Faster Algorithm for Structured John Ellipsoid Computation
arxiv(2022)
摘要
Computing John Ellipsoid is a fundamental problem in machine learning and convex optimization, where the goal is to compute the ellipsoid with maximal volume that lies in a given convex centrally symmetric polytope defined by a matrix $A \in \mathbb{R}^{n \times d}$. In this work, we show two faster algorithms for approximating the John Ellipsoid. $\bullet$ For sparse matrix $A$, we can achieve nearly input sparsity time $\mathrm{nnz}(A) + d^{\omega}$, where $\omega$ is exponent of matrix multiplication. Currently, $\omega \approx 2.373$. $\bullet$ For the matrix $A$ which has small treewidth $\tau$, we can achieve $n \tau^2$ time. Therefore, we significantly improves the state-of-the-art results on approximating the John Ellipsoid for centrally symmetric polytope [Cohen, Cousins, Lee, and Yang COLT 2019] which takes $nd^2$ time.
更多查看译文
AI 理解论文
溯源树
样例
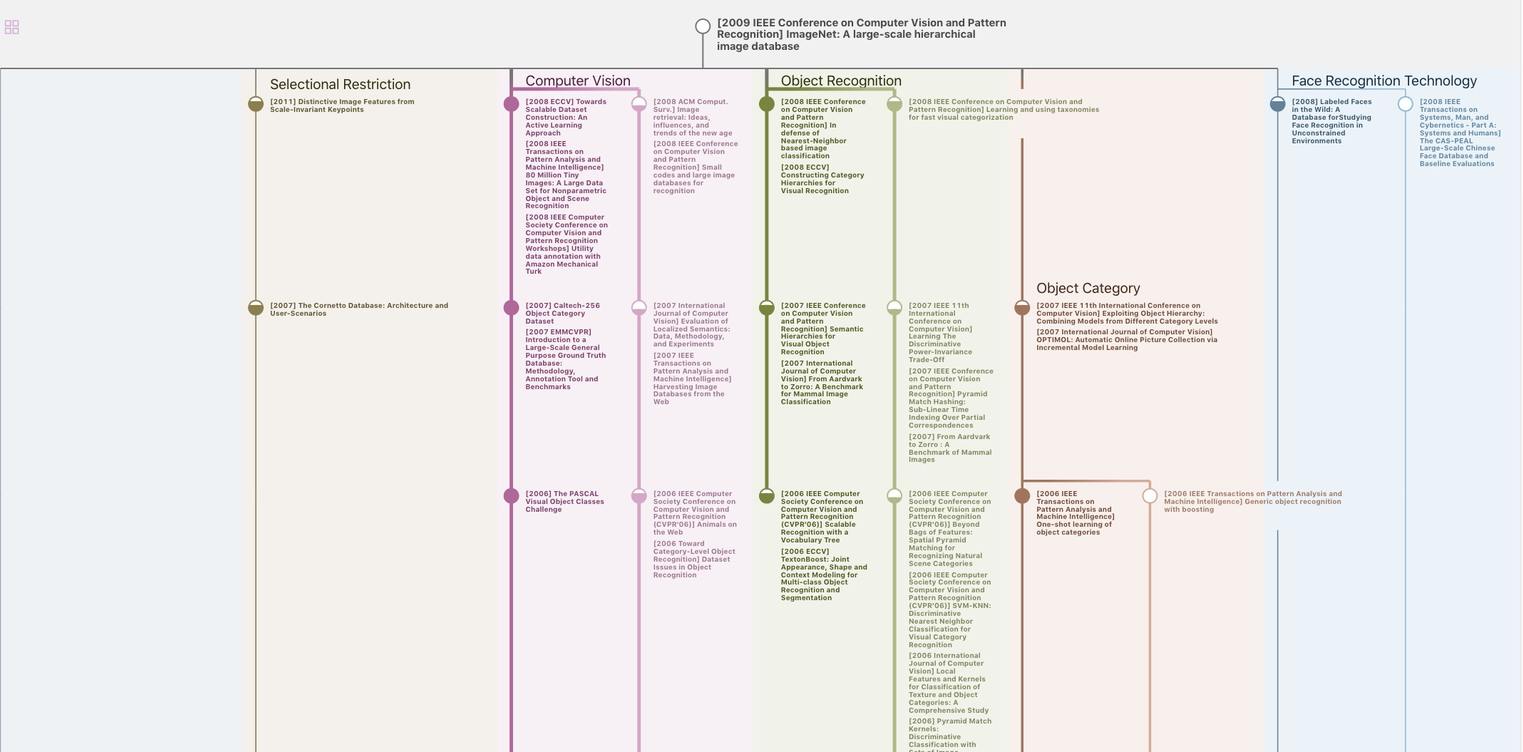
生成溯源树,研究论文发展脉络
Chat Paper
正在生成论文摘要