Improving Neural Network Time Series Prediction with a GA-BFGS Grown Dynamic Architecture
2022 27th International Conference on Automation and Computing (ICAC)(2022)
摘要
A dynamic recurrent neural network is seen to be the most efficient nonlinear approach to time series prediction, especially if its architecture is grown to construct the prediction model. To further advance its performance, this paper develops a hybrid evolutionary algorithm to grow and train the network and its dynamic architecture holistically. The training approach is based on the genetic algorithm (GA) and the fastest quasi-Newton algorithm Broyden-Fletcher-Goldfarb-Shanno (BFGS). The proposed GA-BFGS method is suitable for training both discrete structural parameters and continuous weighting parameters of the network and is applicable to parallel computation. This approach eliminates the need for setting any hyperparameters of the neural network for the highest possible optimality and accuracy to emerge. The method is tested on the Mackey-Glass time series and its forecasting performance is compared with currently widely adopted deep learning methods such as the long short-term memory (LSTM) and echo state networks (ESN) models. Results show that the proposed method incurs lower losses, requires a smaller network size, and performs faster.
更多查看译文
关键词
dynamic recurrent neural network,hybrid evolutionary algorithm,parallel computation,time series prediction
AI 理解论文
溯源树
样例
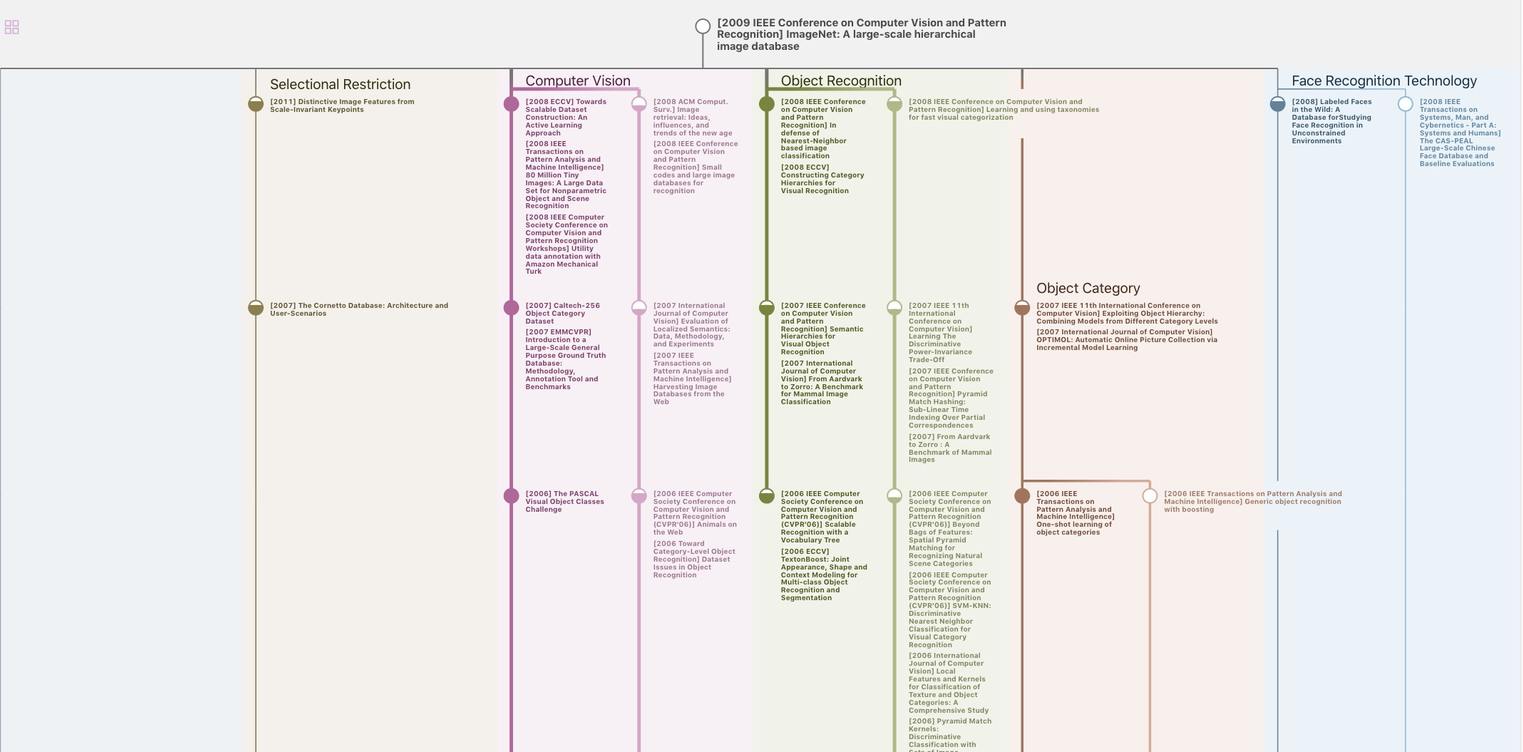
生成溯源树,研究论文发展脉络
Chat Paper
正在生成论文摘要