An Accurate Line-of-Sight Rate Estimation Method Based on LSTM Recurrent Neural Network for Strapdown Imaging Seeker
IEEE Sensors Journal(2022)
摘要
Obtaining accurate line-of-sight (LOS) rates is a challenging task for strapdown imaging seekers. The traditional Kalman filter and its improved algorithm are difficult to deal with the non-Gaussian noise. To this end, we design a model-driven and data-driven filter using long short-term memory (LSTM) recurrent neural network. A decoupling algorithm is proposed to isolate LOS information from the attitude angle and generate one-step predictions. Predictions and observations are used as input for supervised learning, which solves the problem of difficult to describe noise features. Finally, we design a simulation experiment to compare the proposed LSTM model with extended Kalman filter (EKF), unscented Kalman filtering (UKF) and particle filter (PF) on the task of estimating the LOS angle and LOS rate. The experimental results show that the proposed LSTM model is better than the other three methods in terms of accuracy.
更多查看译文
关键词
Kalman filter (KF),line-of-sight (LOS) rate,long short-term memory (LSTM) recurrent neural network (RNN),strapdown imaging seeker
AI 理解论文
溯源树
样例
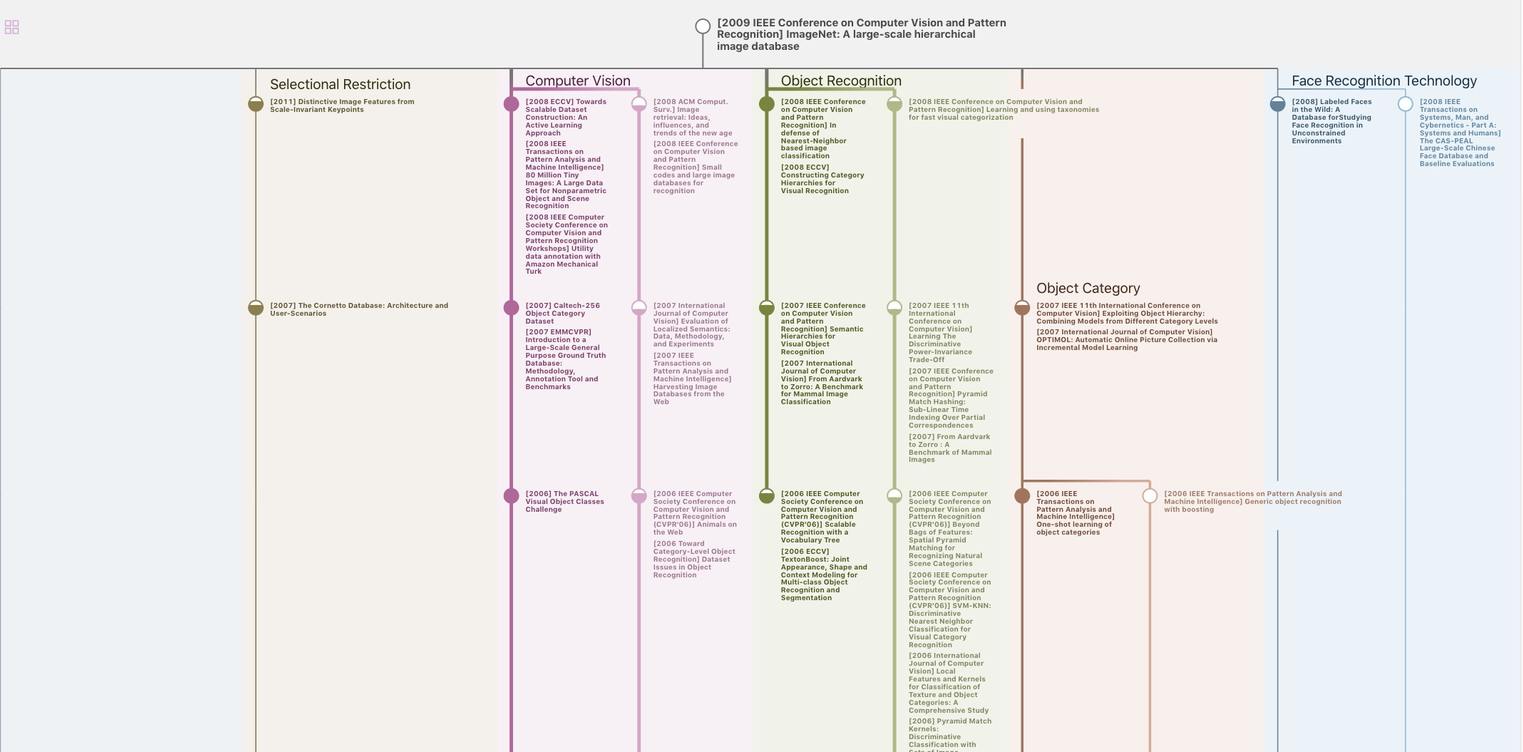
生成溯源树,研究论文发展脉络
Chat Paper
正在生成论文摘要