Estimating the prevalence of chronic kidney disease while accounting for nonrandom testing with inverse probability weighting
Kidney international(2023)
摘要
Chronic kidney disease (CKD) prevalence estimation is central to guiding the medical community and policymakers on where to focus prevention and disease management strategies. Population sources make the estimation of CKD prevalence challenging. In screening cohort studies, healthy individuals are more likely to participate than nonhealthy individuals, which may lead to the underestimation of CKD prevalence. In routine care cohorts, persons undergoing testing for creatinine to estimate glomerular filtration rate (eGFR) and albuminuria may be sicker and with indication for the testing (i.e., with diabetes or hypertension), which may lead to the overestimation of CKD prevalence. Both study designs are thus likely to suffer from volunteer or referral bias, which is ultimately a source of selection bias.1Smith L.H. Selection mechanisms and their consequences: understanding and addressing selection bias.Curr Epidemiol Rep. 2020; 7: 179-189Crossref Google Scholar These types of biases can be overcome if we know the characteristics of the population not included in the study sample, and how these differ from the characteristics of those who are included. However, to the best of our knowledge, this has not been possible to date. Using health data from the complete population of Stockholm during 2015–2018,2Carrero J.J. Elinder C.G. The Stockholm CREAtinine Measurements (SCREAM) project: fostering improvements in chronic kidney disease care.J Intern Med. 2022; 291: 254-268Crossref PubMed Scopus (20) Google Scholar we conducted an observational study to estimate the prevalence of CKD accounting for nonrandom testing for eGFR and albuminuria. At each calendar year, we identified any citizen tested in health care for creatinine, albuminuria, or both and evaluated differences in characteristics from those untested. We used this information to estimate the propensity to receive a kidney function test and used inverse probability weighting to account for this differential testing. Inverse probability weighting assigns higher weights to observations from under-represented groups to make them representative of the whole target population. Thus, weighting by the inverse probability of being tested eliminates bias that arises from selection by the indication of the test, to the extent that the indication of the test is measured and controlled for in the weights. We used this weighted population to calculate the prevalence of Kidney Disease: Improving Global Outcomes (KDIGO) G and A categories as well as CKD stages 1–5,3Kidney Disease: Improving Global Outcomes (KDIGO) Blood Pressure Work GroupKDIGO 2021 clinical practice guideline for the management of blood pressure in chronic kidney disease.Kidney Int. 2021; 99: S1-S87PubMed Scopus (365) Google Scholar exploring weighted CKD prevalence(s) across subgroups, including populations with repeated testing where referral biases were highest. We also explored prevalence estimates in earlier periods. Detailed description of our methodology is provided in Supplementary Tables S1 and S2 and Supplementary Appendix S1. During 2015–2018, there were 1,876,339 adult citizens in Stockholm, of whom 57% and 26% were tested for creatinine-based eGFR or albuminuria, respectively, at least once (Table 1). The mean age was 45.9 (SD 18.9) years, 