Measuring Bias and Fairness in Multiclass Classification
2022 IEEE International Conference on Networking, Architecture and Storage (NAS)(2022)
摘要
Algorithmic bias is of increasing concern, both to the research community, and society at large. Bias in AI is more abstract and unintuitive than traditional forms of discrimination and can be more difficult to detect and mitigate. A clear gap exists in the current literature on evaluating the relative bias in the performance of multi-class classifiers. In this work, we propose two simple yet effective metrics, Combined Error Variance (CEV) and Symmetric Distance Error (SDE), to quantitatively evaluate the class-wise bias of two models in comparison to one another. By evaluating the performance of these new metrics and by demonstrating their practical application, we show that they can be used to measure fairness as well as bias. These demonstrations show that our metrics can address specific needs for measuring bias in multi-class classification. Demonstration code is available at https://github.com/gentry-atkinson/CEV_SDE_demo.git.
更多查看译文
关键词
fairness,bias,model quality,model comparison
AI 理解论文
溯源树
样例
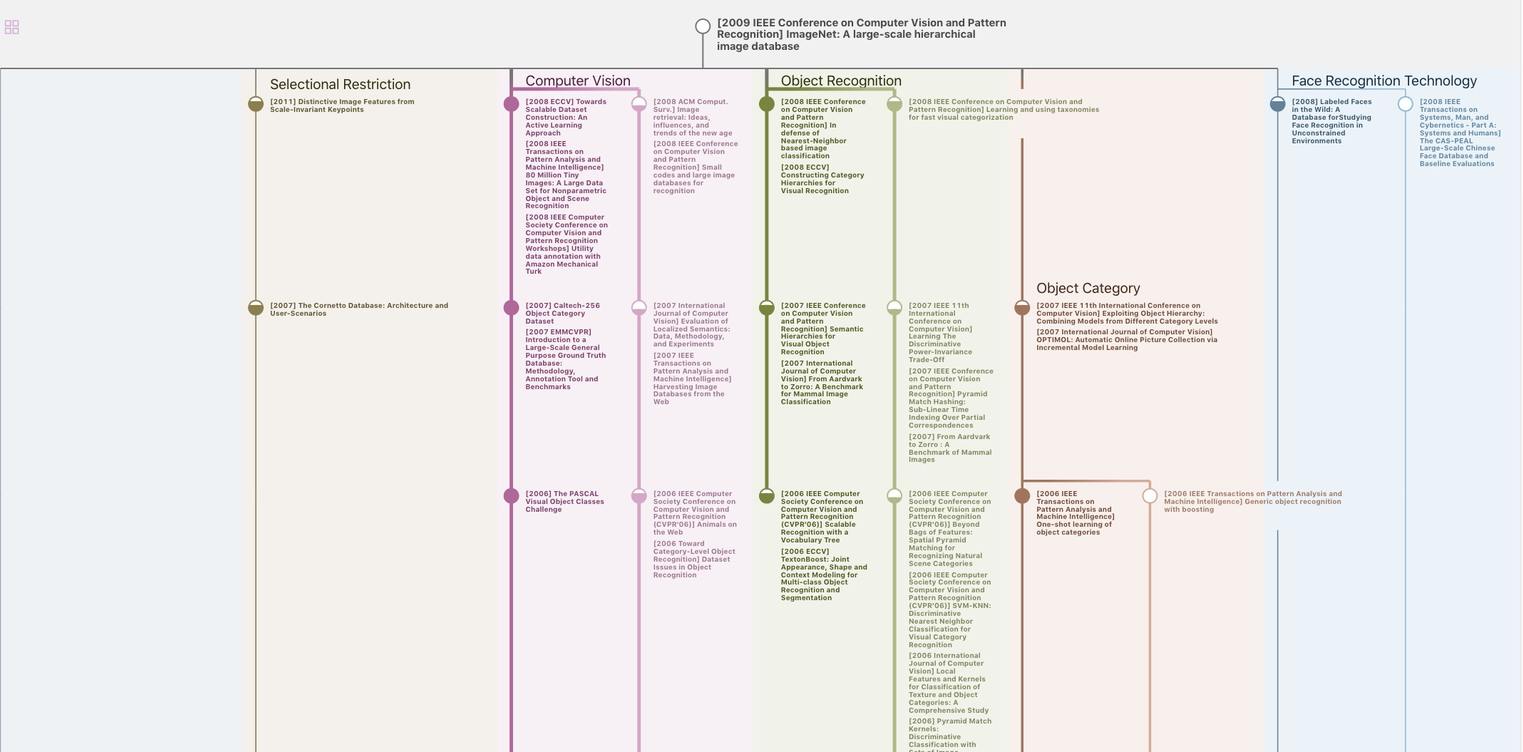
生成溯源树,研究论文发展脉络
Chat Paper
正在生成论文摘要