Estimated Time of Arrival in Autonomous Vehicles Using Gradient Boosting: Real-life case study in public transportation
2022 IEEE International Smart Cities Conference (ISC2)(2022)
摘要
Autonomous Vehicles (AVs) are expected to revolutionise the methods of transportation. Research and innovation in this field is making huge leaps in the last few years, whether it considers vehicles used for private or public transport. Predicting the Estimated Time of Arrival (ETA) is a very important attribute associated with Public Transport (PT). Especially with the rise of AVs' adoption, PT is expected to follow this trend. Therefore, ETA prediction is deemed to be a service that interests the majority of PT stakeholders. PT is a field that automation benefits both stakeholders and commuters, and this research aims to provide a benchmark considering AVs in PT. Within this work, Gradient Boosting (GB) techniques for ETA prediction were employed, namely eXtreme Gradient Boosting (XGBoost), Categorical Boosting (CatBoost) and Light Gradient Boosting Machines (LightGBM). This study proposes competitive ETA prediction methods in Autonomous Buses, while the results of this research are very encouraging and aim to contribute to the overall investigations in the field of autonomous and automated PT.
更多查看译文
关键词
Estimated Time of Arrival,prediction,XGBoost,CatBoost,LightGBM,regression,CCAM
AI 理解论文
溯源树
样例
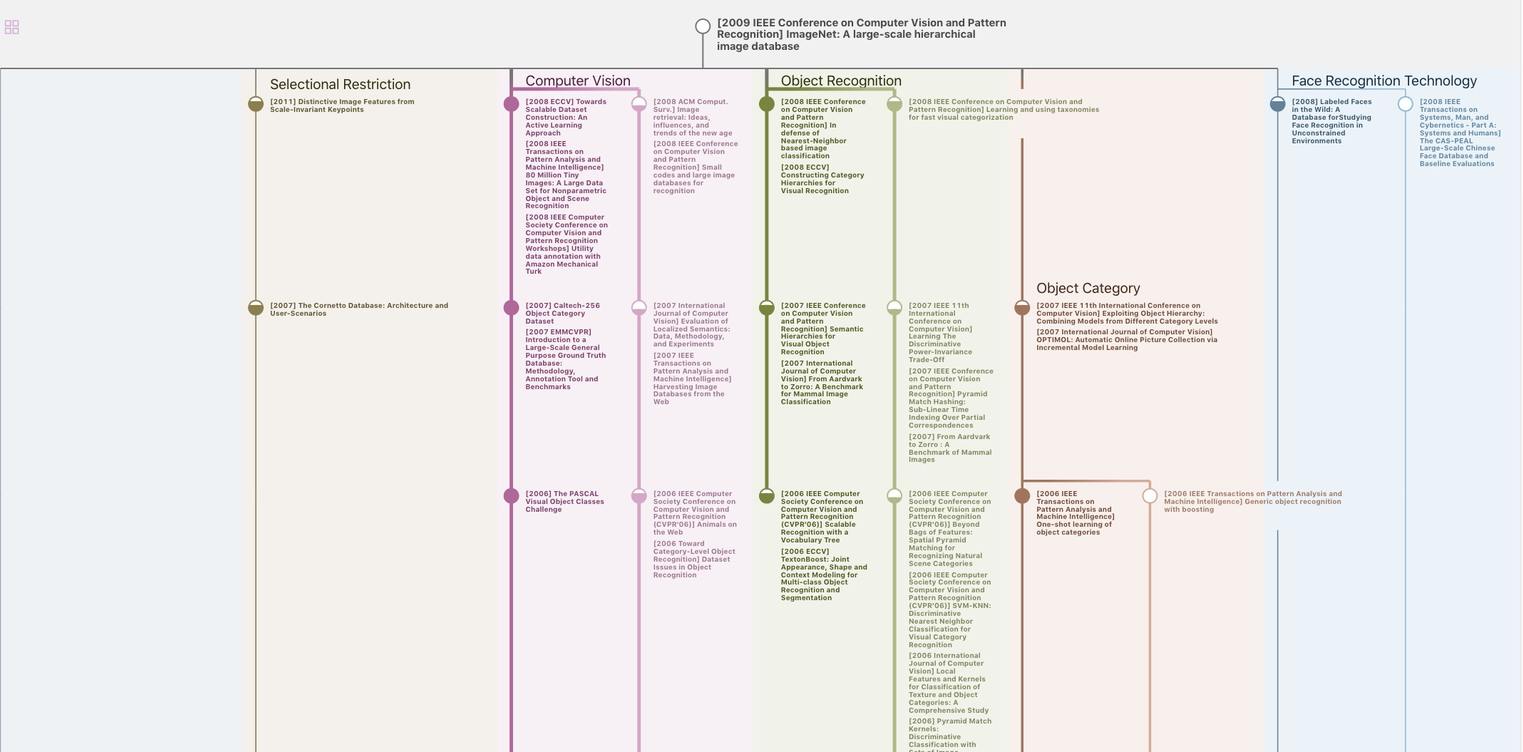
生成溯源树,研究论文发展脉络
Chat Paper
正在生成论文摘要