A Novel Machine Learning-Based Point-Score Model as a Non-Invasive Decision-Making Tool for Identifying Infected Ascites in Patients with Hydropic Decompensated Liver Cirrhosis: A Retrospective Multicentre Study.
Antibiotics (Basel, Switzerland)(2022)
摘要
This study is aimed at assessing the distinctive features of patients with infected ascites and liver cirrhosis and developing a scoring system to allow for the accurate identification of patients not requiring abdominocentesis to rule out infected ascites. A total of 700 episodes of patients with decompensated liver cirrhosis undergoing abdominocentesis between 2006 and 2020 were included. Overall, 34 clinical, drug, and laboratory features were evaluated using machine learning to identify key differentiation criteria and integrate them into a point-score model. In total, 11 discriminatory features were selected using a Lasso regression model to establish a point-score model. Considering pre-test probabilities for infected ascites of 10%, 15%, and 25%, the negative and positive predictive values of the point-score model for infected ascites were 98.1%, 97.0%, 94.6% and 14.9%, 21.8%, and 34.5%, respectively. Besides the main model, a simplified model was generated, containing only features that are fast to collect, which revealed similar predictive values. Our point-score model appears to be a promising non-invasive approach to rule out infected ascites in clinical routine with high negative predictive values in patients with hydropic decompensated liver cirrhosis, but further external validation in a prospective study is needed.
更多查看译文
关键词
ascites,liver cirrhosis,proton pump inhibitor,secondary peritonitis,spontaneous bacterial peritonitis
AI 理解论文
溯源树
样例
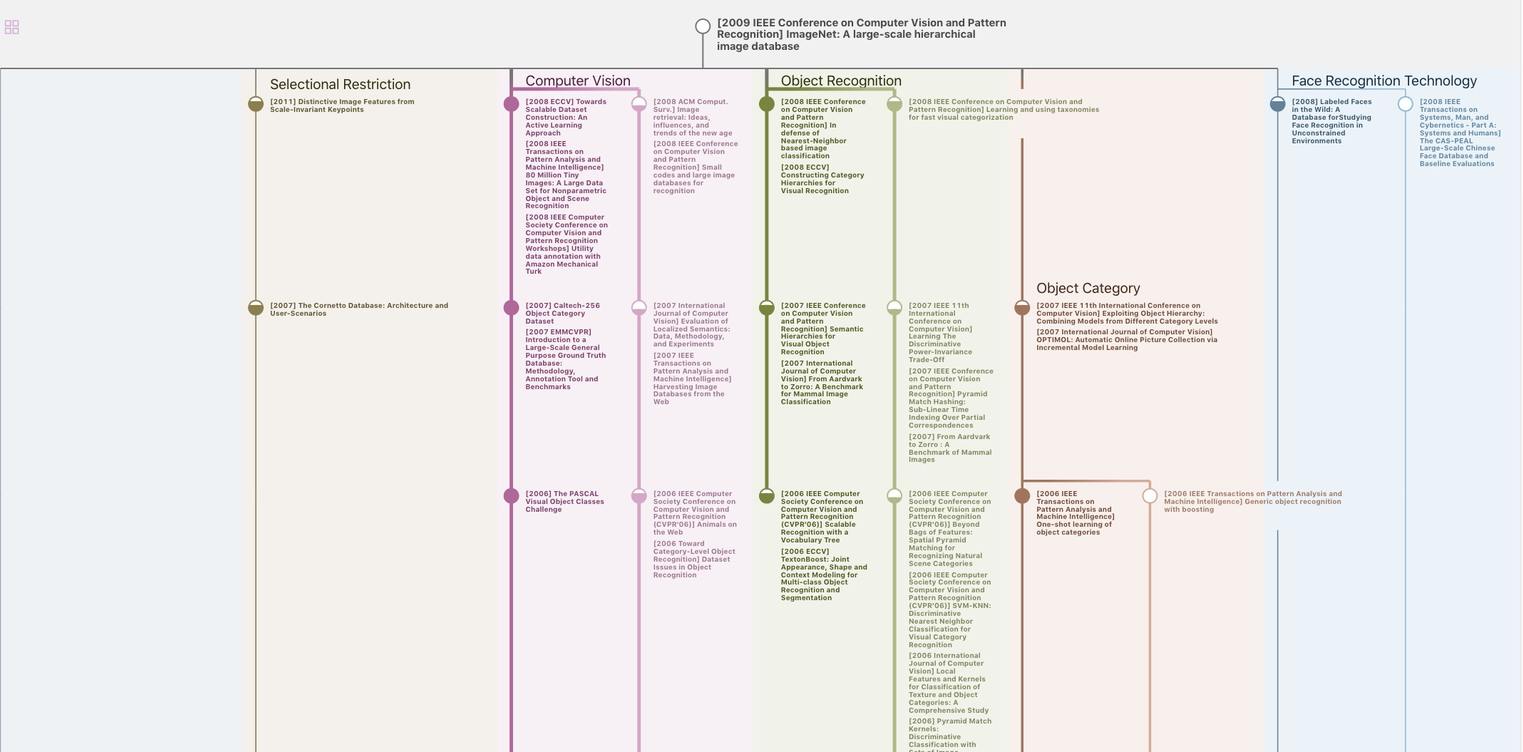
生成溯源树,研究论文发展脉络
Chat Paper
正在生成论文摘要