Attention-Driven Recurrent Imputation for Traffic Speed
IEEE Open Journal of Intelligent Transportation Systems(2022)
摘要
In practice, traffic data collection is often warned by missing data due to communication errors, sensor failures, storage loss, among other factors, leading to impaired data collection and hampering the effectiveness of downstream applications. However, existing imputation approaches focus exclusively on estimating the lost value from incomplete observations and ignore historical data. In this paper, we propose a novel neural network model, namely, Attention-Driven Recurrent Imputation Network (ADRIN), to address the problem of missing traffic data. Specifically, in ADRIN, we devise an Imputation-targeted Long Short-Term Memory (LSTM-I) module for filling in missing data. Meanwhile, we consider the periodicity of historical data and design a historical average calculation module in ADRIN. On this basis, we employ the multi-head self-attention mechanism for further extracting latent temporal features from the output of the two modules. ADRIN is capable of modeling both incomplete observation inputs and historical averages independently to estimate the missing values. We conducted comprehensive experiments on three real-world traffic datasets, to demonstrate that ADRIN consistently outperforms other baselines in a variety of scenarios. Furthermore, ablation experiments are conducted on the various modules of the model, and it is concluded that historical data can significantly enhance the imputation effect.
更多查看译文
关键词
Traffic speed imputation,deep learning,long term short memory,self-attention,intelligent transportation systems
AI 理解论文
溯源树
样例
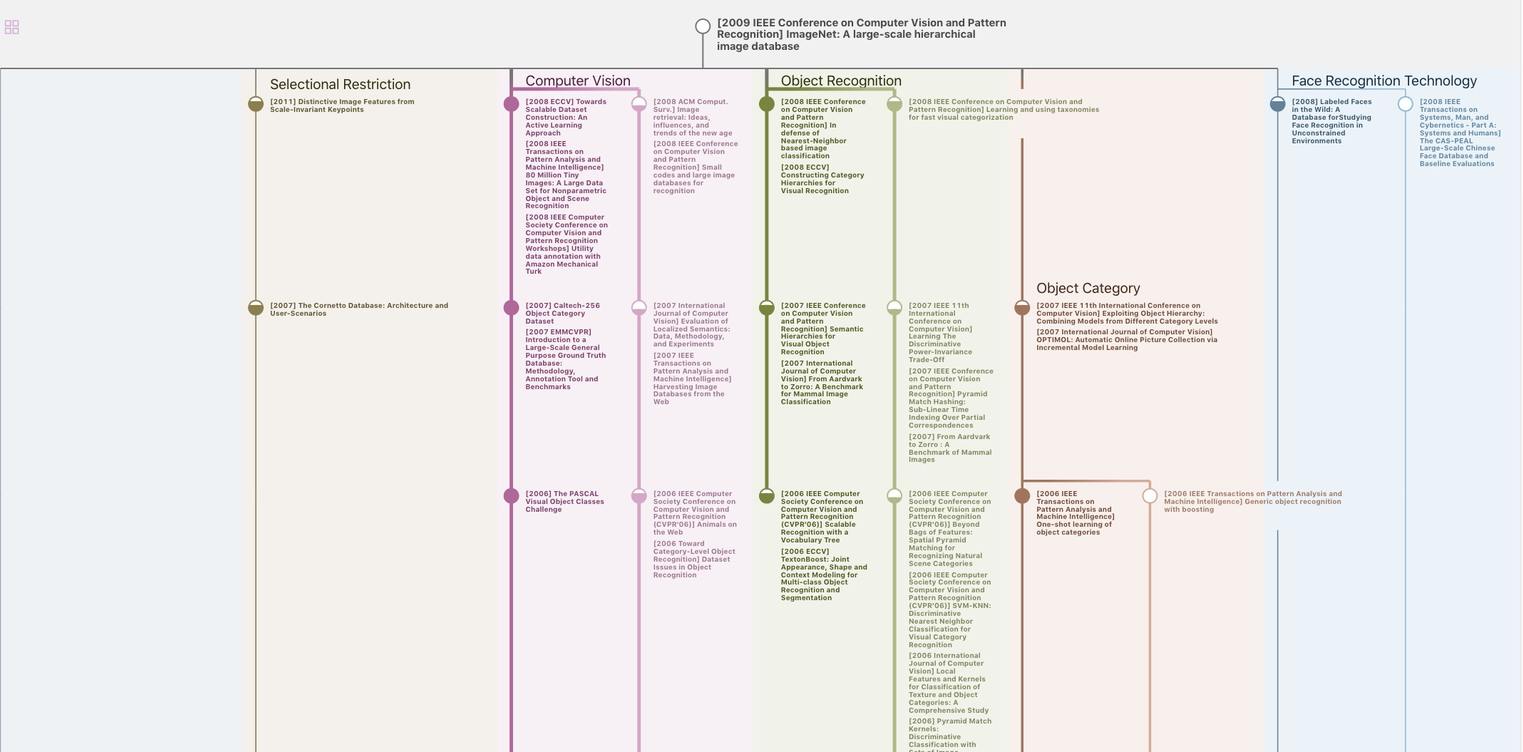
生成溯源树,研究论文发展脉络
Chat Paper
正在生成论文摘要