Optimal binning for a variance based alternative of mutual information in pattern recognition
Neurocomputing(2023)
摘要
Mutual information (MI) is a widely used similarity measure in pattern recognition. MI uses entropy as a measure of uncertainty to quantify the structural similarity of two vectors. Replacing entropy with variance as a measure of uncertainty, an analogous class of similarity measures can be derived and estimated by regression techniques. Recently, the non-linear piecewise constant regression (PWCR) has been proposed to drive similarity measures of this scheme, leading to competitive alternatives of MI. Although PWCR is based on binning, the optimal binning technique for certain problems remained an open question. In this paper, we show mathematically that the optimal binning needs to be aligned with the expected relationship between the vectors being compared. In general, approximately optimal binnings can be found by combinatorial optimization, and in certain cases the optimal binning can be determined by k-means clustering. The theoretical findings are supported by numerical experiments that show a 2–5% increase in the AUC scores in simulated pattern recognition scenarios and improved feature rankings in feature selection problems. The results suggest that the proposed binning techniques could improve the performance of PWCR-driven similarity measures in real-world applications.
更多查看译文
关键词
Dissimilarity,Template matching,Matching by tone mapping,Optimal binning,Explained variance
AI 理解论文
溯源树
样例
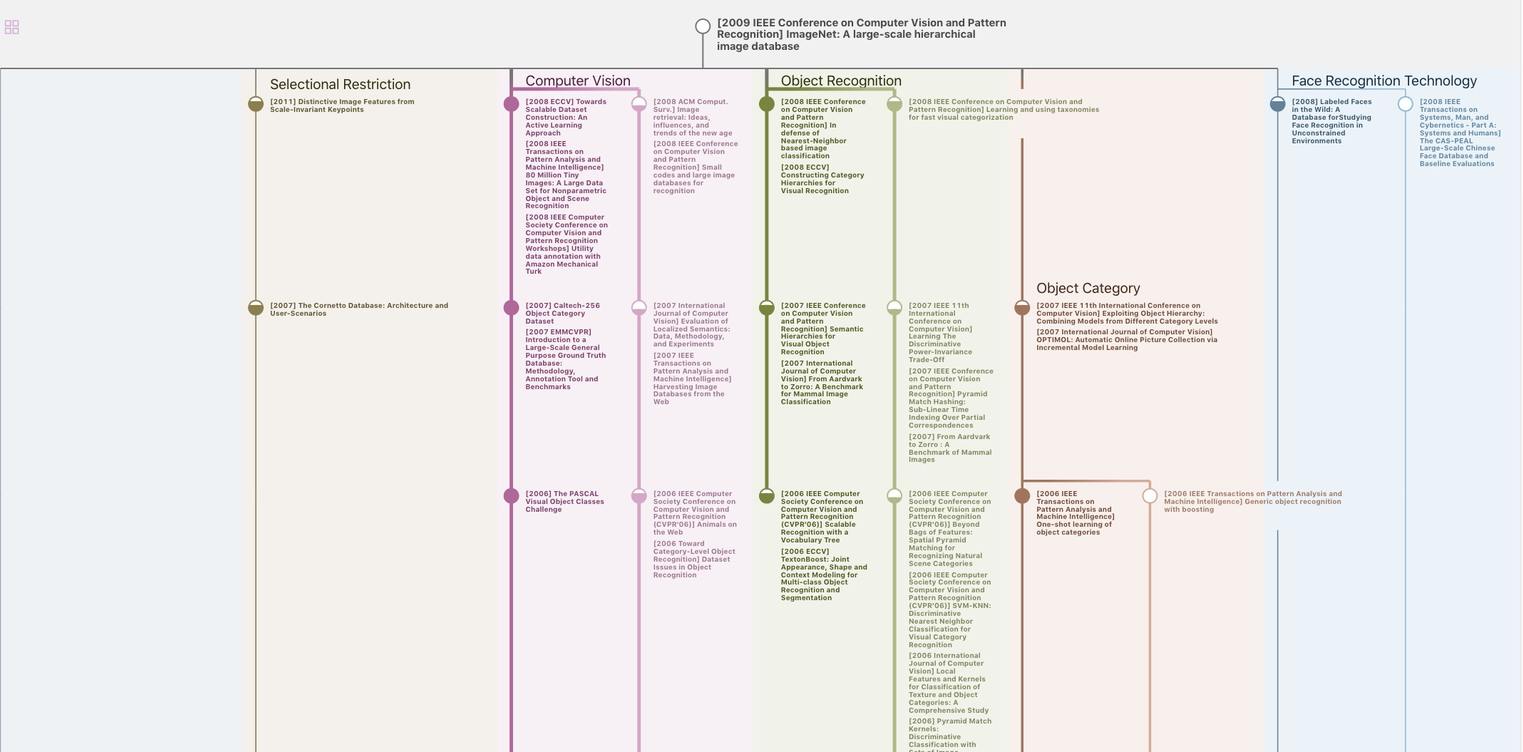
生成溯源树,研究论文发展脉络
Chat Paper
正在生成论文摘要