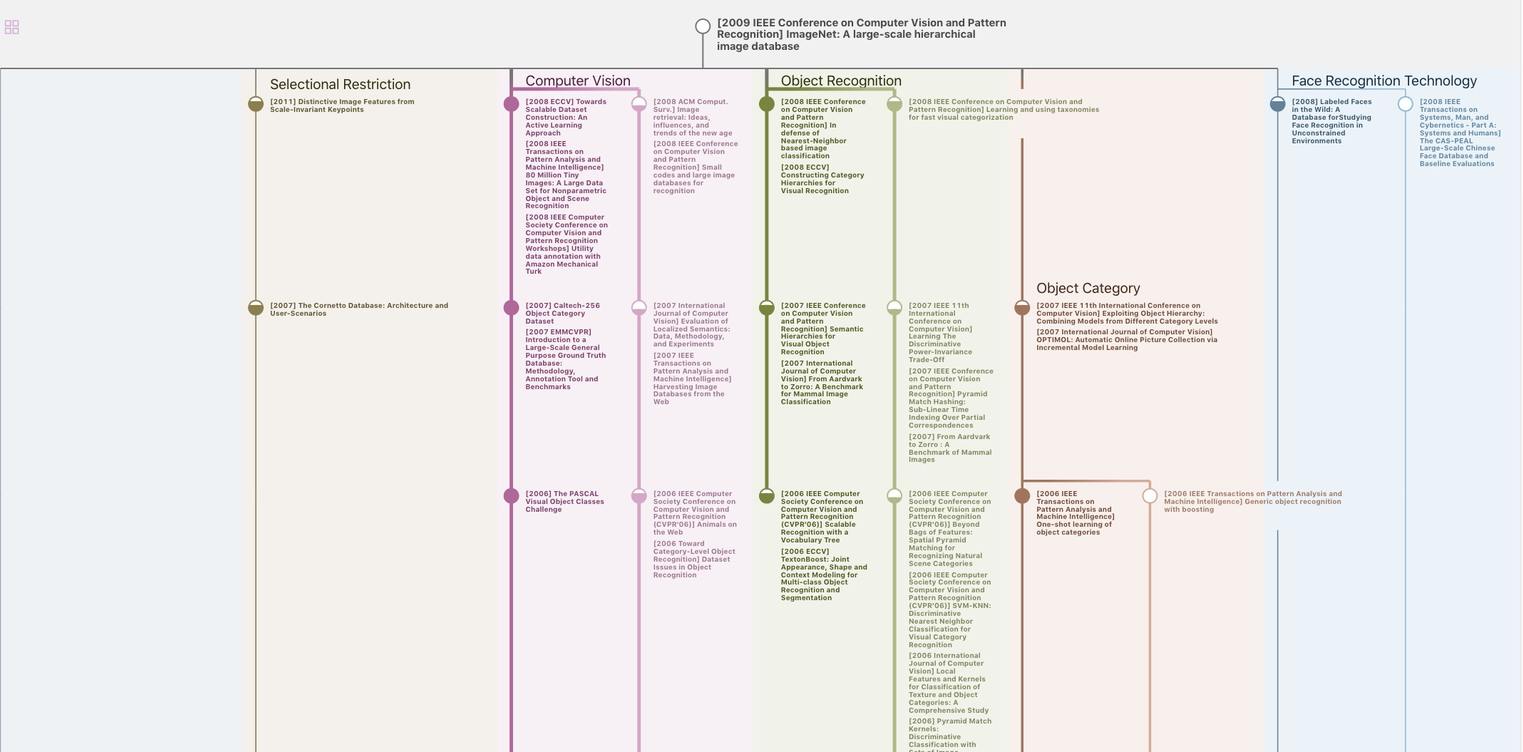
生成溯源树,研究论文发展脉络
Chat Paper
正在生成论文摘要