Comparison of Machine Learning Models for Predicting Indoor Materials from Channel Impulse Response
2022 International Conference on Software, Telecommunications and Computer Networks (SoftCOM)(2022)
摘要
Integrated sensing and communication in future networks will enable enhanced indoor awareness which will offer new possibilities for smart city environments. The use of machine learning (ML) approaches for processing the reflections of the propagating waves in the emerging wireless networks, to yield knowledge about the materials of the surfaces bounding the indoor environment, is a possible research direction. In this work, we formalized the problem as a ML task, i.e. multi-target classification task, and we decomposed it to multiple single-target tasks. We focused on comparison of the optimized performances of size-specific and general models, learned with Nearest Neigh-bors, Multi-Layer Perceptron, Decision Tree, and Random Forest classifiers, on channel impulse response (CIR) data of traced rays on radio links in empty rooms. The results have shown that the performances of the models build for different surfaces and room sizes vary, indicating that the materials of all surfaces should be predicted simultaneously, with single model, based on data from radio links that are placed relatively to the room size.
更多查看译文
关键词
channel impulse response (CIR),hyperparameter tuning,indoor characterization,joint communication and sensing,Machine Learning (ML),ray-tracing
AI 理解论文
溯源树
样例
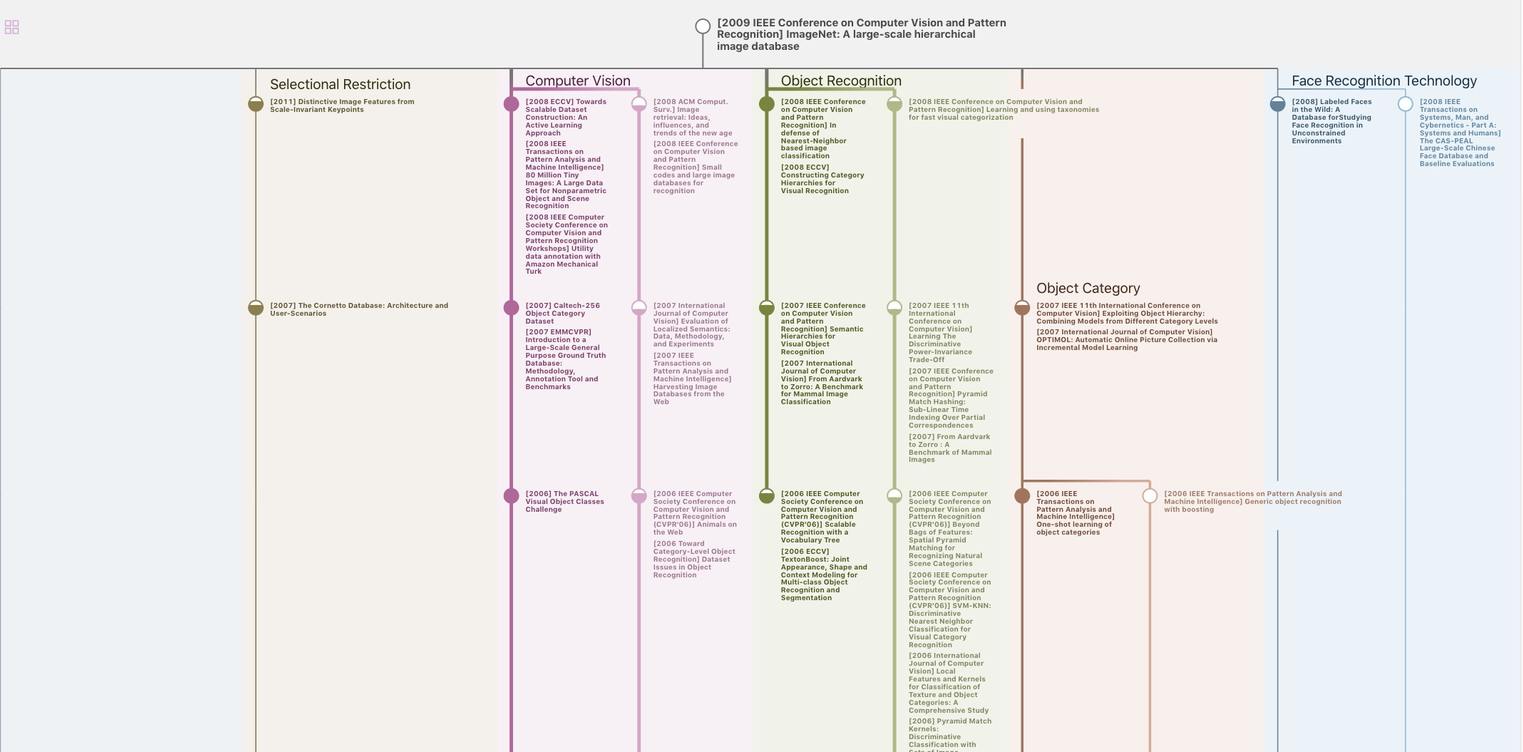
生成溯源树,研究论文发展脉络
Chat Paper
正在生成论文摘要