Deep Learning Discrete Calculus (DLDC): a family of discrete numerical methods by universal approximation for STEM education to frontier research
arxiv(2023)
摘要
The article proposes formulating and codifying a set of applied numerical methods, coined as D eep L earning D iscrete C alculus (DLDC), that uses the knowledge from discrete numerical methods to interpret the deep learning algorithms through the lens of applied mathematics. The DLDC methods aim to leverage the flexibility and ever-increasing resources of deep learning and rich literature on numerical analysis to formulate a general class of numerical methods that can directly use data with uncertainty to predict the behavior of an unknown system as well as elevate the speed and accuracy of numerical solution of the governing equations for known systems. The article is structured into two major sections. In the first section, the building blocks of the DLDC methods are presented and deep learning structures analogous to traditional numerical methods such as finite difference and finite element methods are constructed with a view to incorporate these techniques in Science, Technology, Engineering, Mathematics syllabus for K-12 students. The second section builds upon the building blocks of the previous discussion and proposes new solution schemes for differential and integral equations pertinent to multiscale mechanics. Each section is accompanied by a mathematical formulation of the numerical methods, analogous DLDC formulation, and suitable examples.
更多查看译文
关键词
Numerical methods,Discrete calculus,Kernel learning,Partial differential equation,Convolution
AI 理解论文
溯源树
样例
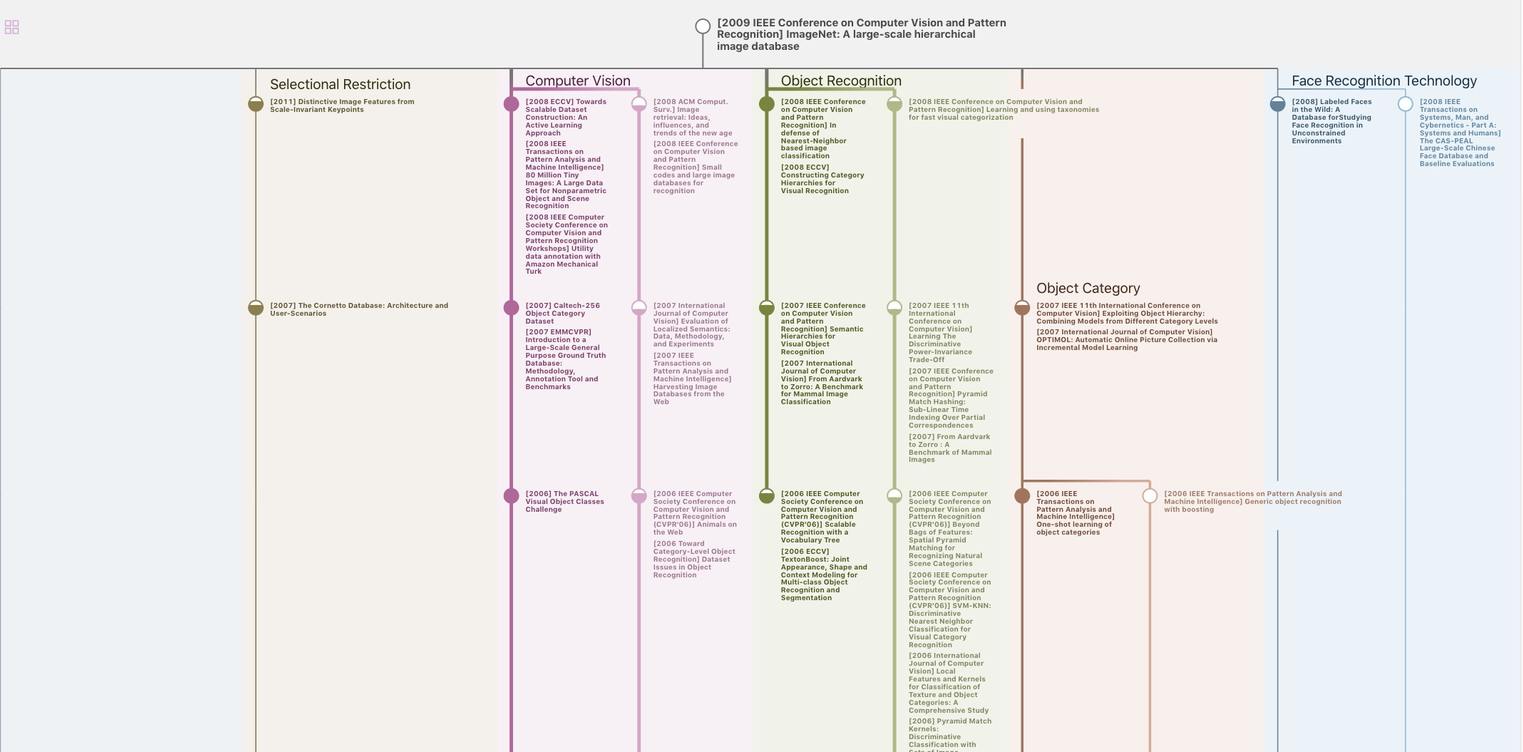
生成溯源树,研究论文发展脉络
Chat Paper
正在生成论文摘要