Biologically plausible unsupervised learning in neural networks with sparse and asymmetric connectivity
biorxiv(2022)
摘要
In the search for biologically plausible but mathematically precise theories of learning in the brain, recent studies have begun to investigate how key assumptions underlying algorithms for supervised learning in artificial neural networks can be relaxed in biologically plausible ways. Turning to unsupervised learning, we develop biologically more plausible variants of the restricted Boltzmann machine (RBM), and benchmark their performance on MNIST. We show that RBMs with asymmetric connectivity can still be successfully trained with contrastive divergence, even if no two units are reciprocally connected. Furthermore, RBMs are able to learn if the forward, visible-to-hidden layer weights are kept constant and only the backward, hidden-to-visible layer weights are updated. These findings indicate that neural networks with biologically plausible connectivity support contrastive learning.
### Competing Interest Statement
The authors have declared no competing interest.
更多查看译文
AI 理解论文
溯源树
样例
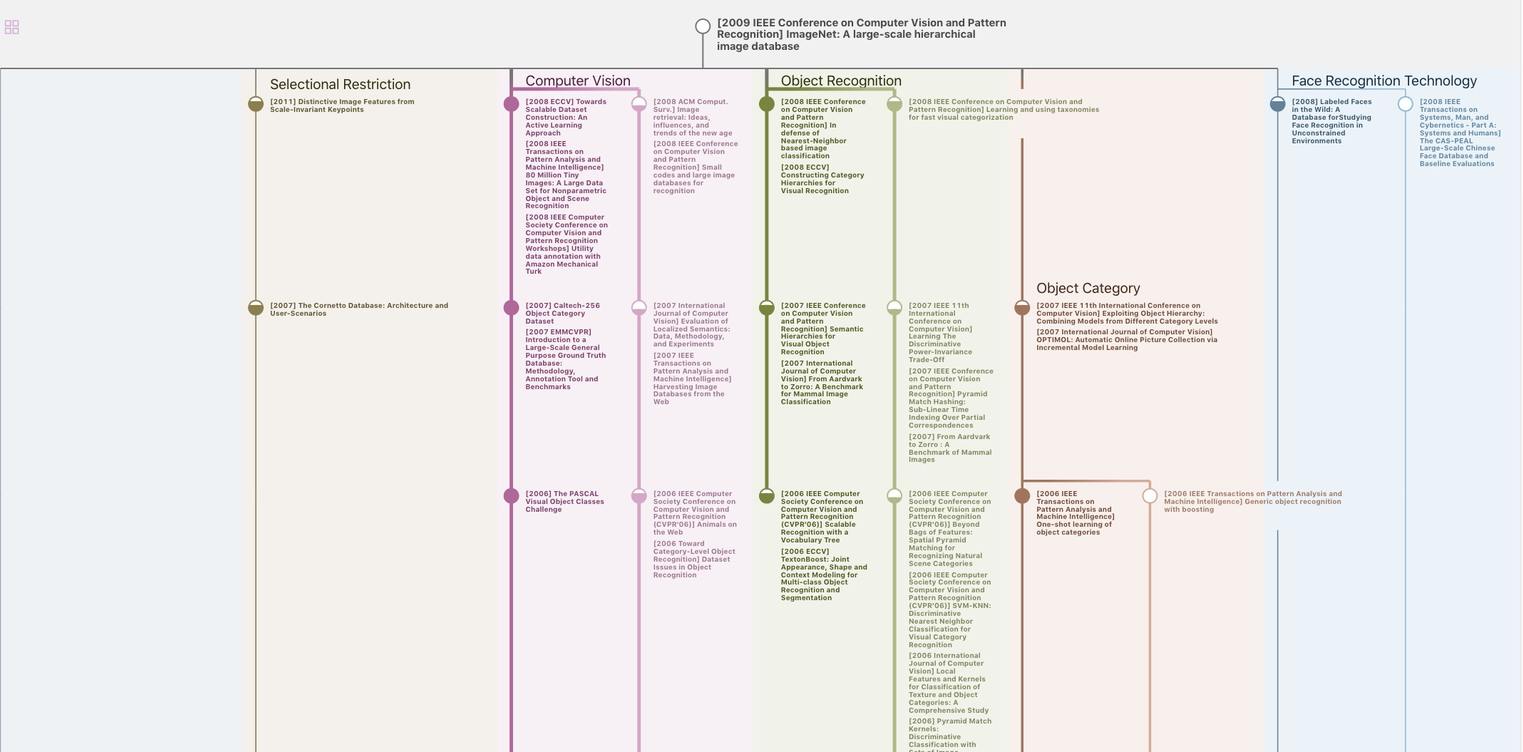
生成溯源树,研究论文发展脉络
Chat Paper
正在生成论文摘要