GCN2CAPS: Graph Convolutional Network to Capsule Network For Wide-Field Robust Graph Learning.
ICPR(2022)
摘要
Graph Neural Networks (GNNs) have achieved remarkable performance in extracting structure-aware node representations for graph signal data. However, existing GNNs overly emphasize the consistency of neighbor nodes in the limited receptive field and severely overlook the wide-field information. In this paper, we propose a novel GCN2Caps that transfers multi-field node representations from graph convolutional network (GCN) to capsule network for wide-field graph learning. Specifically, multi-field GCN is employed to extend the receptive field and extract multi-field features. To perform multi-field interactions, GCN2Caps then explores the inherent relationships between multi-field features and generates the wide-field features through the capsule mechanism. On the basis of the wide-field features, a wide-field min-cut graph constraint is introduced to the loss function to execute complementary constraints on the original graph structure. Extensive experiments on three real-world datasets demonstrate that GCN2Caps significantly outperforms state-of-the-art baselines on semi-supervised node classification and the robustness of GCN2Caps is further validated.
更多查看译文
关键词
Graph Representations,Multi-field Graph Learning,Graph Convolutional Network,Capsule Mechanism,Min-cut Constraint
AI 理解论文
溯源树
样例
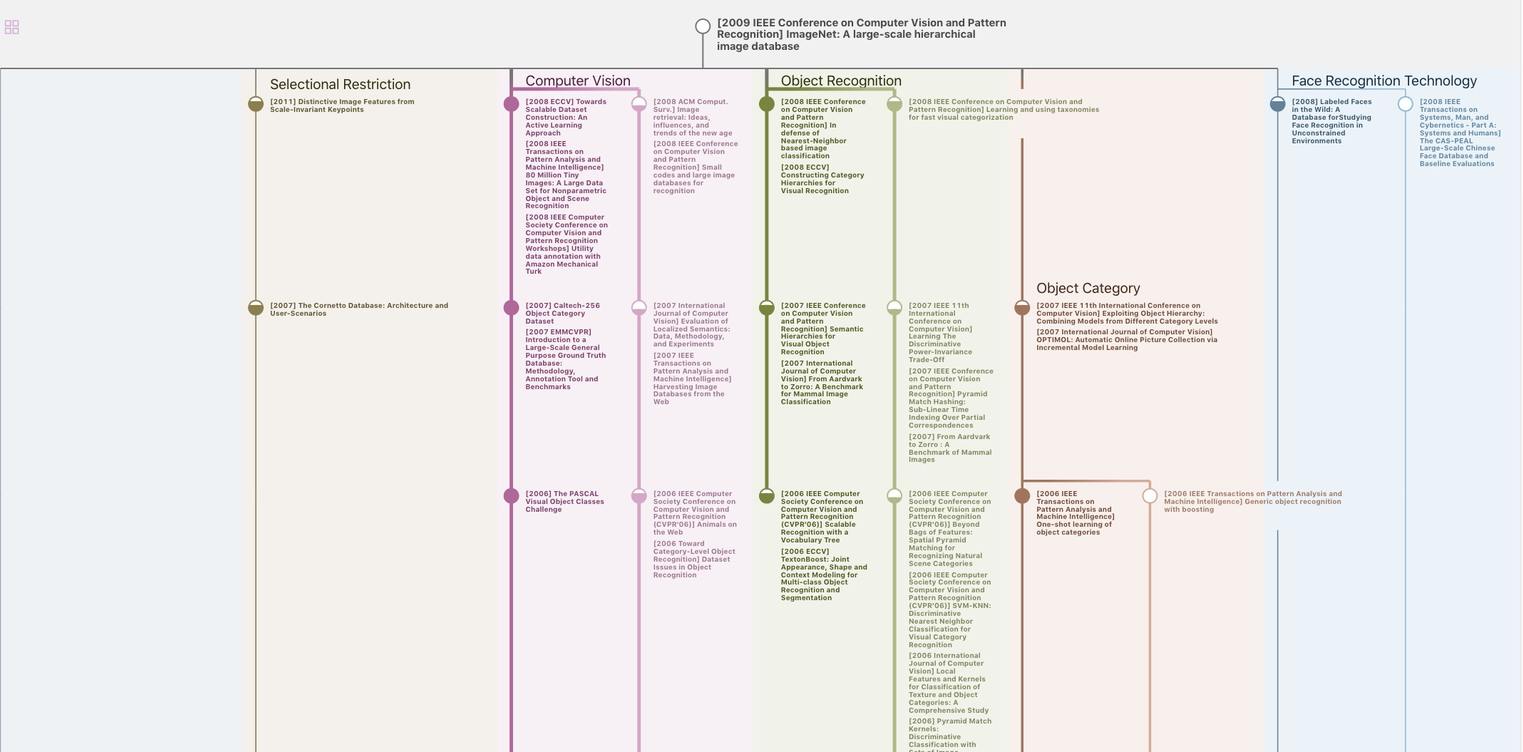
生成溯源树,研究论文发展脉络
Chat Paper
正在生成论文摘要