Transformer-based Video Summarization With Spatial-Temporal Representation
2022 8th International Conference on Big Data and Information Analytics (BigDIA)(2022)
摘要
Video summarization is an important topic studied by researchers. With the application of deep learning, CNN and RNN have also been used to generate video summarization. However, because a video contains many frames and the video timing span is large, the spatial-temporal architecture of the video is complex, but it is necessary to abstract the spatial-temporal structure information to generate a summarization, and it is also the focus of the researchers recently. Based on previous researchers' research, we put forward a new way for video summary generation, which consists of three deep neural network models. First, a 2D convolutional CNN is used to process video frames, convert a short video into a vector form that can be flexibly calculated, and then use 1D convolution to perform sequence analysis on the timing information of data, and then use the Transformer encorder model that is currently used in natural language processing to further extract timing information, and finally use up-sampling to obtain output to make its dimension the same as video frames number in input short video. Through training learning allows the model to get importance scores which indicate the importance of video frames, and then select key shots to obtain video summaries. Experimental data illustrates that our model get better performance than existing methods on two generic datasets.
更多查看译文
关键词
Video Summarization,Convolutional Neural Network,Transformer,Deep Learning
AI 理解论文
溯源树
样例
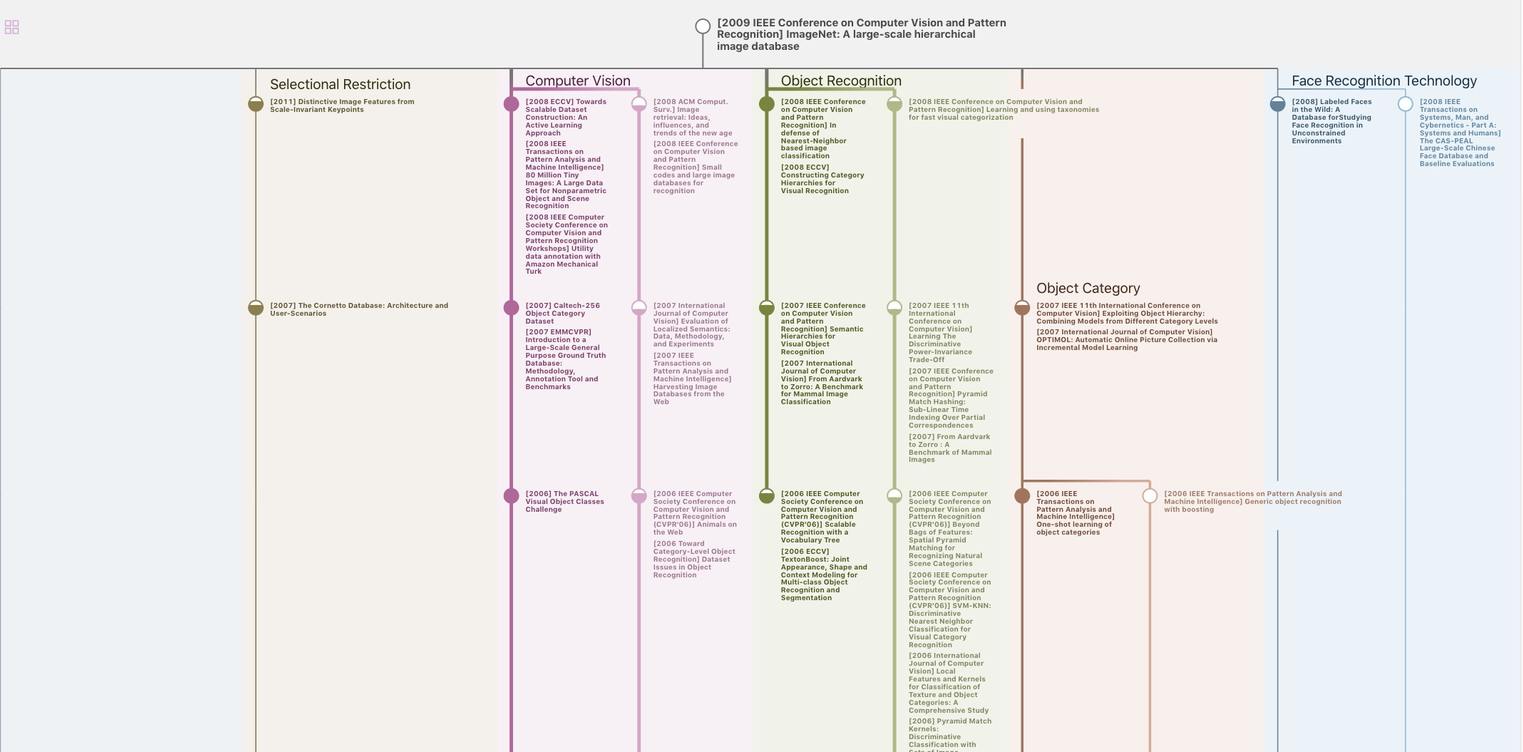
生成溯源树,研究论文发展脉络
Chat Paper
正在生成论文摘要