Computing Particle Size Distribution of Mineral Rocks using Deep Learning-based Instance Segmentation
2022 10th European Workshop on Visual Information Processing (EUVIP)(2022)
摘要
This work presents a deep learning based system for estimating the particle size distribution of two types of rocks in a flow-through environment in mineral processing. Deep Learning has become one of the most important topics in Computer Vision, however, less is known about applying deep neural networks in industrial use cases, where data availability is very limited. Due to this limitation, previous works have focused the efforts on generating synthetic images and benchmark against them or against similar datasets. Because slurry environments exhibit a high level of complexity and image noises, it is almost impossible to transfer knowledge from synthetic data, hence our efforts are aimed towards working only with real data. Target images contain apatite and phlogopite particles in slurry, presenting complex scenarios like overlapping particles, blurry or mixed particles. The proposed system segments all instances of particles, then computes a size distribution based on the predicted masks and the magnification of the imaging device. Finally, the result is benchmarked against a reference method, that uses laser diffraction. Two state-of-the-art neural networks are compared, highlighting tradeoffs that need to be considered for a practical implementation of the system.
更多查看译文
关键词
synthetic images,slurry environments,image noises,synthetic data,target images,overlapping particles,mixed particles,imaging device,state-of-the-art neural networks,particle size distribution,mineral rocks,deep learning-based instance segmentation,deep learning based system,flow-through environment,mineral processing,computer vision,deep neural networks,industrial use cases,data availability
AI 理解论文
溯源树
样例
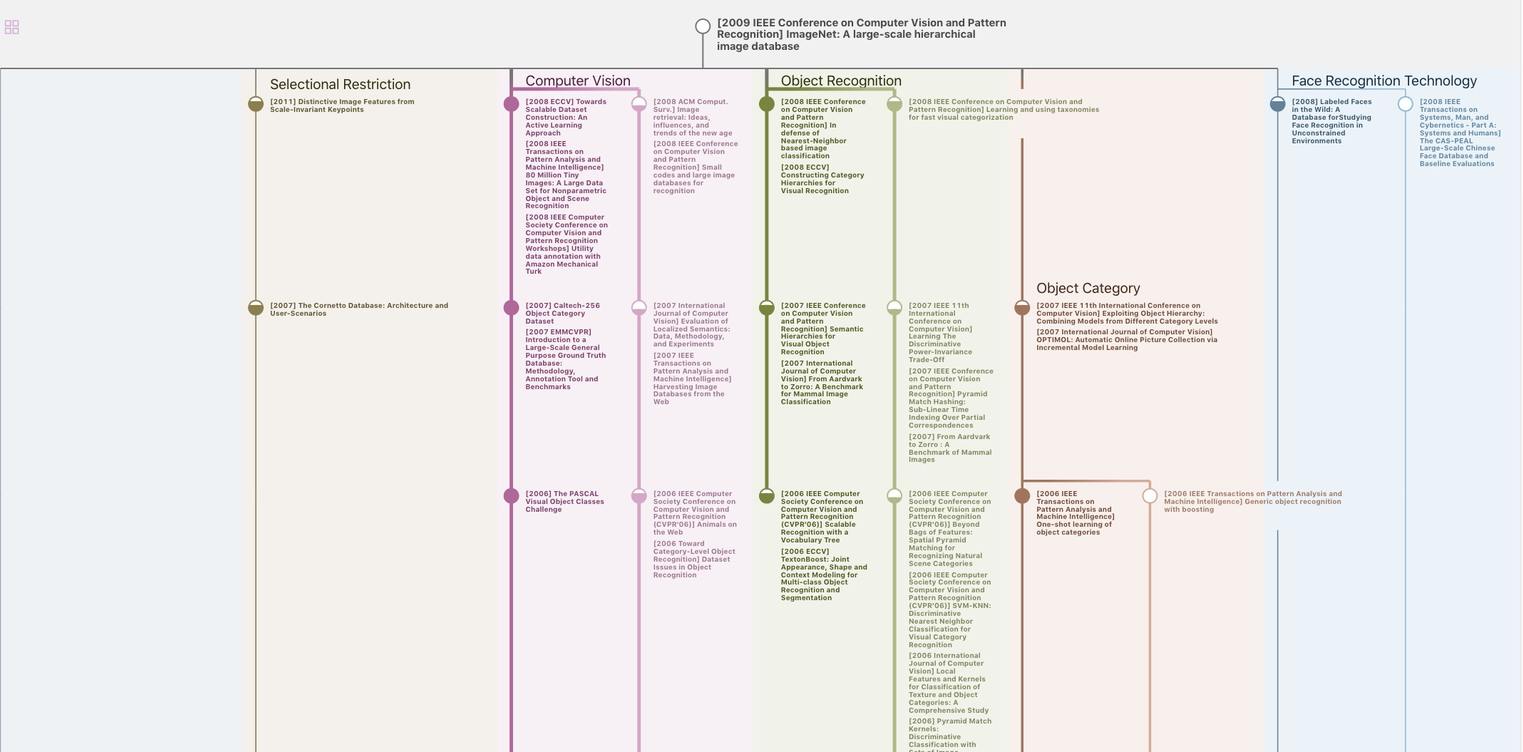
生成溯源树,研究论文发展脉络
Chat Paper
正在生成论文摘要