Reinforcement Learning for Curbside Space Management with Infrastructure Autonomy and Mixed Vehicle Connectivity.
International Conference on Intelligent Transportation Systems (ITSC)(2022)
摘要
Urban curbside parking has been a headache for a wide range of urban stakeholders. It is difficult to solve and is rarely regarded independent from the well-studied parking management problem. However, a closer look at its properties and a comparison points out the unique features of curbside parking game that involves both the parking/cruising traffic and the roadway traffic. Two gaps in literature and prototypes that shape the future of the curbside are identified. And to bridge them, this paper proposes to innovatively solve it by infrastructure autonomy, modeling the curbs as agents. Later, this study considers heterogeneity of vehicles in two dimensions and connects them to reduce problem complexity. A model for curbside space management (CSM) is developed and solved via a reinforcement learning (RL) scheme. Partial observations and full information are fed to different components in the model respectively for robust training. Results based on simulation show the proposed model outperform two baseline control strategies and learns robustly.
更多查看译文
关键词
curbside space management,infrastructure autonomy,reinforcement
AI 理解论文
溯源树
样例
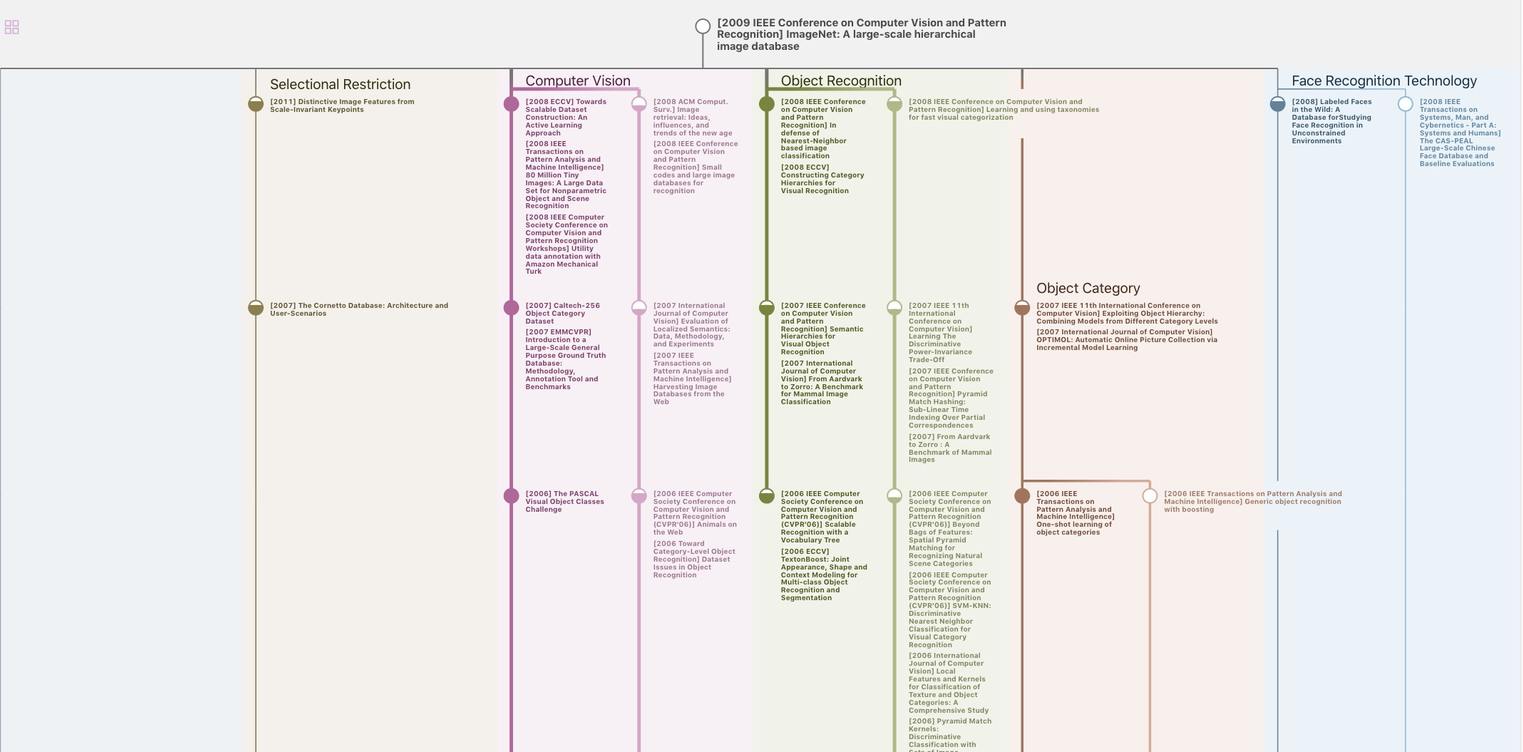
生成溯源树,研究论文发展脉络
Chat Paper
正在生成论文摘要