52% were men, and the mean eGFR was 90.9 (SD 21.1) ml/min per 1.73 m2. The median (interquartile range) number of creatinine and albuminuria measurements per individual was 2 (1, 3) (range: 1–4) and 1 (1, 2) (range: 1–4), respectively. Tested individuals were older, more often women, and had more comorbid conditions (e.g., hypertension or diabetes) than nontested individuals. Annual testing rates and characteristics of citizens during 2011–2014 and 2007–2010 are similar and shown in Supplementary Table S3, Supplementary Appendix S2, and Supplementary Tables S4–S7.Table 1Characteristics of the population during 2015–2018, by whether they were tested for eGFR and/or albuminuriaCharacteristicTested for creatinineTested for albuminuriaTested for creatinine and albuminuriaaDefined by the presence of at least 1 measurement of creatinine and albuminuria in the same calendar year.Never tested for creatinine and/or albuminuria, N = 780,256Overall, N = 1,876,339No, N = 813,020 (43%)Yes, N = 1,063,319 (57%)No, N = 1,397,903 (74%)Yes, N = 478,436 (26%)No, N = 1,453,236 (77.5%)Yes, N = 423,103 (22.5%)Number of testsN/A2,327,195N/A815,451N/A732,091N/A2,410,555Tests per patient, median (IQR) [range]N/A2 (1, 3) [1–4]N/A1 (1, 2) [1–4]N/A1 (1, 2) [1–4]N/A2 (1, 3) [1–4]Age, yr, mean (SD)37.1 (15.0)52.7 (18.8)42.7 (18.0)54.7 (19.0)42.5 (17.9)56.9 (18.4)37.2 (15.1)45.9 (18.9)Sex, n (%)Men424,635 (52)485,034 (46)700,859 (50)208,810 (44)715,242 (49)194,427 (46)415,901 (53)909,669 (48)Women388,385 (48)578,285 (54)697,044 (50)269,626 (56)737,994 (51)228,676 (54)364,355 (47)966,670 (52)eGFR, ml/min per 1.73 m2, (SD)N/A90.9 (21.1)90.0 (21.6)86.8 (22.6)90.3 (21.7)87.1 (22.6)N/A90.9 (21.1)KDIGO G categories, n (%)G1N/A580,442 (55)159,346 (52)196,120 (47)165,260 (53)202,390 (48)N/A580,442 (55)G2N/A404,899 (38)119,201 (39)169,478 (41)121,161 (39)172,723 (41)N/A404,899 (38)G3aN/A50,995 (4.8)16,388 (5.4)29,400 (7.1)16,529 (5.3)29,736 (7.0)N/A50,995 (4.8)G3bN/A18,672 (1.8)6233 (2.1)11,895 (2.9)6267 (2.0)11,982 (2.8)N/A18,672 (1.8)G4N/A5365 (0.5)1678 (0.6)3879 (0.9)1683 (0.5)3895 (0.9)N/A5365 (0.5)G5N/A2946 (0.3)688 (0.2)2376 (0.6)688 (0.2)2377 (0.6)780,2562946 (0.3)MissingN/AN/A1,094,36965,2881,141,6480N/AN/AKDIGO A categories, n (%)A110,123 (99.7)252,822 (91)N/A443,402 (93)15,972 (99.4)388,045 (92)N/A262,945 (92)A220 (0.2)18,741 (6.8)N/A28,404 (5.9%)53 (0.3)28,413 (6.7)N/A18,761 (6.5)A36 (<0.1)5087 (1.8)N/A6630 (1.4)9 (<0.1)6645 (1.6)N/A5093 (1.8)Missing802,871786,6691,397,90301,437,2020780,2561,589,540Comorbid conditions, n (%)Hypertension29,259 (3.6)316,554 (30)148,885 (11)197,630 (41)149,398 (10)198,106 (47)28,529 (3.7)345,813 (18)Diabetes mellitus7480 (0.9)106,565 (10)23,883 (1.7)94,370 (20)24,453 (1.7)94,077 (22)7081 (0.9)114,045 (6.1)Myocardial infarction1847 (0.2)29,187 (2.7)13,351 (1.0)17,236 (3.6)13,329 (0.9)17,559 (4.2)1814 (0.2)31,034 (1.7)Peripheral arterial disease1918 (0.2)25,484 (2.4)12,758 (0.9)14,905 (3.1)12,631 (0.9)15,261 (3.6)1871 (0.2)27,402 (1.5)Cerebrovascular disease4301 (0.5)46,551 (4.4)25,647 (1.8)25,191 (5.3)25,629 (1.8)25,468 (6.0)4199 (0.5)50,852 (2.7)Heart failure2661 (0.3)43,301 (4.1)22,145 (1.6)24,021 (5.0)21,926 (1.5)25,109 (5.9)2616 (0.3)45,962 (2.4)Medications, n (%)RASi17,460 (2.1)252,798 (24)103,510 (7.4)164,017 (34)105,318 (7.2)162,608 (38)17,042 (2.2)270,258 (14)Diuretic6307 (0.8)100,541 (9.5)45,146 (3.2)60,620 (13)46,147 (3.2)60,314 (14)6149 (0.8)106,848 (5.7)β-Blockers15,491 (1.9)181,229 (17)86,053 (6.2)105,355 (22)88,364 (6.1)104,199 (25)14,984 (1.9)196,720 (10)Lipid-lowering therapy9926 (1.2)160,629 (15)63,290 (4.5)106,327 (22)64,438 (4.4)105,542 (25)9686 (1.2)170,555 (9.1)eGFR, estimated glomerular filtration rate; IQR, interquartile range; KDIGO, Kidney Disease: Improving Global Outcomes; N/A, not available; RASi, renin-angiotensin system inhibitor.a Defined by the presence of at least 1 measurement of creatinine and albuminuria in the same calendar year. Open table in a new tab eGFR, estimated glomerular filtration rate; IQR, interquartile range; KDIGO, Kidney Disease: Improving Global Outcomes; N/A, not available; RASi, renin-angiotensin system inhibitor. Our a priori identified predictors included all covariates described in Supplementary Table S1 and showed, in general, good ability to predict the likelihood of being tested for albuminuria/creatinine, with the area under the curve of 0.77 or higher (Supplementary Figure S1). Weighting for the inverse probability of testing brought balance to the distribution of covariates with standardized mean differences at or below 0.1 (Supplementary Figure S2). The unweighted period prevalence of CKD stages 1–5 was 18.6% (18.0%–18.8%) (Supplementary Table S8). After weighting, it decreased to 10.6% (10.0%–10.8%). The weighted prevalence of CKD stages 3–5 was 5.4% (95% confidence interval: 5.3%−5.5%), and the weighted prevalence of albuminuria A2 and A3 was 5.4% (5.4%–5.5%) and 1.1% (1.0%–1.1%), respectively. Supplementary Table S9 shows similar prevalence estimates for earlier study periods. The prevalence of CKD increased markedly with older age and was higher among men (Figure 1a and Supplementary Tables S10 and S11), among individuals with diabetes mellitus, hypertension, or cardiovascular disease (Figure 1b). Supplementary Figures S3 and S4 show similar proportions for the earlier study periods. In Supplementary Table S12, we provide the examples of subpopulations over- or under-represented if selected by the presence of albuminuria or creatinine, and the effect of weighting in their CKD prevalence estimates (Supplementary Appendix S2). We identified 538,543 participants who had ≥2 creatinine measurements at least 3 months apart, and we calculated their sustained eGFR over time. Of those, 245,383 participants had, in addition, ≥2 albuminuria measurements that allowed us to evaluate their sustained albuminuria over time. Overall, there were important differences in the characteristics of participants with/without repeated laboratory testing (Supplementary Table S13). The unweighted prevalence of this selected population was 23.1% (22.5%–23.5%), which decreased to 11.4% (11.1%–11.7%) after weighting (Supplementary Table S14). By evaluating the complete population of Stockholm, Sweden, we show that creatinine and albuminuria testing are directed to high-risk populations, and that evaluating CKD prevalence based on this directed testing in routinely collected health care data provides exaggerated estimates of the population CKD burden. We also show that by weighting against the remaining untested population, CKD stage 1–5 prevalence figures decrease to proportions that agree with current global meta-analyses.4Mills K.T. Xu Y. Zhang W. et al.A systematic analysis of worldwide population-based data on the global burden of chronic kidney disease in 2010.Kidney Int. 2015; 88: 950-957Abstract Full Text Full Text PDF PubMed Scopus (532) Google Scholar Studies assessing the prevalence of CKD have provided disparate estimates,5Brück K. Stel V.S. Gambaro G. et al.CKD prevalence varies across the European general population.J Am Soc Nephrol. 