Diffusion-SDF: Conditional Generative Modeling of Signed Distance Functions
arxiv(2023)
摘要
Probabilistic diffusion models have achieved state-of-the-art results for image synthesis, inpainting, and text-to-image tasks. However, they are still in the early stages of generating complex 3D shapes. This work proposes Diffusion-SDF, a generative model for shape completion, single-view reconstruction, and reconstruction of real-scanned point clouds. We use neural signed distance functions (SDFs) as our 3D representation to parameterize the geometry of various signals (e.g., point clouds, 2D images) through neural networks. Neural SDFs are implicit functions and diffusing them amounts to learning the reversal of their neural network weights, which we solve using a custom modulation module. Extensive experiments show that our method is capable of both realistic unconditional generation and conditional generation from partial inputs. This work expands the domain of diffusion models from learning 2D, explicit representations, to 3D, implicit representations.
更多查看译文
关键词
conditional generative modeling,signed distance
AI 理解论文
溯源树
样例
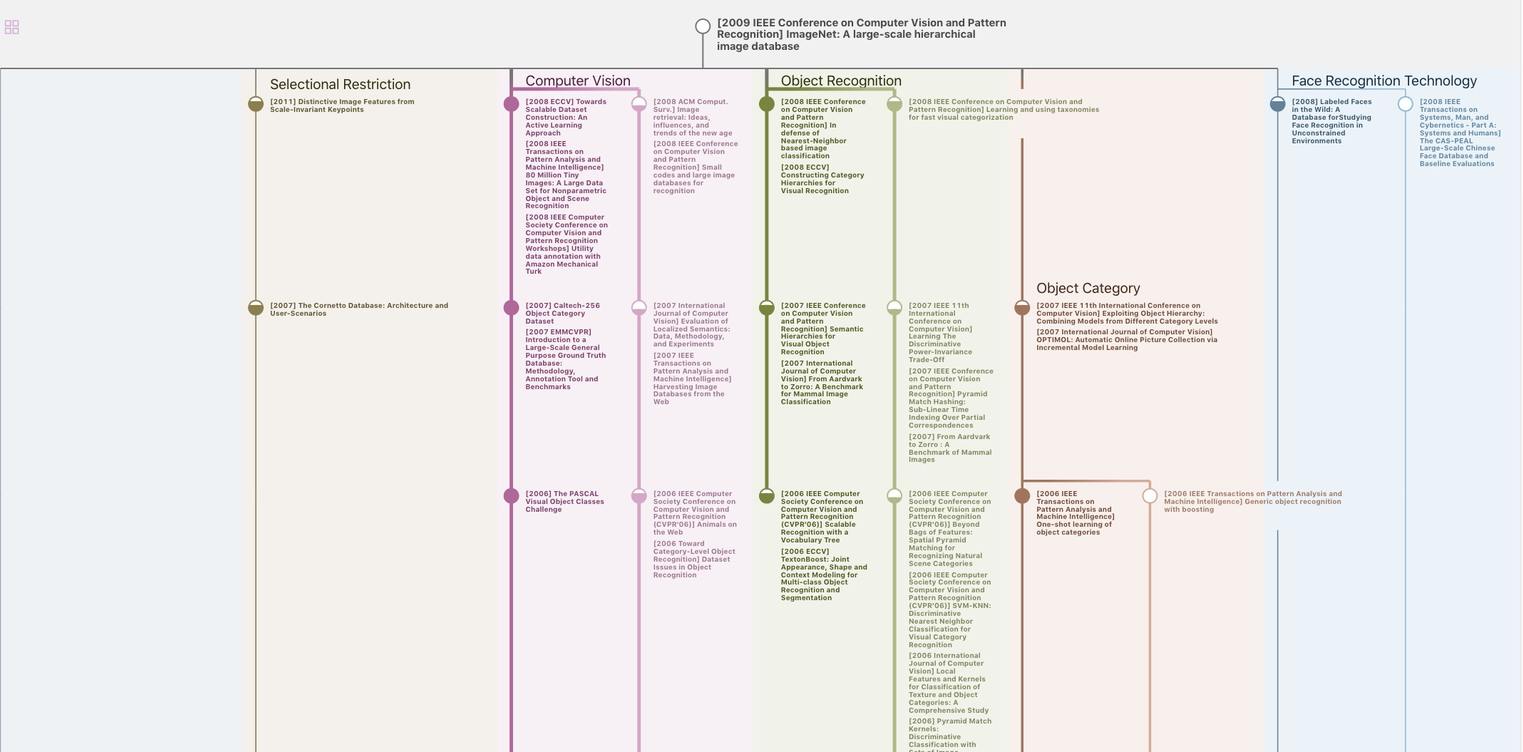
生成溯源树,研究论文发展脉络
Chat Paper
正在生成论文摘要