Efficient tuning of $k$ NN hyperparameters for indoor positioning with N-TBEA
2022 14th International Congress on Ultra Modern Telecommunications and Control Systems and Workshops (ICUMT)(2022)
摘要
Machine Learning is a very popular approach for indoor positioning. However, most of models rely on a set of hyperparameters, which need to be properly set. When the number of hyperparameters is large, exploring all the combinations of values (what is known as brute force) can be computationally prohibitive, especially in those cases where the training or operational time is high, such as in the kNN algorithm in fingerprint-based indoor positioning. This paper introduces
$\boldsymbol{N}$
-Tuple Bandit Evolutionary Algorithm (N-TBEA) to find the hyperparameters in this last case. N-TBEA is an efficient exploration technique which evaluates the feasibility of similar combinations of parameters. The results show that N-TBEA can provide a solution with an accuracy similar to the best combination of parameters retrieved using brute force, which shows the potential of N-TBEA to be used in other advanced machine learning models.
更多查看译文
关键词
Indoor positioning,Machine learning,Optimum parameter selection
AI 理解论文
溯源树
样例
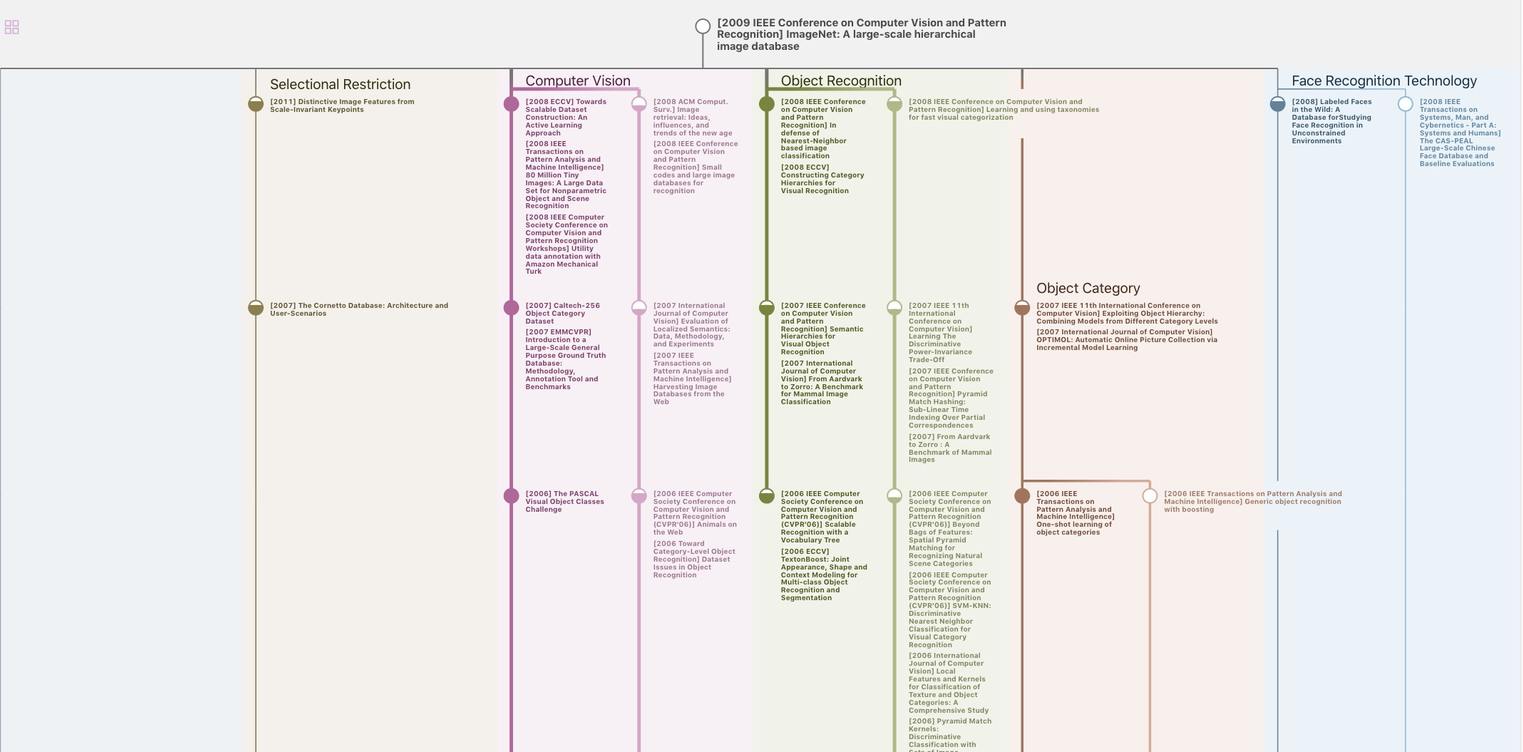
生成溯源树,研究论文发展脉络
Chat Paper
正在生成论文摘要