Reinforcement Learning Based Modulation for Balancing Capacitor Voltage and Thermal Stress to Enhance Current Capability of MMCs
2022 IEEE 13th International Symposium on Power Electronics for Distributed Generation Systems (PEDG)(2022)
摘要
Balancing DC capacitor voltage of many submodules (SMs) is one of the important issues in modular multilevel converter (MMC) systems. In addition, the balance of thermal stress between SMs should be considered to equalize the lifetime expectation of semiconductors and to enhance the current capability of MMC systems. However, it is complicated to balance all the various factors satisfactorily at the same time. Recent machine learning (ML) techniques can achieve optimal results through learning using numerous data acquired in complex environments. Therefore, this paper proposes a new modulation based on reinforcement learning (RL), which is a subclass of ML methods, to optimally balance the capacitor voltage and thermal stress of SMs. A deep Q-network (DQN) agent, which is one of the RL algorithms, is applied in accordance with a nearest-level modulation (NLM), and main features of the DQN agent are described in this paper. The effectiveness of the proposed modulation based on RL is verified by simulations results.
更多查看译文
关键词
Modular multilevel converter,submodule DC capacitor voltage,thermal balancing,reinforcement
AI 理解论文
溯源树
样例
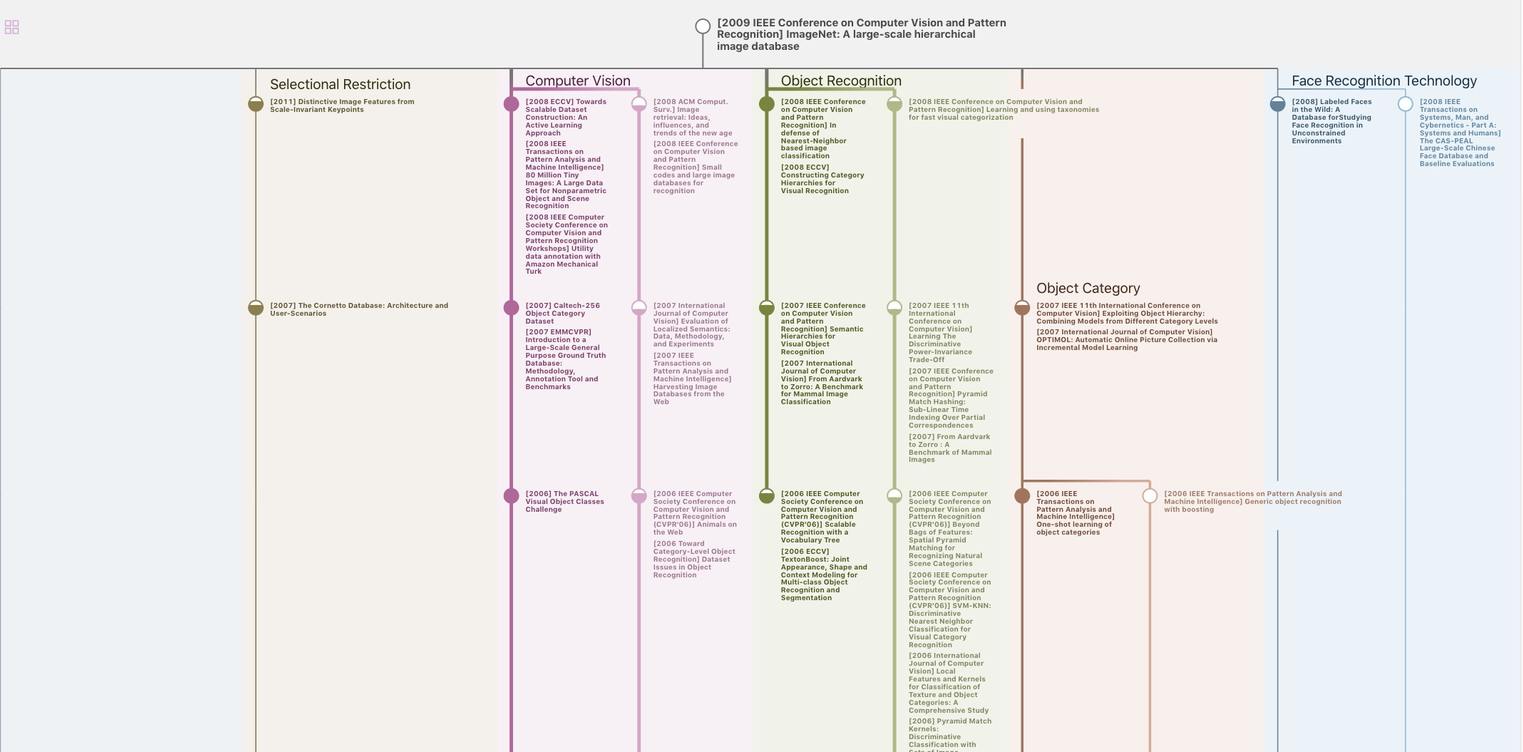
生成溯源树,研究论文发展脉络
Chat Paper
正在生成论文摘要