2016; 27: 2135-2147Crossref PubMed Scopus (347) Google Scholar in part attributed to the (lack of) representativeness of screening cohorts and methods for ascertainment of eGFR and albuminuria.6Brück K. Jager K.J. Dounousi E. et al.Methodology used in studies reporting chronic kidney disease prevalence: a systematic literature review.Nephrol Dial Transplant. 2015; 30: iv6-iv16Crossref PubMed Scopus (63) Google Scholar Routine health care data may be used to evaluate CKD prevalence as they have the advantage of large sample sizes, but are affected by the health system coverage uptake, access to care, and the reasons that prompted the testing for these kidney measures. We show that propensity score weighting, accounting for health status of the untested population, provided stable estimates of CKD prevalence that did not differ substantially across periods, special subgroups, or populations with repeated testing, in which selection biases were highest. Using this method, we observe that between 10% and 11% of adults in our region suffer from CKD, and that this prevalence proportion has been rather stable during the 12 years evaluated. Generalization of our methods to other large health systems may enhance CKD surveillance efforts by using weights to derive population prevalence estimates from select patient samples. Our approach can also be extended to other initiatives: for example, the CKD surveillance system7Centers for Disease Control and PreventionChronic Kidney Disease (CKD) Surveillance Project—United States.https://nccd.cdc.gov/ckd/data.aspxGoogle Scholar in the US population is based on a limited number of National Health and Nutrition Examination Survey participants with an eGFR <60 (n = 2700) and eGFR <30 (n = 239) ml/min. As study strengths, we emphasize the complete population capture of approximately 3 million individuals with millions of kidney function tests and health conditions among those not tested. As study limitations, we acknowledge that unmeasured explanatory factors are present in both tested and untested population. More complex methods, like random forests or entropy balancing, may have helped us increasing the performance of our models, but we purportedly chose covariates easily available in databases to allow reproducibility by others. We acknowledge the lack of information on body mass index, diabetes duration, or blood pressure, which are also the risk factors of CKD and influence the probability of being tested. We conclude that when using routine care data sources, weighting to account for untested individuals may provide more reliable prevalence estimates than naïve evaluation of clinically tested patients. JJC acknowledges consultancy for AstraZeneca and grants support to Karolinska Institutet from AstraZeneca, Vifor Pharma, and Astellas (all outside the submitted work). All other authors declared no competing interests. Research reported in this publication was supported by the Swedish Research Council (#2019-01059) and an unrestricted grant from AstraZeneca Sweden. Download .docx (2.6 MB) Help with docx files Supplementary File (Word)
更多查看译文
关键词
chronic kidney injury,epidemiology and statistics,renal function testing
AI 理解论文
溯源树
样例
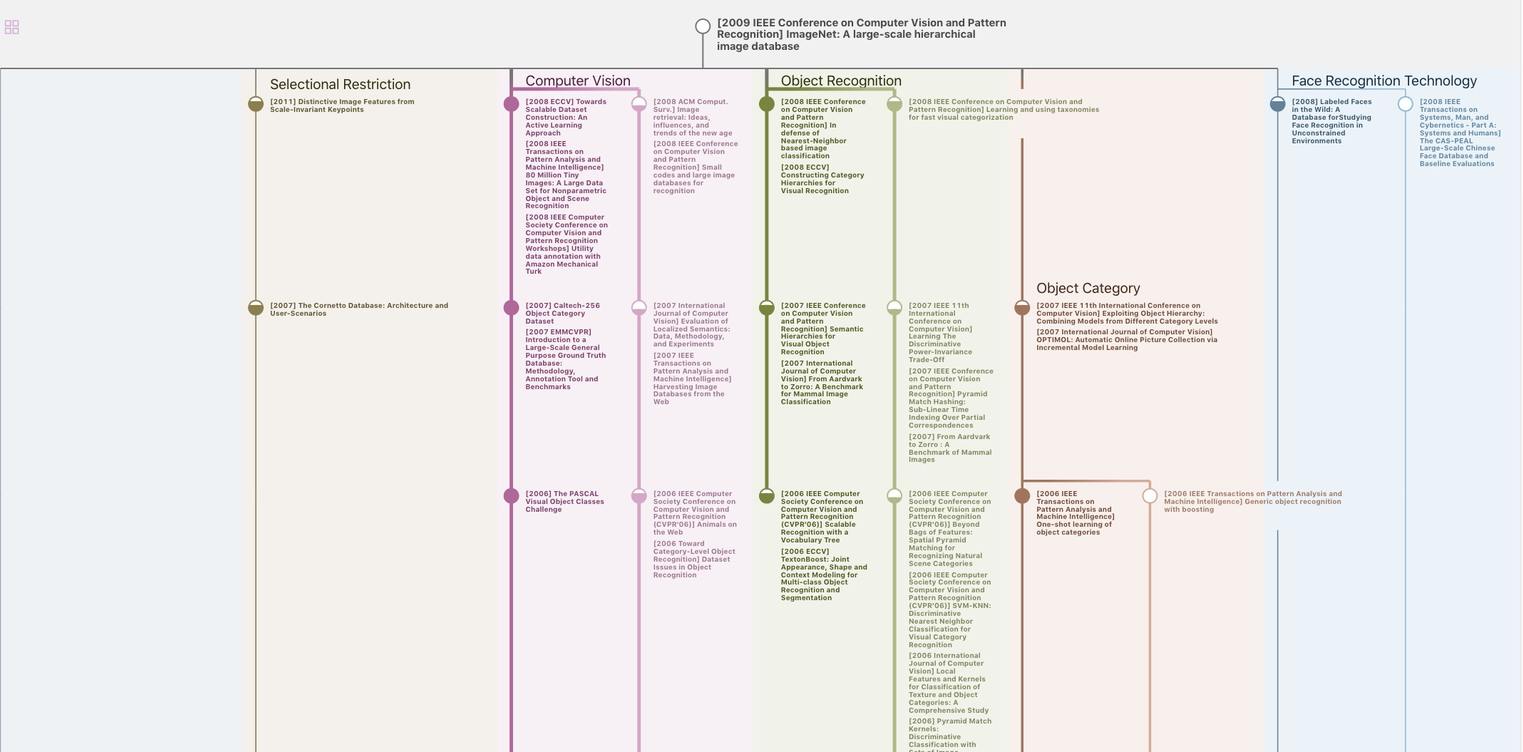
生成溯源树,研究论文发展脉络
Chat Paper
正在生成论文摘要